Evolution of the population of a genetic algorithm using particle swarm optimization: application to clustering analysis
Operational Research(2008)
摘要
This paper presents a new memetic algorithm, which is based on the concepts of genetic algorithms (GAs), particle swarm optimization (PSO) and greedy randomized adaptive search procedure (GRASP), for optimally clustering N objects into K clusters. The proposed algorithm is a two phase algorithm which combines a memetic algorithm for the solution of the feature selection problem and a GRASP algorithm for the solution of the clustering problem. In this paper, contrary to the genetic algorithms, the evolution of each individual of the population is realized with the use of a PSO algorithm where each individual have to improve its physical movement following the basic principles of PSO until it will obtain the requirements to be selected as a parent. Its performance is compared with other popular metaheuristic methods like classic genetic algorithms, tabu search, GRASP, ant colony optimization and PSO. In order to assess the efficacy of the proposed algorithm, this methodology is evaluated on datasets from the UCI Machine Learning Repository. The high performance of the proposed algorithm is achieved as the algorithm gives very good results and in some instances the percentage of the corrected clustered samples is very high and is larger than 96%.
更多查看译文
关键词
Clustering analysis,Feature selection problem,Memetic algorithms,Particle swarm optimization,GRASP
AI 理解论文
溯源树
样例
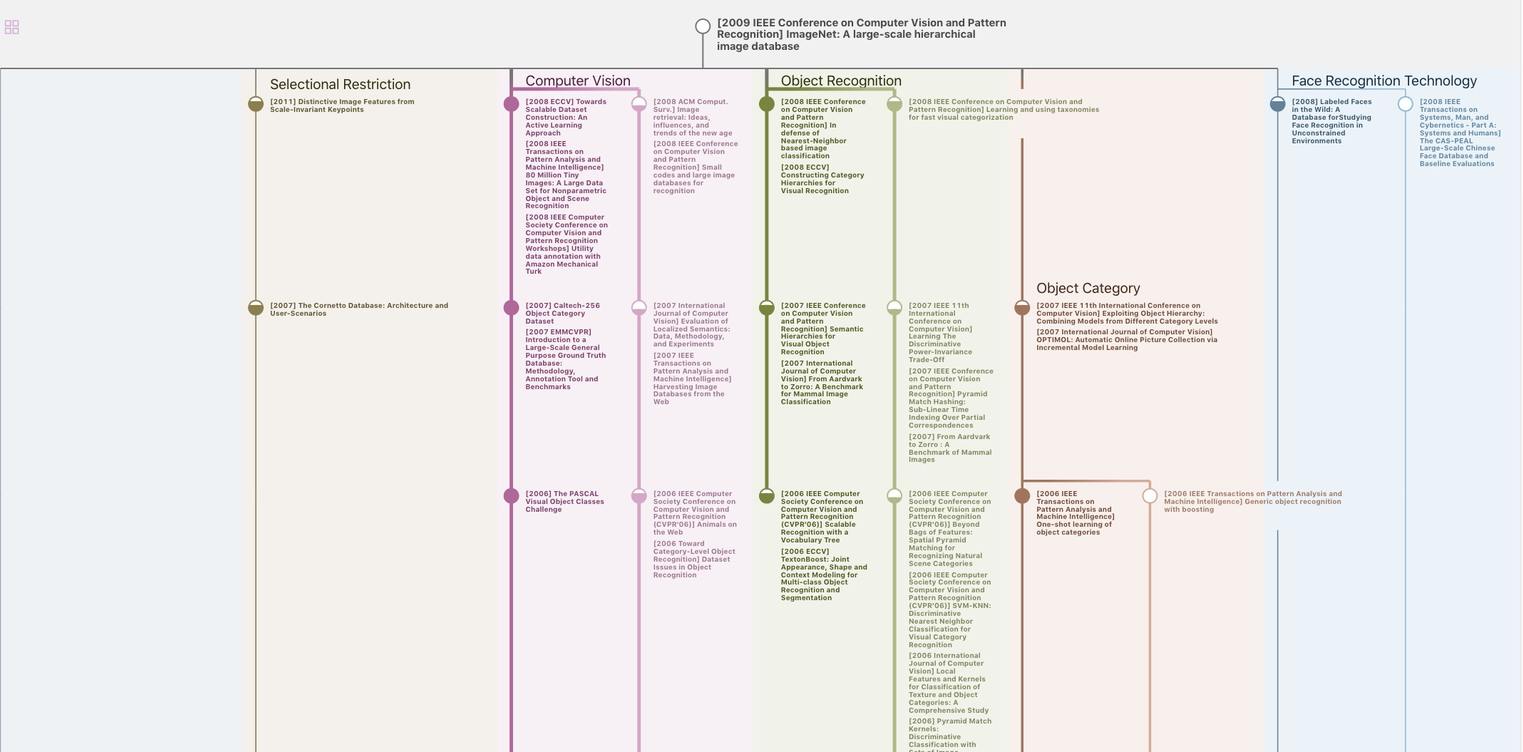
生成溯源树,研究论文发展脉络
Chat Paper
正在生成论文摘要