Ranking Techniques for Cluster Based Search Results in a Textual Knowledge-base
IC-AI(2009)
摘要
This paper presents a framework and methodology to improve the search experience in digital library systems. The approach taken is to cluster a textual knowledgebase along multiple relations and return search results in the form of small, focused clusters. Specifically, we generate multiple relationship networks, one per relationship type, and then cluster these networks. At search time, we present a ranked set of clusters—one ranking per relationship type. The intuition for this approach is that returning clusters of contextually related information provides users with a situational and contextual awareness of the search results rather than returning a ranked list of only those documents that match the query. We address the use of both implicit (such as textual content) and explicit (such as citations, authors etc.) relations between documents. The primary question we focus on is how to rank the clusters, given a search query. We explore two approaches: a text-based rank (using the text's similarity to the user's query) and a social network-based rank (using information centrality). A comparison of these two ranking methods suggest that using information centrality for ranking is very useful for ranking clusters and its documents because the documents that characterize that cluster get the highest rank.
更多查看译文
关键词
social network,knowledge base,digital library
AI 理解论文
溯源树
样例
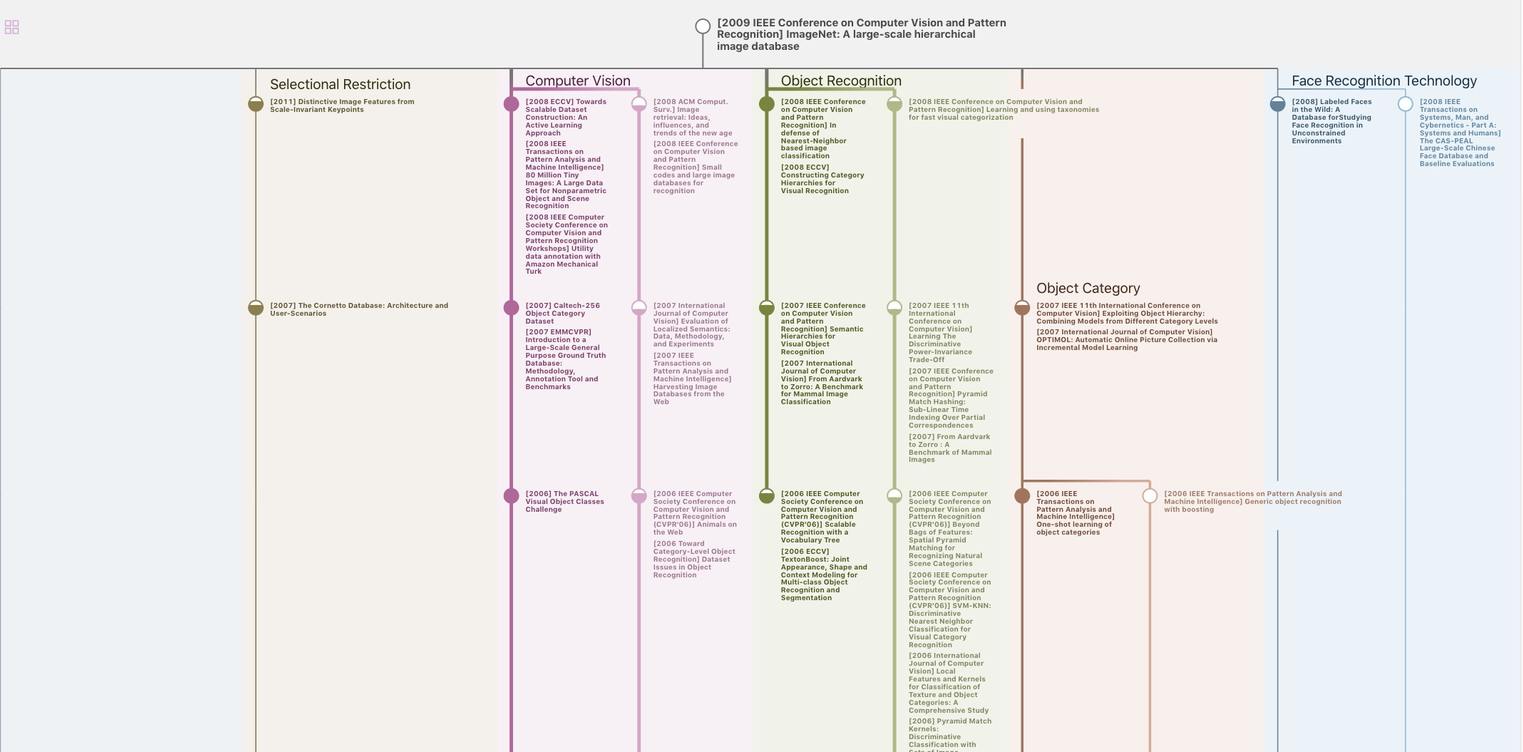
生成溯源树,研究论文发展脉络
Chat Paper
正在生成论文摘要