A data-driven model for predicting initial productivity of offshore directional well based on the physical constrained eXtreme gradient boosting (XGBoost) trees
Journal of Petroleum Science and Engineering(2022)
摘要
The initial productivity of directional oil wells is essential to research during the early development stage of offshore oilfields. Since the influence factors of productivity are numerous, the nonlinear relationship among them and productivity is hard to accurately describe by the physical models in practical application. The data-driven model provides an alternative way to deal with this problem, although it neglects the physical correlation between productivity and its influence factors. Therefore, for combining the advantage of the physical model and data-driven model, the reservoir engineering theory was used to constrain the data-driven method in this study. Based on the eXtreme gradient boosting (XGBoost) trees algorithm, this paper proposed a novel physical constrained data-driven model to predict the initial productivity. The reservoir engineering theory, including the productivity formula and the monotonic correlation between productivity and the input feature, was employed as the physical constraints in this model. Moreover, the Spearman Correlation Coefficient and a modified Recursive Feature Elimination were combined to develop a feature selection method for quantitatively selecting the main influence factors of the initial productivity. Based on the production and geological data from 87 wells, the permeability, completed thickness, oil viscosity, clay content, correct factor for interference, choke size, and drawdown were selected from 19 features as the main influence factors of the initial productivity. The prediction accuracy of the model proposed in this paper is 80.18%, which is better than the previous data-driven models. Furthermore, the physical constraints have been proved to improve the forecasting accuracy of the data-driven method by 10.09%.
更多查看译文
关键词
Initial productivity,Data-driven model,EXtreme gradient boosting trees,Physical constraints,Directional oil well
AI 理解论文
溯源树
样例
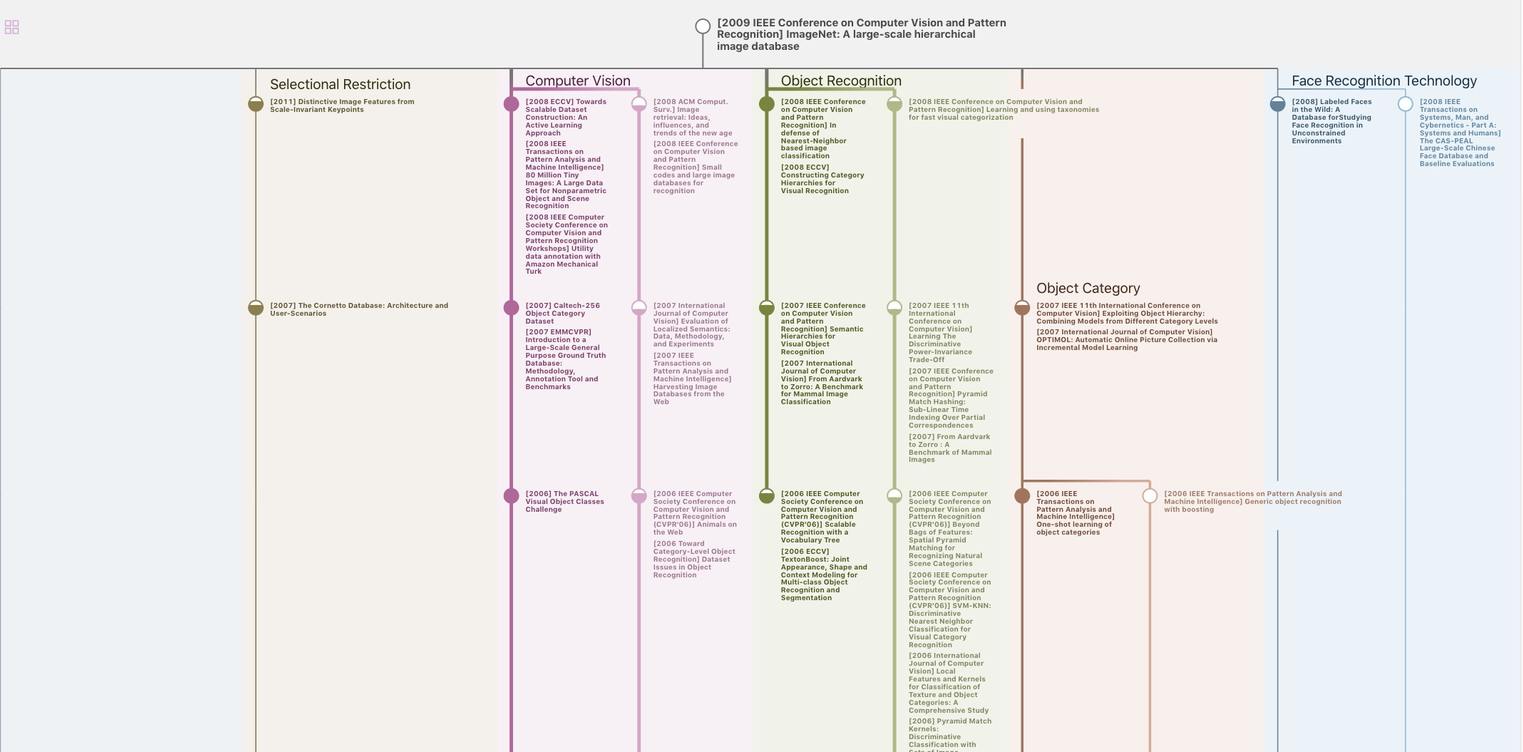
生成溯源树,研究论文发展脉络
Chat Paper
正在生成论文摘要