Multi-view forests based on Dempster-Shafer evidence theory: a new classifier ensemble method
SPPRA '08: Proceedings of the Fifth IASTED International Conference on Signal Processing, Pattern Recognition and Applications(2008)
摘要
In this paper, a method to construct ensembles of tree-structured classifiers using multi-view learning instead of feature subset selection is proposed. An essential requirement to train an effective classifier ensemble is the diversity among the base individual classifiers. In order to construct diverse individual classifiers, it is assumed that the object to be classified is represented through multiple feature sets (views). Different views of the data can then be combined to improve the accuracy of the learning task. Multi-view Forests have been examined on a benchmark data set of 3D-object recognition based on 2D camera images. Results show that multi-view learning can improve the performance of the individual classifiers and the whole ensemble, and thus promoting the use of multi-view forests.
更多查看译文
关键词
base individual classifier,diverse individual classifier,individual classifier,multi-view forest,multi-view learning,benchmark data,effective classifier ensemble,feature subset selection,multiple feature set,whole ensemble,Dempster-Shafer evidence theory,Multi-view forest,new classifier ensemble method
AI 理解论文
溯源树
样例
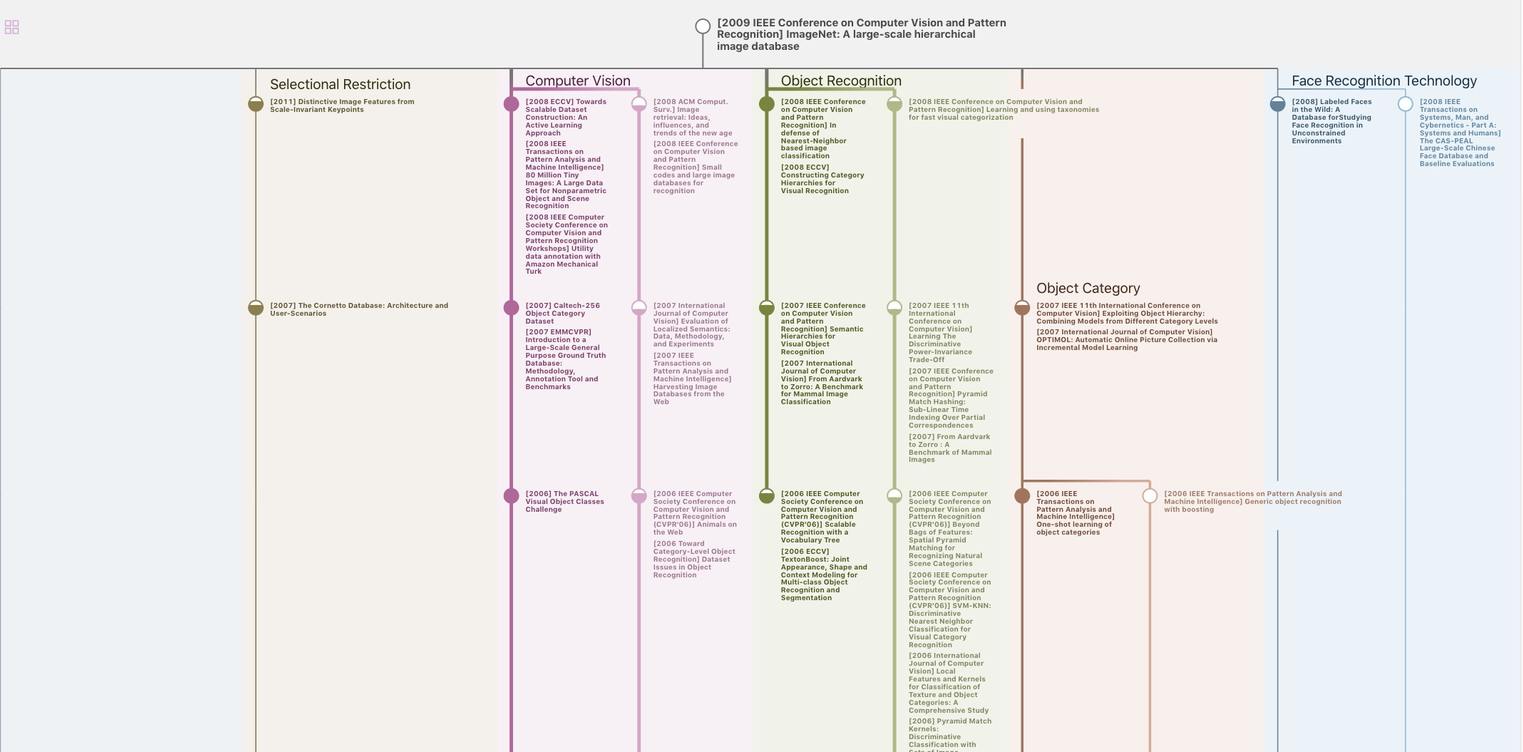
生成溯源树,研究论文发展脉络
Chat Paper
正在生成论文摘要