Content-Based Musical Similarity Computation using the Hierarchical Dirichlet Process
ISMIR 2013(2008)
摘要
We develop a method for discovering the latent structure in MFCC feature data using the Hierarchical Dirichlet Process (HDP). Based on this structure, we compute timbral simi- larity between recorded songs. The HDP is a nonparametric Bayesian model. Like the Gaussian Mixture Model (GMM), it represents each song as a mixture of some number of multivariate Gaussian distributions However, the number of mixture components is not fixed in the HDP, but is deter- mined as part of the posterior inference process. Moreover, in the HDP the same set of Gaussians is used to model all songs, with only the mixture weights varying from song to song. We compute the similarity of songs based on these weights, which is faster than previous approaches that com- pare single Gaussian distributions directly. Experimental re- sults on a genre-based retrieval task illustrate that our HDP- based method is both faster and produces better retrieval quality than such previous approaches.
更多查看译文
关键词
gaussian mixture model,hierarchical dirichlet process,gaussian distribution,bayesian model
AI 理解论文
溯源树
样例
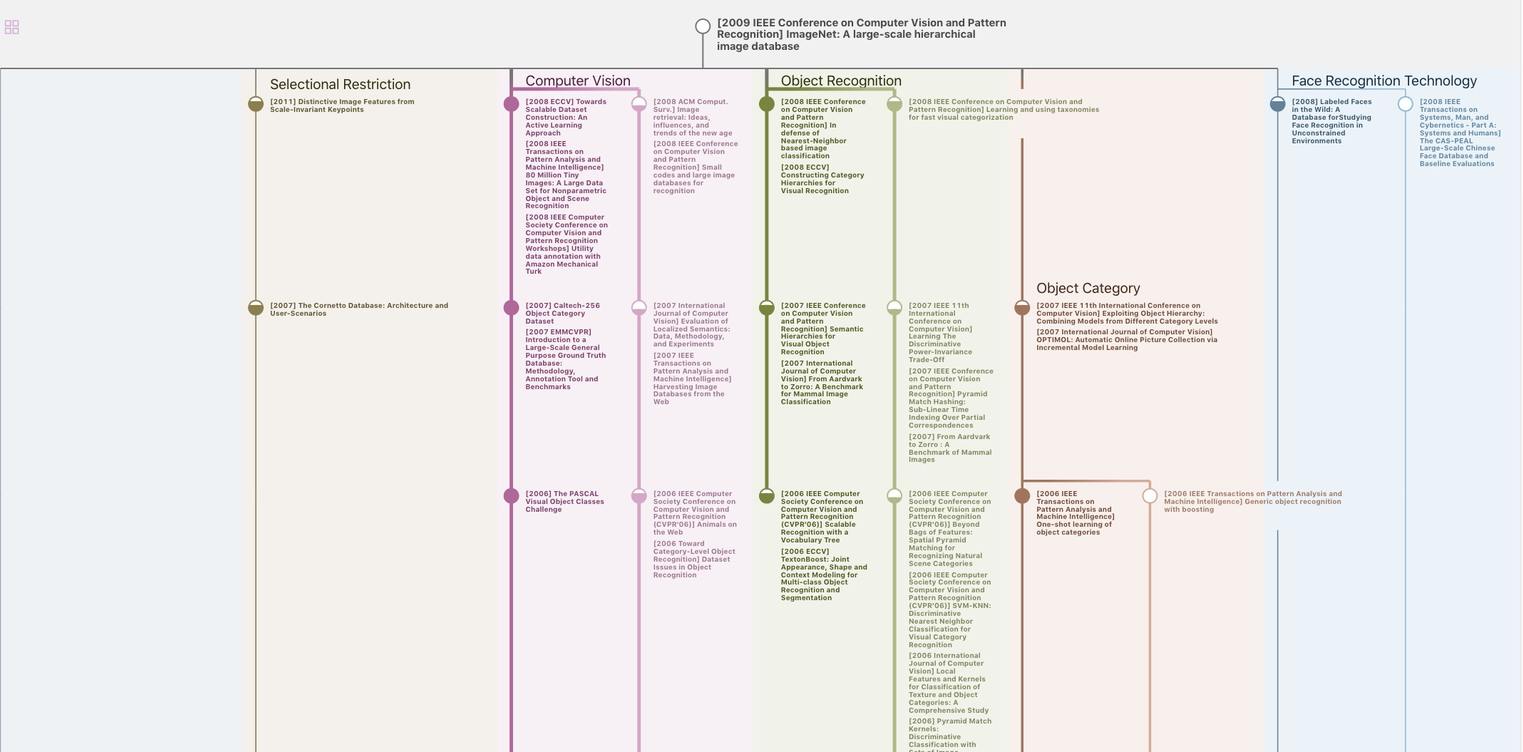
生成溯源树,研究论文发展脉络
Chat Paper
正在生成论文摘要