Cascading Spatio-temporal Pattern Discovery: A Summary of Results
SDM(2010)
摘要
Given a collection of Boolean spatio-temporal(ST) event types, the cascading spatio-temporal pattern (CSTP) dis- covery process finds partially ordered subsets of event-types whose instances are located together and occur in stages. For example, analysis of crime datasets may reveal frequent oc- currence of misdemeanors and drunk driving after bar clos- ings on weekends and after large gatherings such as football games. Discovering CSTPs from ST datasets is important for application domains such as public safety (e.g. crime at- tractors and generators) and natural disaster planning(e.g. hurricanes). However, CSTP discovery is challenging for sev- eral reasons, including both the lack of computationally ef- ficient, statistically meaningful metrics to quantify interest- ingness, and the large cardinality of candidate pattern sets that are exponential in the number of event types. Exist- ing literature for ST data mining focuses on mining totally ordered sequences or unordered subsets. In contrast, this pa- per models CSTPs as partially ordered subsets of Boolean ST event types. We propose a new CSTP interest measure (the Cascade Participation Index) that is computationally cheap(O(n 2 ) vs. exponential, where n is the dataset size) as well as statistically meaningful. We propose a novel al- gorithm exploiting the ST nature of datasets and evaluate filtering strategies to quickly prune uninteresting candidates. We present a case study to find CSTPs from real crime re- ports and provide a statistical explanation. Experimental results indicate that the proposed multiresolution spatio- temporal(MST) filtering strategy leads to significant savings in computational costs.
更多查看译文
关键词
theorems,indexation,time series analysis,total order,algorithms,natural disaster,partial order,statistical analysis,pattern recognition,data bases,information processing,data mining
AI 理解论文
溯源树
样例
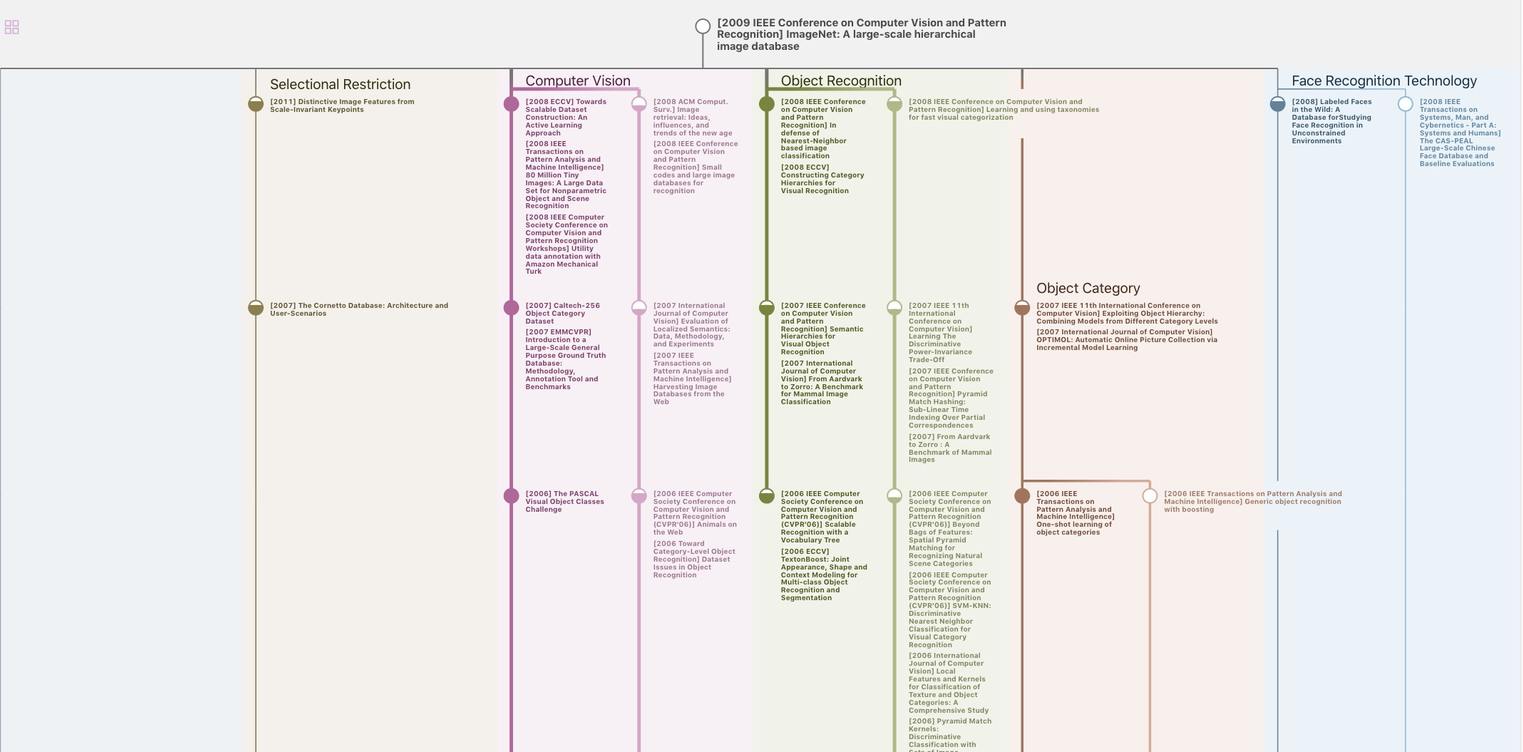
生成溯源树,研究论文发展脉络
Chat Paper
正在生成论文摘要