Learning Effective Event Models to Recognize a Large Number of Human Actions
IEEE Transactions on Multimedia(2014)
摘要
Human action recognition in videos is an important problem in computer vision, but it is very challenging, especially when recognizing a large number of human actions. First, it is difficult to capture the crucial motion patterns that discriminate among these actions. Second, the method should be scalable for large datasets because more training examples are often collected for more action classes. In this paper, we employ latent models to capture the crucial motion patterns, and we propose an effective learning algorithm that can efficiently address large datasets. To capture the crucial motion patterns, we define an “event” for each category, and we add a latent variable that indicates the start of the event. The event has a length of several frames that can differ across the categories. To train effective latent models for a large number of action classes, we employ a multi-class formulation with latent variables, and we address this problem by solving a dual quadratic programming (QP) problem with linear inequality constraints. To make the algorithm scalable for large datasets, we propose an improved QP solver that converges quickly for large QP problems that have a very large number of linear inequality constraints in real-world applications. We examine the proposed approach on the HMDB51 and UCF50 datasets. Comparison results have been reported to demonstrate the effectiveness of the proposed technique. Our approach outperforms state-of-the-art results for both datasets.
更多查看译文
关键词
Videos,Support vector machines,Training,Hidden Markov models,Feature extraction,Quadratic programming,Visualization
AI 理解论文
溯源树
样例
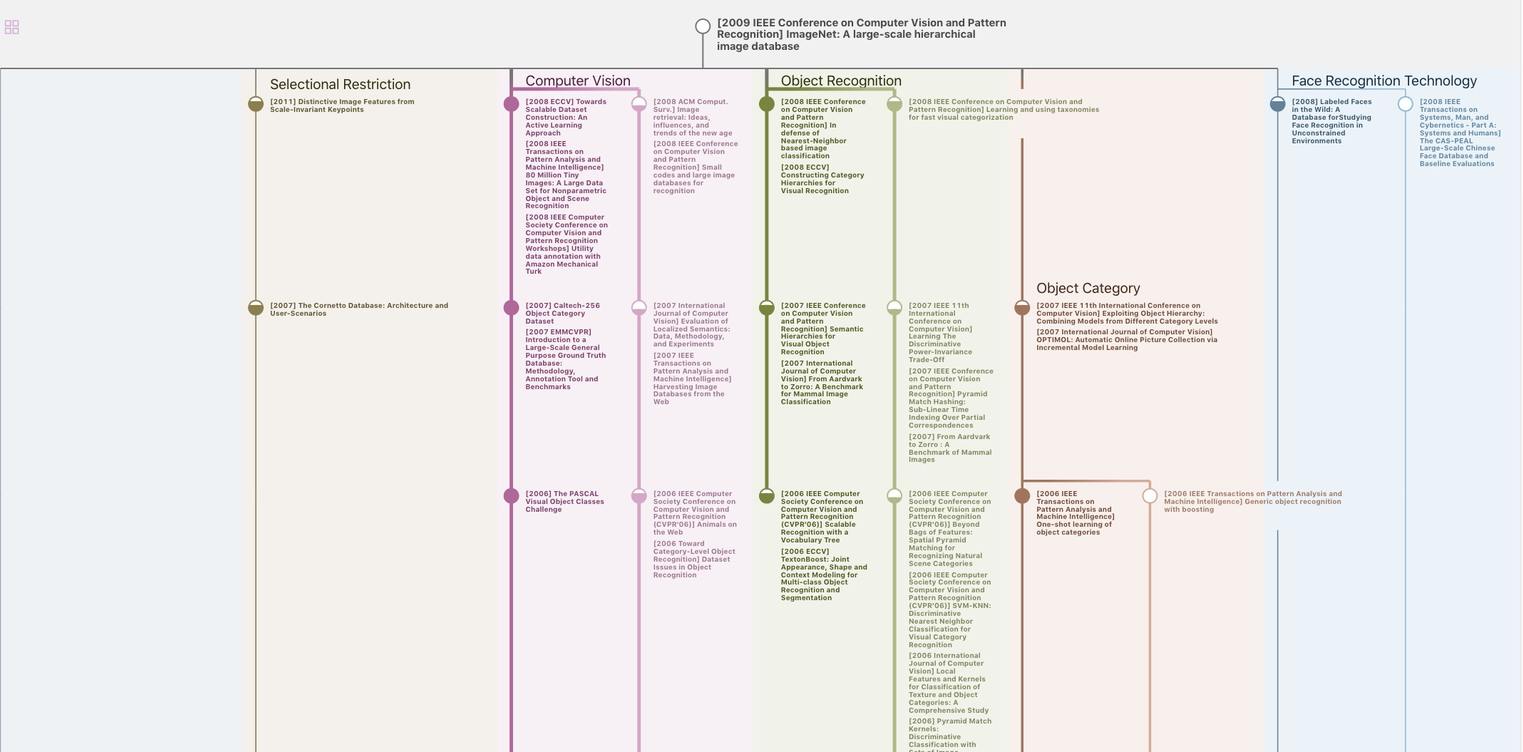
生成溯源树,研究论文发展脉络
Chat Paper
正在生成论文摘要