Viterbi Training Improves Unsupervised Dependency Parsing.
CoNLL '10: Proceedings of the Fourteenth Conference on Computational Natural Language Learning(2010)
摘要
We show that Viterbi (or "hard") EM is well-suited to unsupervised grammar induction. It is more accurate than standard inside-outside re-estimation (classic EM), significantly faster, and simpler. Our experiments with Klein and Manning's Dependency Model with Valence (DMV) attain state-of-the-art performance --- 44.8% accuracy on Section 23 (all sentences) of the Wall Street Journal corpus --- without clever initialization; with a good initializer, Viterbi training improves to 47.9%. This generalizes to the Brown corpus, our held-out set, where accuracy reaches 50.8% --- a 7.5% gain over previous best results. We find that classic EM learns better from short sentences but cannot cope with longer ones, where Viterbi thrives. However, we explain that both algorithms optimize the wrong objectives and prove that there are fundamental disconnects between the likelihoods of sentences, best parses, and true parses, beyond the well-established discrepancies between likelihood, accuracy and extrinsic performance.
更多查看译文
关键词
classic EM,Viterbi training,Brown corpus,Wall Street Journal corpus,best parses,extrinsic performance,previous best result,state-of-the-art performance,true parses,Dependency Model,unsupervised dependency parsing
AI 理解论文
溯源树
样例
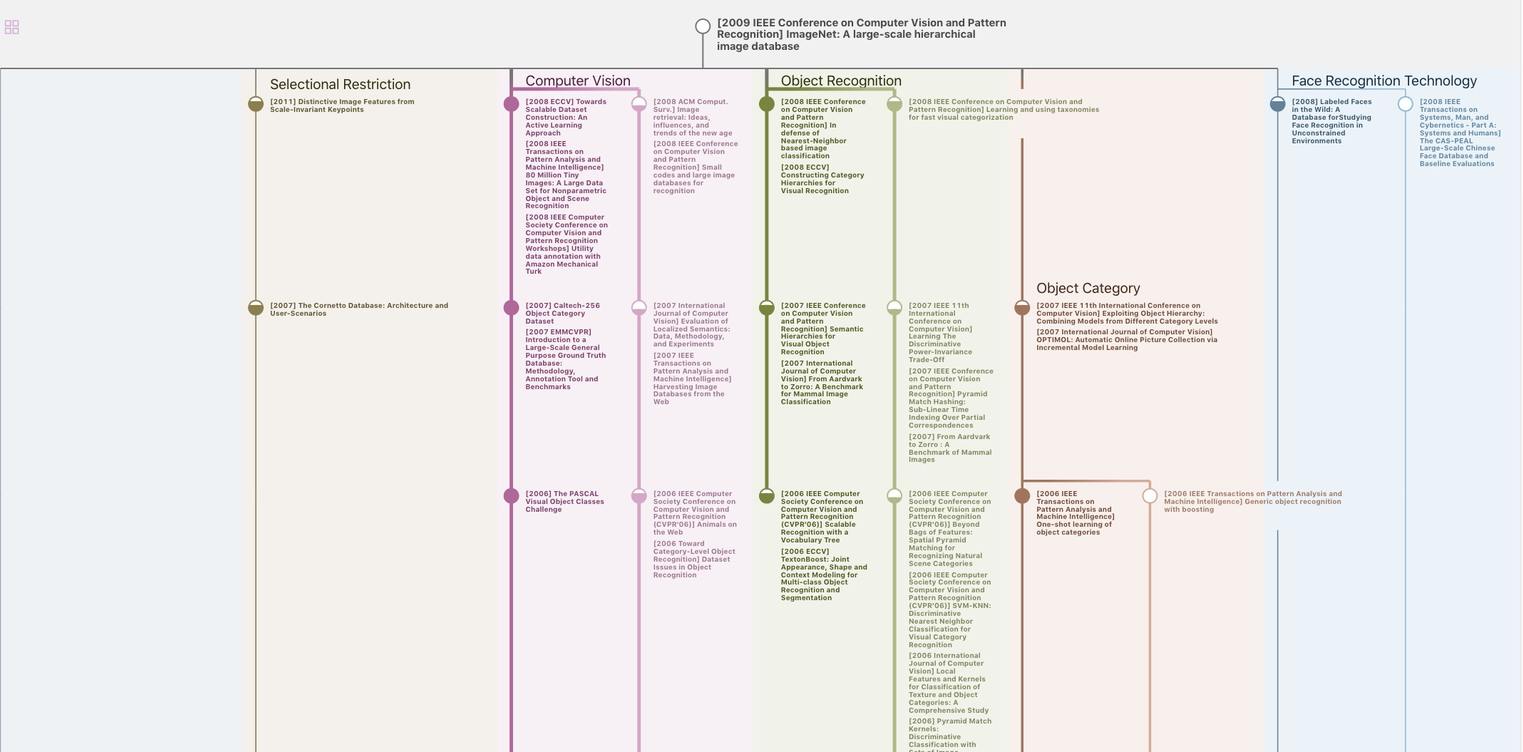
生成溯源树,研究论文发展脉络
Chat Paper
正在生成论文摘要