Exploring Patient Risk Groups with Incomplete Knowledge
ICDM(2013)
摘要
Patient risk stratification, which aims to stratify a patient cohort into a set of homogeneous groups according to some risk evaluation criteria, is an important task in modern medical informatics. Good risk stratification is the key to good personalized care plan design and delivery. The typical procedure for risk stratification is to first identify a set of risk-relevant medical features (also called risk factors), and then construct a predictive model to estimate the risk scores for individual patients. However, due to the heterogeneity of patients' clinical conditions, the risk factors and their importance vary across different patient groups. Therefore a better approach is to first segment the patient cohort into a set of homogeneous groups with consistent clinical conditions, namely risk groups, and then develop group-specific risk prediction models. In this paper, we propose RISGAL (RISk Group Analysis), a novel semi-supervised learning framework for patient risk group exploration. Our method segments a patient similarity graph into a set of risk groups such that some risk groups are in alignment with (incomplete) prior knowledge from the domain experts while the remaining groups reveal new knowledge from the data. Our method is validated on public benchmark datasets as well as a real electronic medical record database to identify risk groups from a set of potential Congestive Heart Failure (CHF) patients.
更多查看译文
关键词
patient clinical condition heterogeneity,congestive heart failure patients,electronic medical records,risk group analysis,risk-relevant medical features,patient risk stratification,electronic health records,learning (artificial intelligence),domain experts,group-specific risk prediction models,risk factors,risk analysis,electronic medical record database,incomplete knowledge,risgal,chf,semisupervised learning framework,patient care,patient cohort,personalized care plan design,risk scores,medical informatics,risk evaluation criteria,graph theory,homogeneous groups,patient risk group exploration,medical computing,semi-supervised learning,patient similarity graph,learning artificial intelligence
AI 理解论文
溯源树
样例
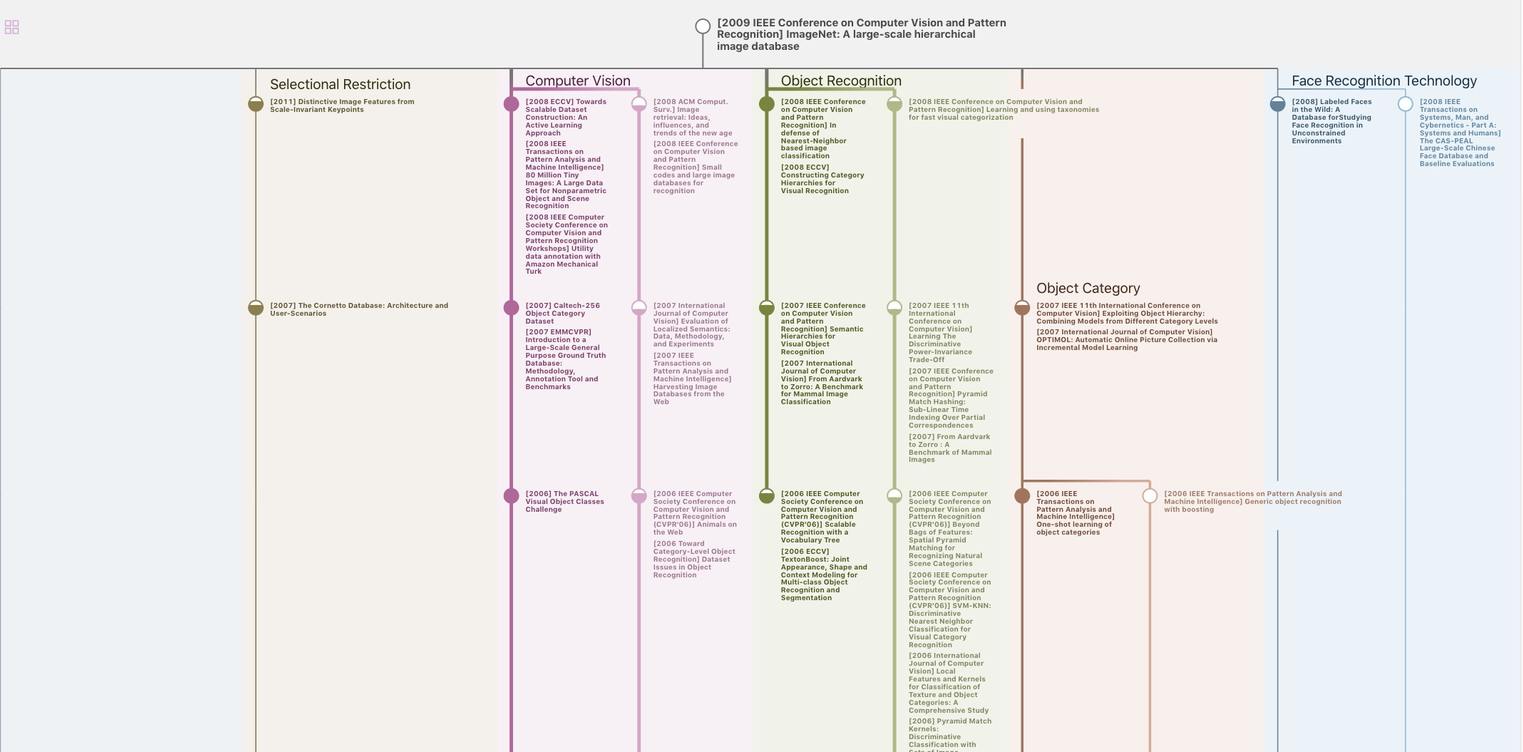
生成溯源树,研究论文发展脉络
Chat Paper
正在生成论文摘要