A Parallel Multi-Objective Evolutionary Algorithm For Phylogenetic Inference
LION'10: Proceedings of the 4th international conference on Learning and intelligent optimization(2010)
摘要
The increasing availability of large sequence data proposes new challenges for phylogenetic reconstruction. The search and evaluation of these datasets largely surpass the memory and processing capability of a single machine. In this context, parallel and distributed computing can be used not only to speedup the search, but also to improve the solution quality, search robustness and to solve larger problem instances [1]. On the other hand, it has been shown that applying distinct reconstruction methods to the same input data can generate conflicting trees [2,3]. In this regard, a multi-objective approach can be a relevant contribution since it can search for phylogenies using more than a single criterion. One of the first studies that models phylogenetic inference as a multi-objective optimization problem (MOOP) was developed by the author of this paper [4]. In this approach, the multi-objective approach used the maximum parsimony (MP) and maximum likelihood (ML) as optimality criteria [5]. The proposed multi-objective evolutionary algorithm (MOEA) [6], called PhyloMOEA, produces a set of distinct solutions representing a trade-off between the considered objectives. In this paper, we present a new parallel PhyloMOEA version developed using the ParadisEO metaheuristic framework [7].
更多查看译文
关键词
Phylogenetic Inference,Multi-Objective Optimization,Parallel Computing
AI 理解论文
溯源树
样例
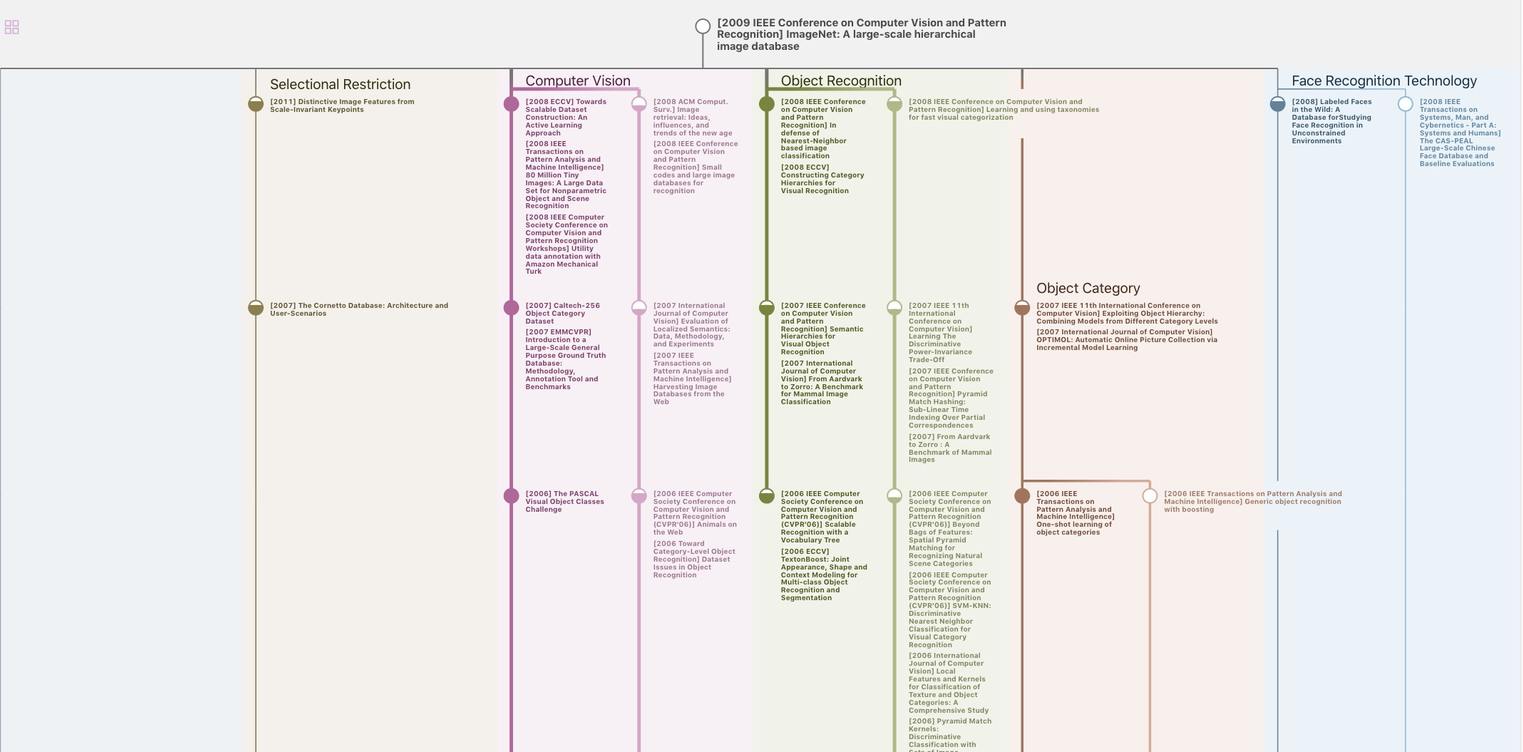
生成溯源树,研究论文发展脉络
Chat Paper
正在生成论文摘要