Longitudinal aggregate data model-based meta-analysis with NONMEM: approaches to handling within treatment arm correlation
Journal of Pharmacokinetics and Pharmacodynamics(2010)
摘要
Literature data are often reported as multiple (longitudinal) mean outcomes observed in several groups of patients within a study. Observations within a study are correlated because the patients come from a common population, and the mean observations over time within a treatment arm are correlated because they are based on the same set of patients. As a result, model-based meta-analysis may require more than two levels of random effects to correctly characterize this correlation structure. Using simulation, we explored and evaluated ways to implement multi-level random effects in NONMEM. Simulation models that were linear and non-linear in the random effects were investigated. We compared estimation models that included study and/or treatment arm-level random effects, with and without residual correlation. With all estimation strategies, the fixed random effects parameters were accurately estimated. With regard to correctly characterizing the variability, models that accounted for correlation within a study and treatment arm over time were the best in some situations, while models that accounted for study-level correlation only were better in others. Models that included only treatment arm-level random effects were not superior in any scenario.
更多查看译文
关键词
Model-based meta-analysis,Longitudinal data,Multi-level random effects,Correlation,NONMEM
AI 理解论文
溯源树
样例
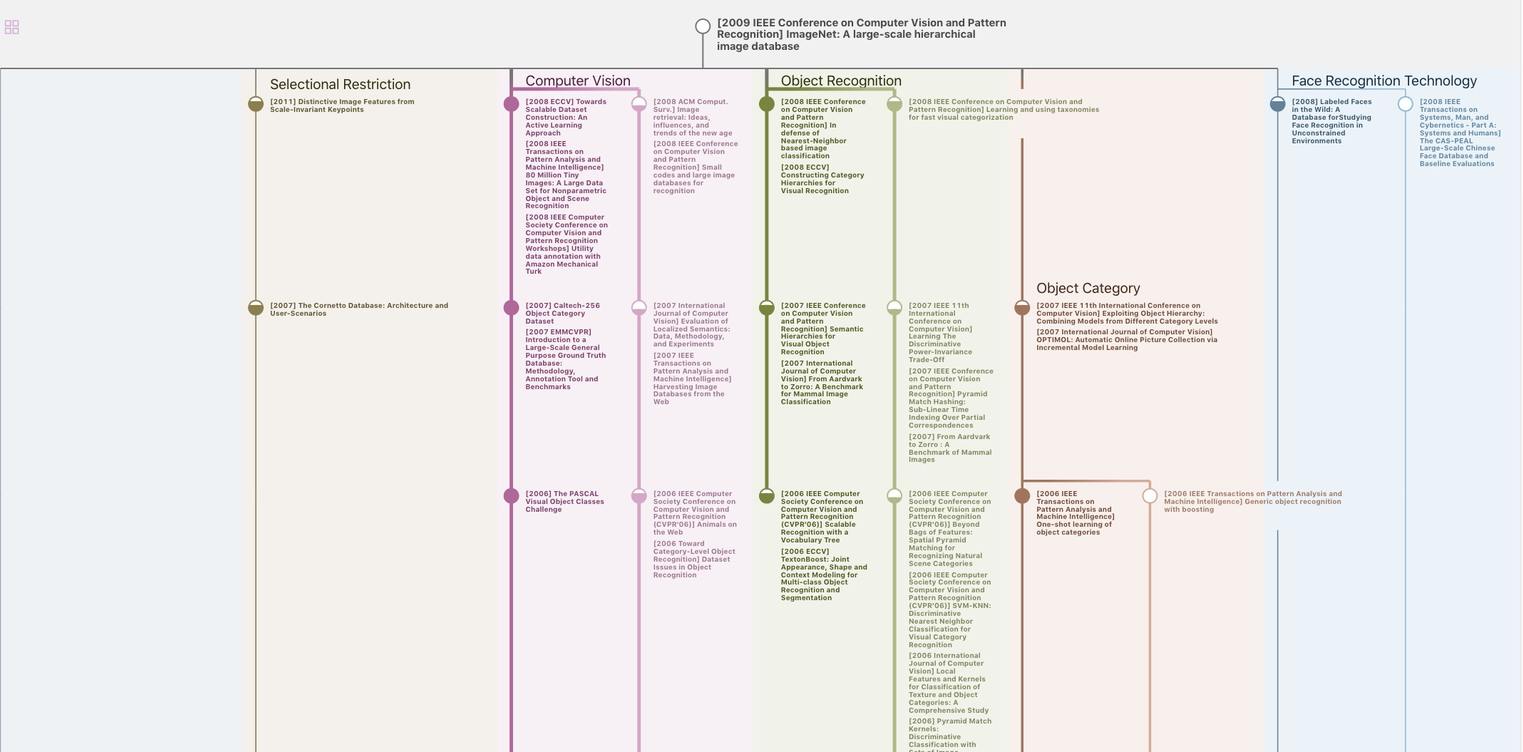
生成溯源树,研究论文发展脉络
Chat Paper
正在生成论文摘要