Deterministic Statistical Mapping Of Sentences To Underspecified Semantics
IWCS '11: Proceedings of the Ninth International Conference on Computational Semantics(2014)
摘要
We present a method for training a statistical model for mapping natural language sentences to semantic expressions. The semantics are expressions of an underspecified logical form that has properties making it particularly suitable for statistical mapping from text. An encoding of the semantic expressions into dependency trees with automatically generated labels allows application of existing methods for statistical dependency parsing to the mapping task (without the need for separate traditional dependency labels or parts of speech). The encoding also results in a natural per-word semantic-mapping accuracy measure. We report on the results of training and testing statistical models for mapping sentences of the Penn Treebank into the semantic expressions, for which per-word semantic mapping accuracy ranges between 79 % and 86 % depending on the experimental conditions. The particular choice of algorithms used also means that our trained mapping is deterministic (in the sense of deterministic parsing), paving the way for large-scale text-to-semantic mapping.
更多查看译文
关键词
semantic expression,statistical model,large-scale text-to-semantic mapping,mapping sentence,mapping task,semantic mapping accuracy range,statistical mapping,trained mapping,statistical dependency,dependency tree,Deterministic statistical mapping,underspecified semantics
AI 理解论文
溯源树
样例
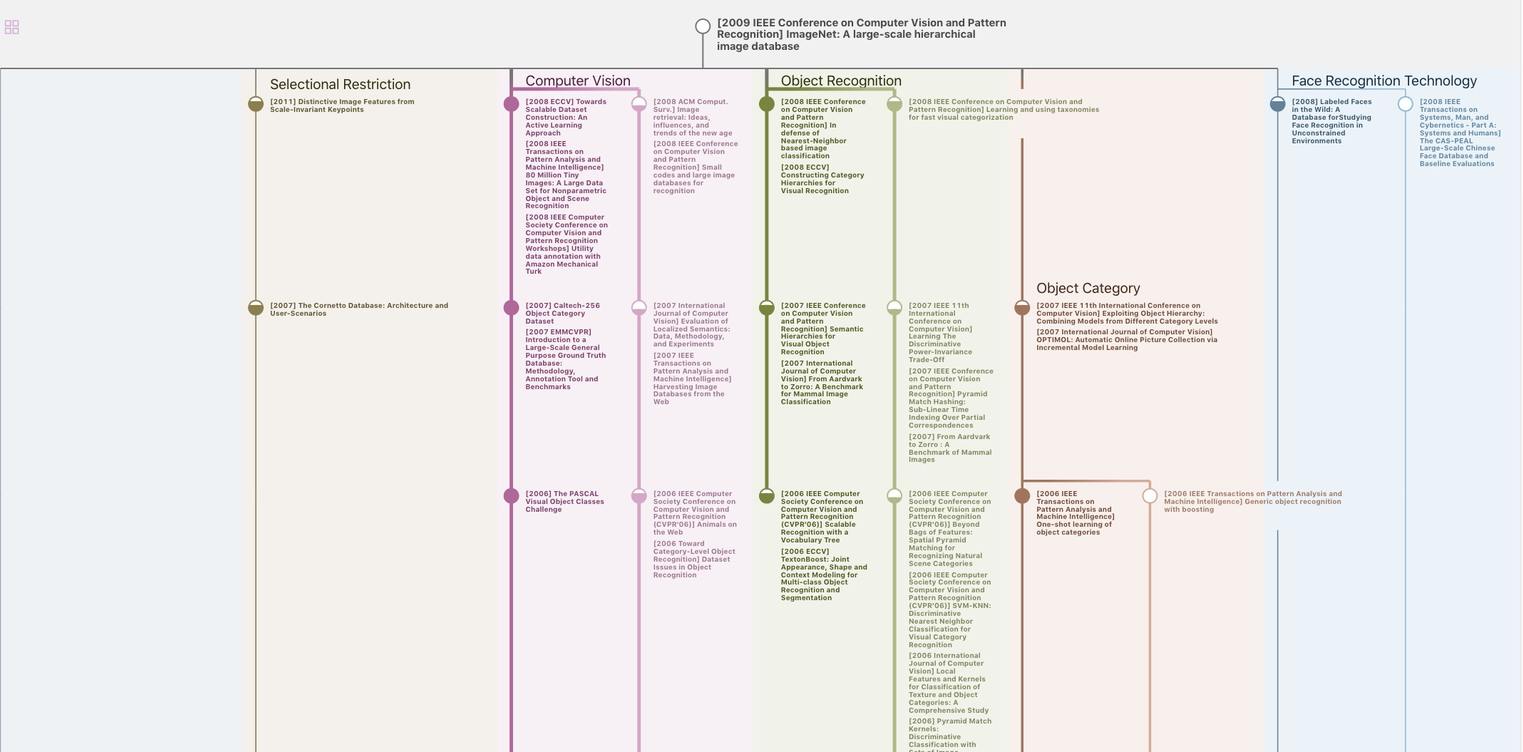
生成溯源树,研究论文发展脉络
Chat Paper
正在生成论文摘要