Structured prediction with indirect supervision
MLSLP(2011)
摘要
Structured tasks, which often involve many interdependent decisions for each example, are the backbone for many important applications such as natural language processing tasks. The models built for structured tasks need to be capable of assigning values to a set of interdependent variables. In this thesis, we point out that the strong dependencies between the decisions in structured tasks can be exploited to simplify both the learning task and the annotation effort – it is sometimes possible to supply partial and indirect supervision to only some of the target variables or to other variables that are derivatives of the target variables and thus reduce the supervision effort significantly. Based on this intuition, this thesis addresses the problem of reducing the cost of labeling for structural tasks. We tackle this problem by developing advanced machine learning algorithms that can learn and generalize from indirect supervision in addition to labeled data. Indirect supervision can come in the form of constraints or weaker supervision signals. Our proposed learning frameworks can handle both structured output problems and problems with latent structures. We demonstrate the effectiveness of the learning with indirect supervision framework for many natural language processing tasks.
更多查看译文
关键词
weaker supervision signal,interdependent decision,annotation effort,natural language processing task,indirect supervision framework,structured task,indirect supervision,structured prediction,structured output problem,supervision effort,target variable
AI 理解论文
溯源树
样例
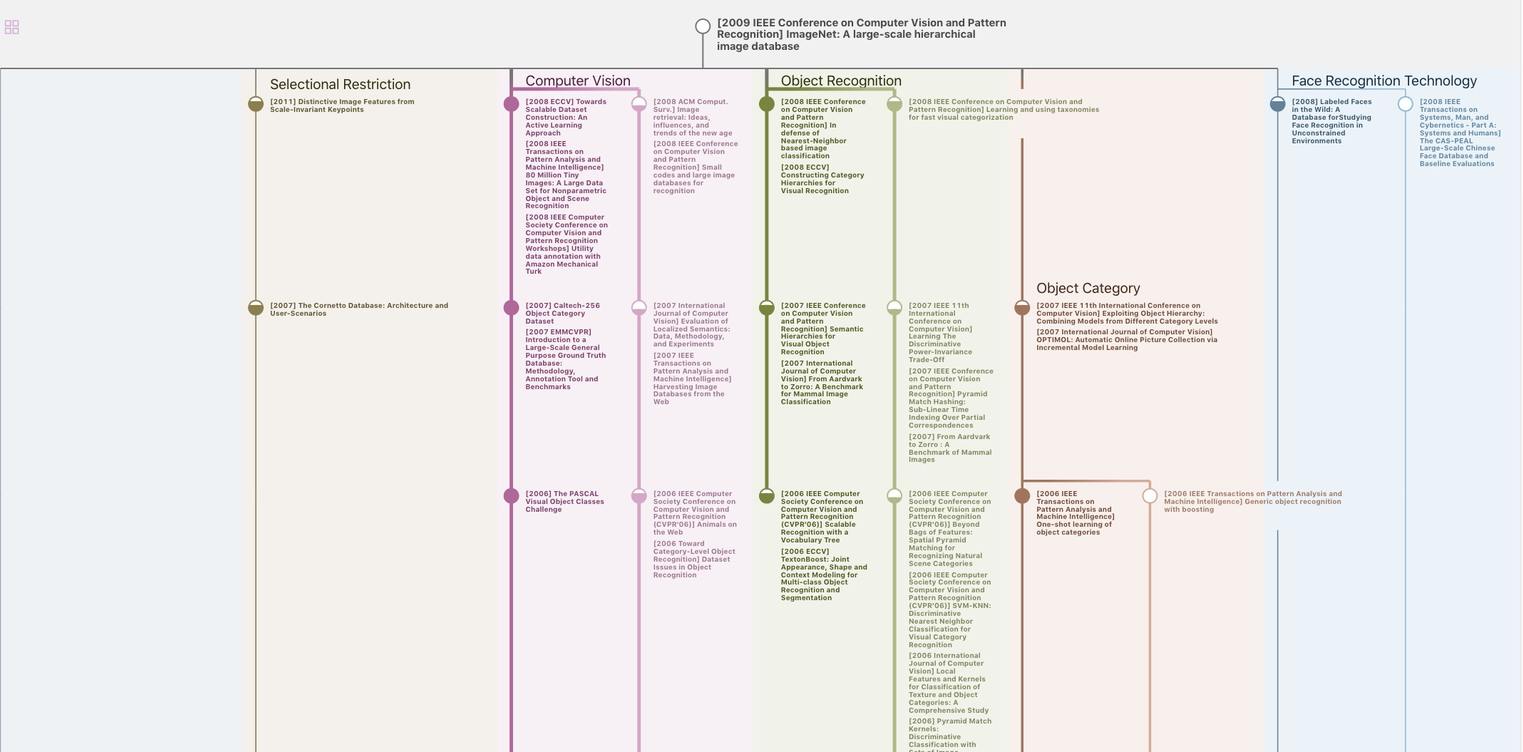
生成溯源树,研究论文发展脉络
Chat Paper
正在生成论文摘要