Instance-Based Action Models for Fast Action Planning
national conference on artificial intelligence(2007)
摘要
Two main challenges of robot action planning in real domains are uncertain action effects and dynamic environments. In this paper, an instance-basedaction model is learned empirically by robots trying actions in the environment. Modeling the action planning problem as a Markov decision process, the action model is used to build the transition function. In static environments, standard value iteration techniques are used for computing the optimal policy. In dynamic environments, an algorithm is proposed for fast replanning, which updates a subset of state-action values computed for the static environment. As a test-bed, the goal scoring task in the RoboCup 4-legged scenario is used. The algorithms are validated in the problem of planning kicks for scoring goals in the presence of opponent robots. The experimental results both in simulation and on real robots show that the instance-based action model boosts performance over using parametric models as done previously, and also incremental replanning significantly improves over original off-line planning.
更多查看译文
关键词
instance-based action models,parametric model,fast action planning,action planning problem,static environment,instance-basedaction model,action model,uncertain action effect,original off-line planning,instance-based action model,dynamic environment,robot action planning,value iteration,markov decision process,test bed
AI 理解论文
溯源树
样例
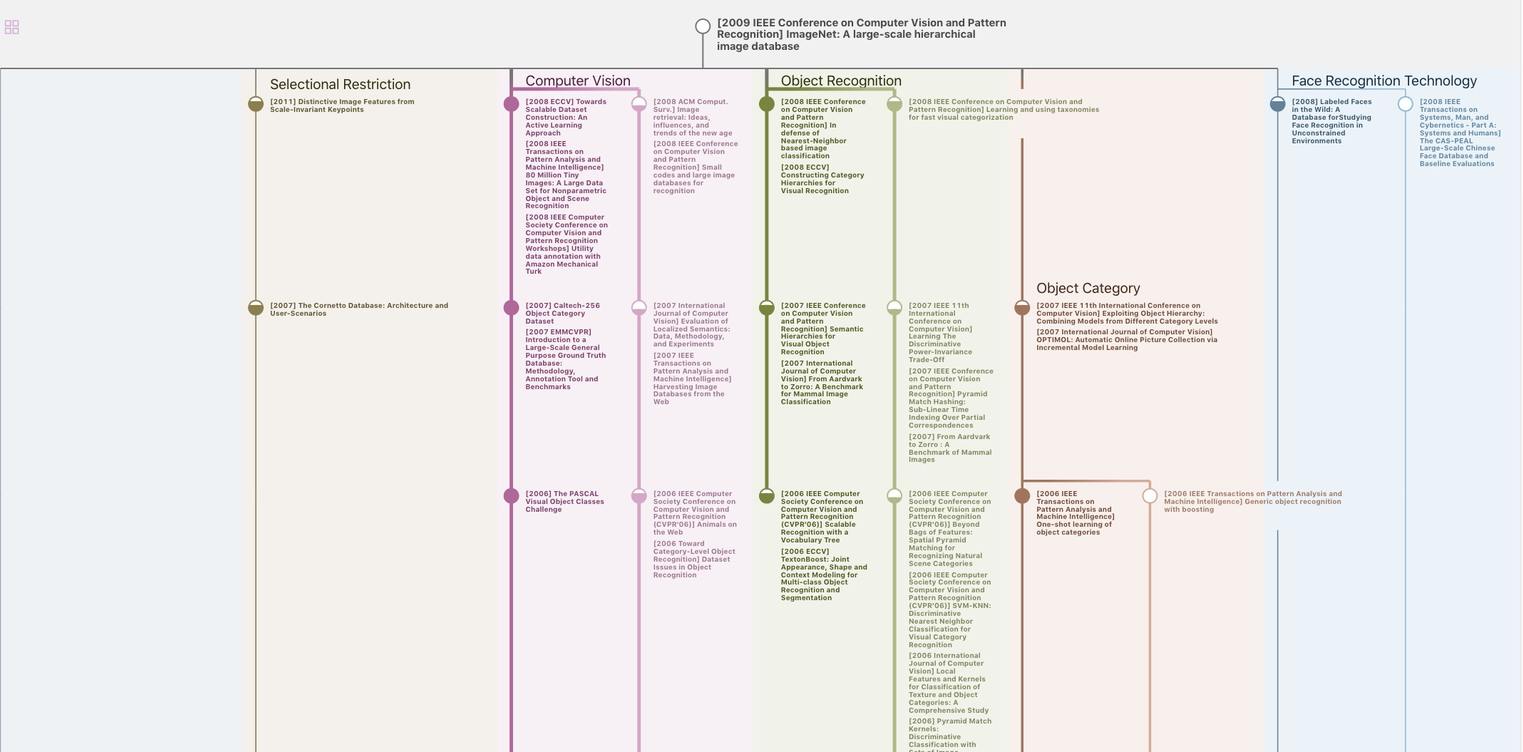
生成溯源树,研究论文发展脉络
Chat Paper
正在生成论文摘要