Optimizing Linear Counting Queries Under Differential Privacy
MOD(2010)
摘要
Differential privacy is a robust privacy standard that has been successfully applied to a range of data analysis tasks. But despite much recent work, optimal strategies for answering a collection of related queries are not known.We propose the matrix mechanism, a new algorithm for answering a workload of predicate counting queries. Given a workload, the mechanism requests answers to a different set of queries, called a query strategy, which are answered using the standard Laplace mechanism. Noisy answers to the workload queries are then derived from the noisy answers to the strategy queries. This two stage process can result in a more complex correlated noise distribution that preserves differential privacy but increases accuracy.We provide a formal analysis of the error of query answers produced by the mechanism and investigate the problem of computing the optimal query strategy in support of a given workload. We show this problem can be formulated as a rank-constrained semidefinite program. Finally, we analyze two seemingly distinct techniques, whose similar behavior is explained by viewing them as instances of the matrix mechanism.
更多查看译文
关键词
private data analysis,output perturbation,differential privacy,sernidefinite program
AI 理解论文
溯源树
样例
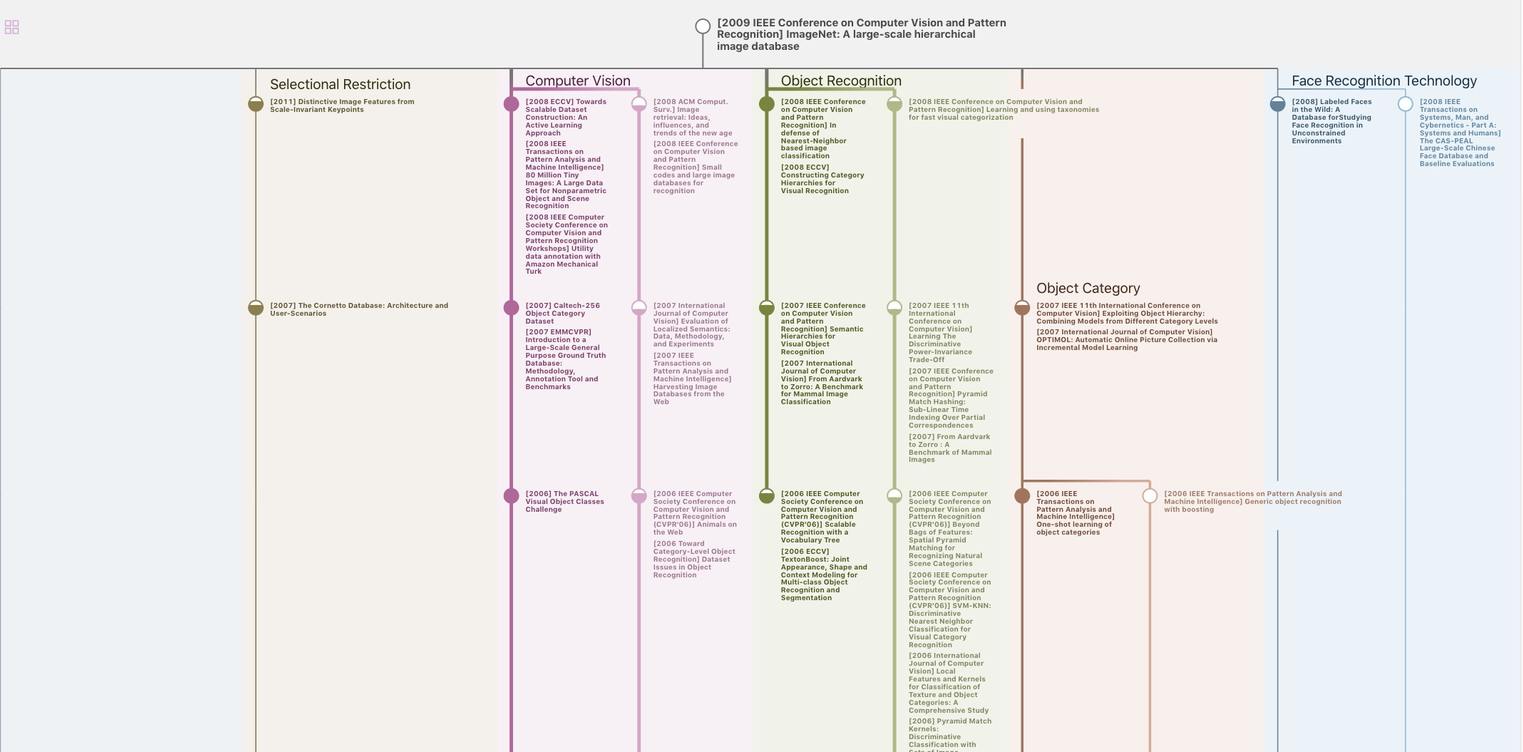
生成溯源树,研究论文发展脉络
Chat Paper
正在生成论文摘要