Conversational case-based reasoning in medical decision making.
Artificial Intelligence in Medicine(2011)
摘要
Balancing the trade-offs between solution quality, problem-solving efficiency, and transparency is an important challenge in medical applications of conversational case-based reasoning (CCBR). For example, test selection in CCBR is often based on strategies in which the absence of a specific hypothesis (e.g., diagnosis) to be confirmed makes it difficult to explain the relevance of test results that users are asked to provide. In this paper, we present an approach to CCBR in medical classification and diagnosis that aims to increase transparency while also providing high levels of accuracy and efficiency.We present an algorithm for CCBR called iNN(k) in which feature selection is driven by the goal of confirming a target class and informed by a measure of a feature's discriminating power in favor of the target class. As we demonstrate in a CCBR system called CBR-Confirm, this enables a CCBR system to explain the relevance of any question it asks the user. We evaluate the algorithm's accuracy and efficiency on a selection of datasets related to medicine and health care.The performance of iNN(k) on a given dataset is shown to depend on the value of k and on whether local or global feature selection is used in the algorithm. The combination of these parameters for which iNN(k) is most effective in addressing the trade-off between accuracy and efficiency is identified for each of the selected datasets. For example, only 42% and 51% on average of features in a complete problem description were needed by iNN(k) to provide accuracy levels of 86.5% and 84.3% respectively on the lymphography and SPECT heart datasets from the UCI machine learning repository.Our results demonstrate the ability of iNN(k) to provide high levels of accuracy on most of the selected datasets, while often requiring the user to provide only a small subset of the features in a complete problem description, and enabling a CCBR system to explain the relevance of any question it asks the user.
更多查看译文
关键词
Conversational case-based reasoning,Feature selection,Explanation of reasoning,Transparency,Medical classification and diagnosis
AI 理解论文
溯源树
样例
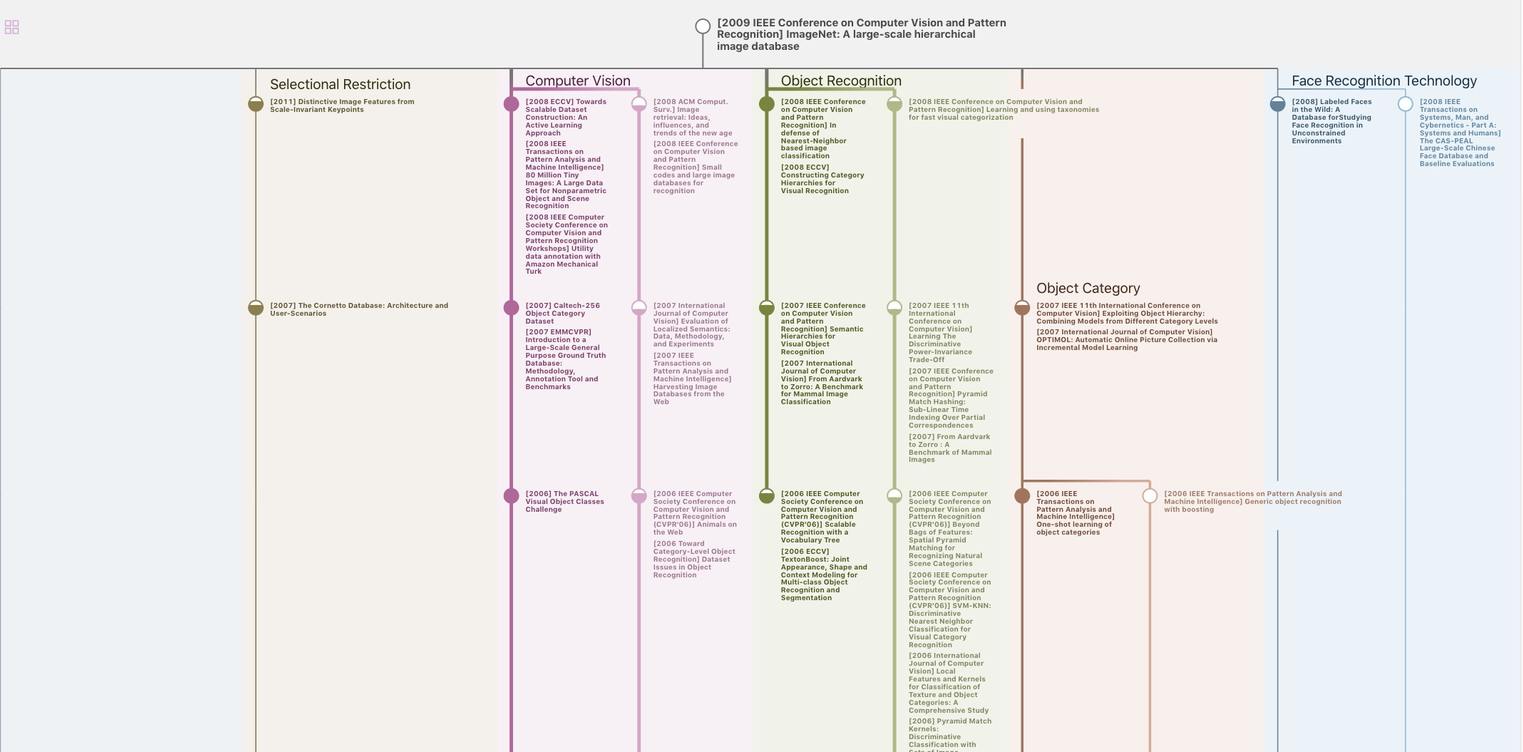
生成溯源树,研究论文发展脉络
Chat Paper
正在生成论文摘要