Comparative post fatigue residual property predictions of reinforced and unreinforced poly(ethylene terephthalate) fibers using artificial neural networks
Composites Part A: Applied Science and Manufacturing(2010)
摘要
A set of experiments has been performed on poly(ethylene terephthalate) (PET control) and PET fibers with vapor grown carbon nanofibers (PET–VGCNF) to assess the mechanical integrity of the materials due to a repeated cyclic loading. Artificial neural networks (ANNs) have been used to examine the residual strength and elastic modulus degradation behavior of the filaments as a function of the input mechanical testing variables (maximum fatigue stress – σmax, stress ratio – R, # cycles – N, undeformed modulus – E) and a damage variable that has been identified as the residual strain from fatigue, εR. The relationship of how these input variables relate to the degradation of the elastic modulus, E, and the fracture strength, σf, has been determined. The results indicate that ANNs can be used to predict the residual strength and modulus degradation behavior of PET and PET/VGCNF single filaments under various loading conditions. Backpropagation (BP) with momentum and conjugate gradient algorithms have been utilized to successfully train a multilayer perceptron (MLP) network for modeling the mechanical behavior of single polymeric filaments subsequent to fatigue loading. Also, the mechanical behavior of the PET control and PET–VGCNF differs as a function of the input fatigue conditions prescribed. The main difference was that the PET control samples exhibited a distinct hardening effect in the low residual strain limit and this was not observed for the PET–VGCNF samples. The neural networks used in this work were successful at replicating the hardening behavior for the PET control samples and the mechanical behavior changes for the PET–VGCNF samples as a function of σmax, R, and εR.
更多查看译文
关键词
A. Fibers,B. Nanocomposite,C. Fatigue,D. Neural networks,E. Residual strength
AI 理解论文
溯源树
样例
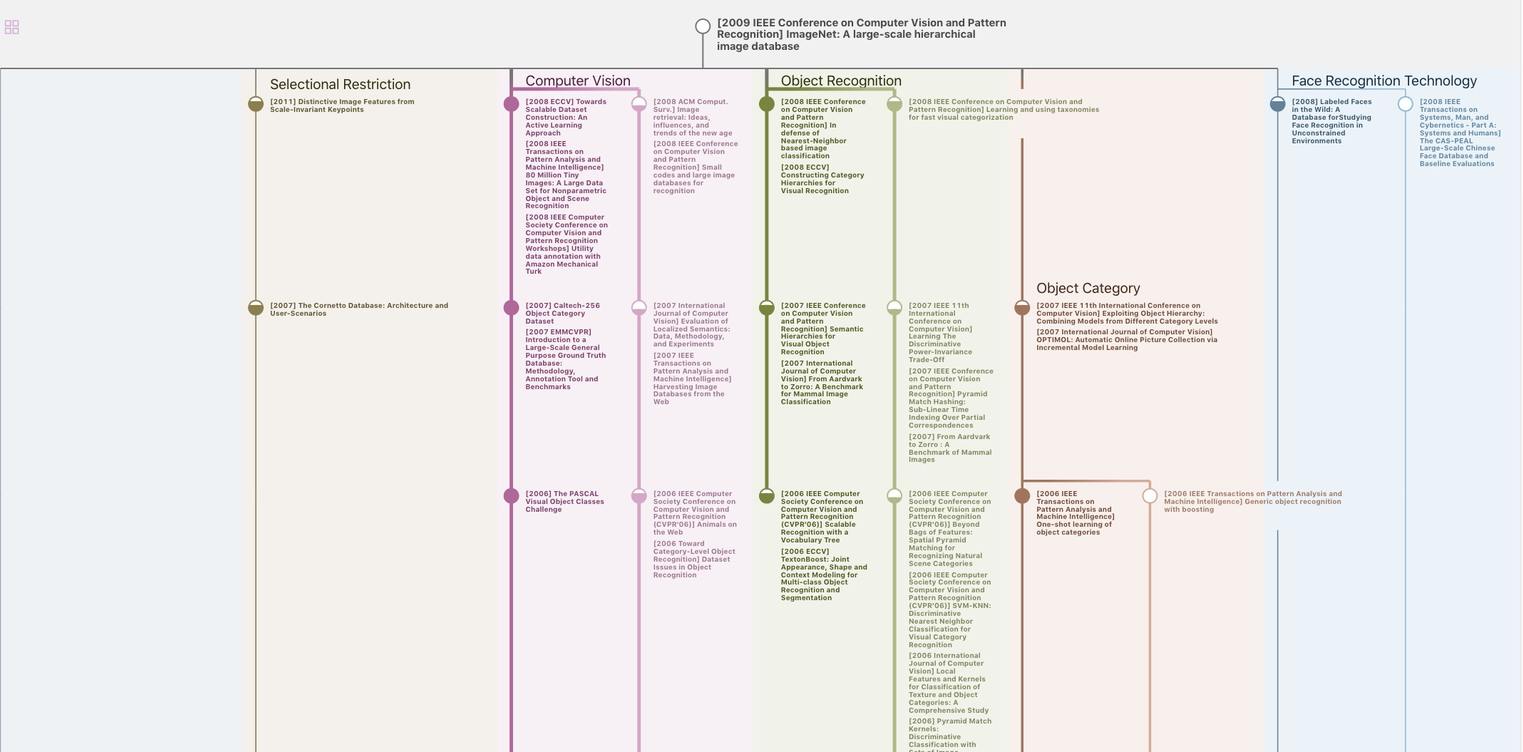
生成溯源树,研究论文发展脉络
Chat Paper
正在生成论文摘要