Applying Probabilistic Thematic Clustering for Classification in the TREC 2005 Genomics Track
TREC(2005)
摘要
Our group participated in the categorization task of the TREC Genomics Track. We introduced and investigated a cluster-based approach for classifying documents. We first clustered the abstracts of the negative training examples based on their term distribution, then built a classifier to distinguish between each cluster and the set of positive examples. The large number of resulting classifiers (a total of 14-19 classifiers per domain) was combined to categorize the test set. We also conducted experiments for cluster- based feature selection; Rather than select features from the whole negative and positive training sets, we selected features from each of the clusters and took the union of these features as the selected features for representing the whole training and test data. We compared our cluster-based multi-classifier approach against a simple naïve Bayes classification. We also compared the cluster-based feature selection strategy with the commonly used Chi-square-based feature selection. 1. Introduction Text categorization was one of the two tasks in the TREC 2005 Genomics Track. It was concerned with the classification of articles from four major categories, including alleles of mutant phenotypes, embryologic gene expression, tumor biology, and gene ontology (GO) annotation. The task was to identify documents that are relevant to these categories, using a classifier trained on the labeled data. The full text articles for both training and test set were given, although we used only title, abstract and MeSH terms in our experiments. All articles in the training set were published in 2002, while articles in the test set were published in 2003. Therefore, both the training and test examples are not selected uniformly at random. The text categorization task provides the crosswalk files for both the training and test data as well. The corresponding PubMed ID (PMID) of each article is given in these files.
更多查看译文
关键词
gene expression,feature selection
AI 理解论文
溯源树
样例
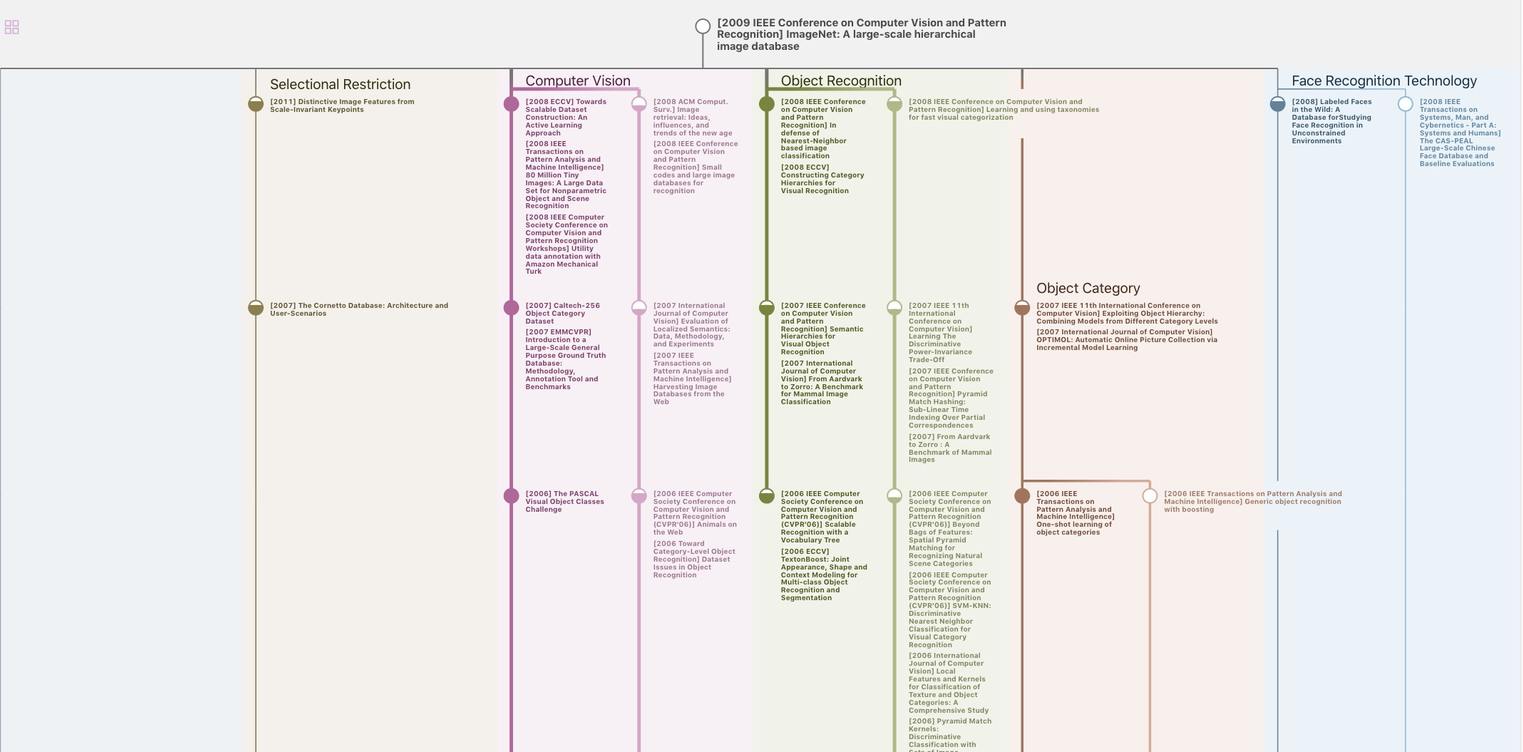
生成溯源树,研究论文发展脉络
Chat Paper
正在生成论文摘要