The dataminer's guide to scalable mixed-membership and nonparametric bayesian models
KDD(2013)
摘要
Large amounts of data arise in a multitude of situations, ranging from bioinformatics to astronomy, manufacturing, and medical applications. For concreteness our tutorial focuses on data obtained in the context of the internet, such as user generated content (microblogs, e-mails, messages), behavioral data (locations, interactions, clicks, queries), and graphs. Due to its magnitude, much of the challenges are to extract structure and interpretable models without the need for additional labels, i.e. to design effective unsupervised techniques. We present design patterns for hierarchical nonparametric Bayesian models, efficient inference algorithms, and modeling tools to describe salient aspects of the data.
更多查看译文
关键词
present design pattern,additional label,medical application,interpretable model,effective unsupervised technique,hierarchical nonparametric bayesian model,behavioral data,large amount,efficient inference algorithm,salient aspect,nonparametric bayesian model,association rule mining,clustering
AI 理解论文
溯源树
样例
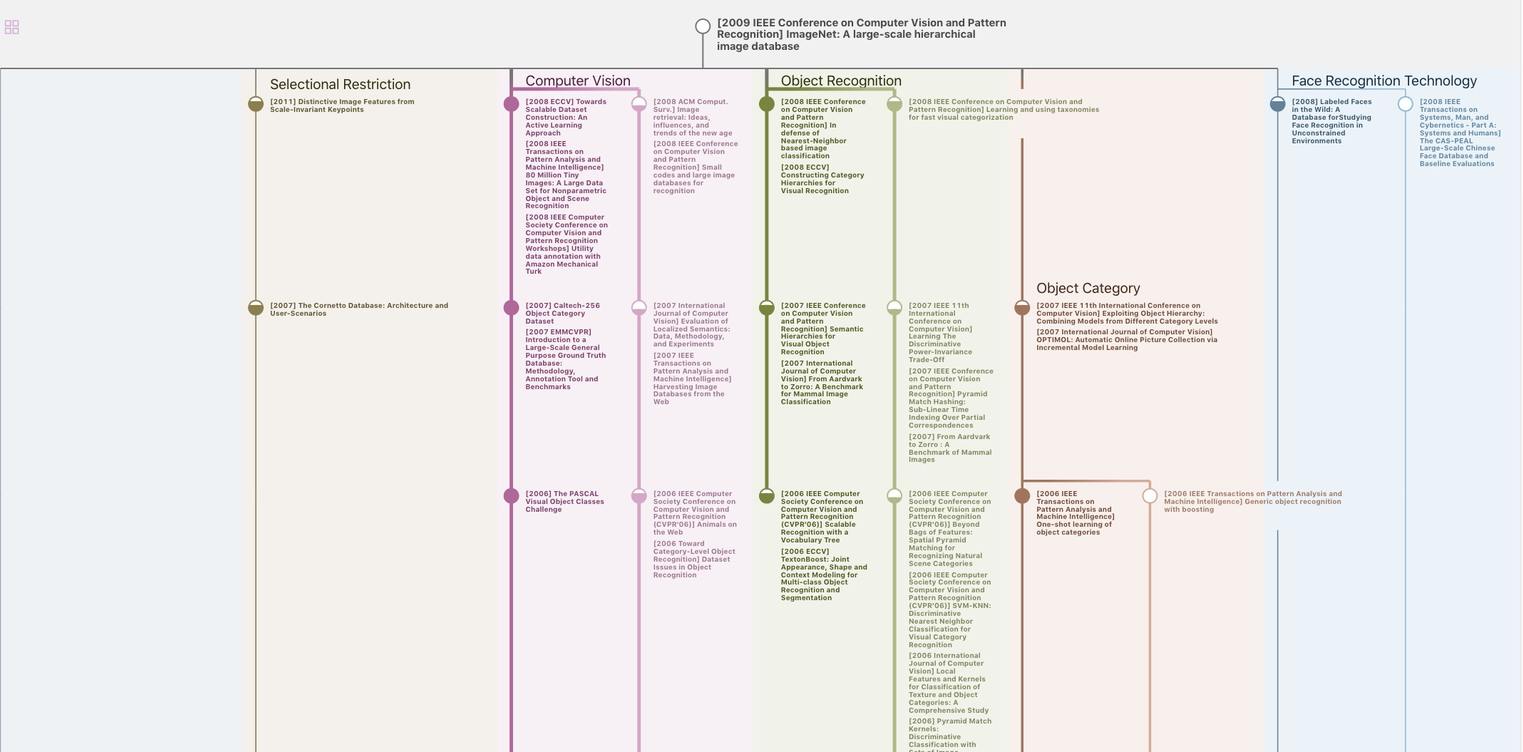
生成溯源树,研究论文发展脉络
Chat Paper
正在生成论文摘要