Distributed Learning Mechanism Against Flooding Network Attacks
msra(2008)
摘要
Adaptive techniques based on machine learning and data mining are gaining relevance in self- management and self-defense for networks and dis- tributed systems. In this paper, we focus on early detection and stopping of distributed flooding attacks and network abuses. We extend the framework pro- posed by Zhang and Parashar (2006) to cooperatively detect and react to abnormal behaviors before the tar- get machine collapses and network performance de- grades. In this framework, nodes in an intermediate network share information about their local trac ob- servations, improving their global trac perspective. In our proposal, we add to each node the ability of learning independently, therefore reacting dierently according to its situation in the network and local trac conditions. In particular, this frees the admin- istrator from having to guess and manually set the parameters distinguishing attacks from non-attacks: now such thresholds are learned and set from expe- rience or past data. We expect that our framework provides a faster detection and more accuracy in front of distributed flooding attacks than if static filters or single-machine adaptive mechanisms are used. We show simulations where indeed we observe a high rate of stopped attacks with minimum disturbance to the legitimate users.
更多查看译文
关键词
network performance,data mining,machine learning
AI 理解论文
溯源树
样例
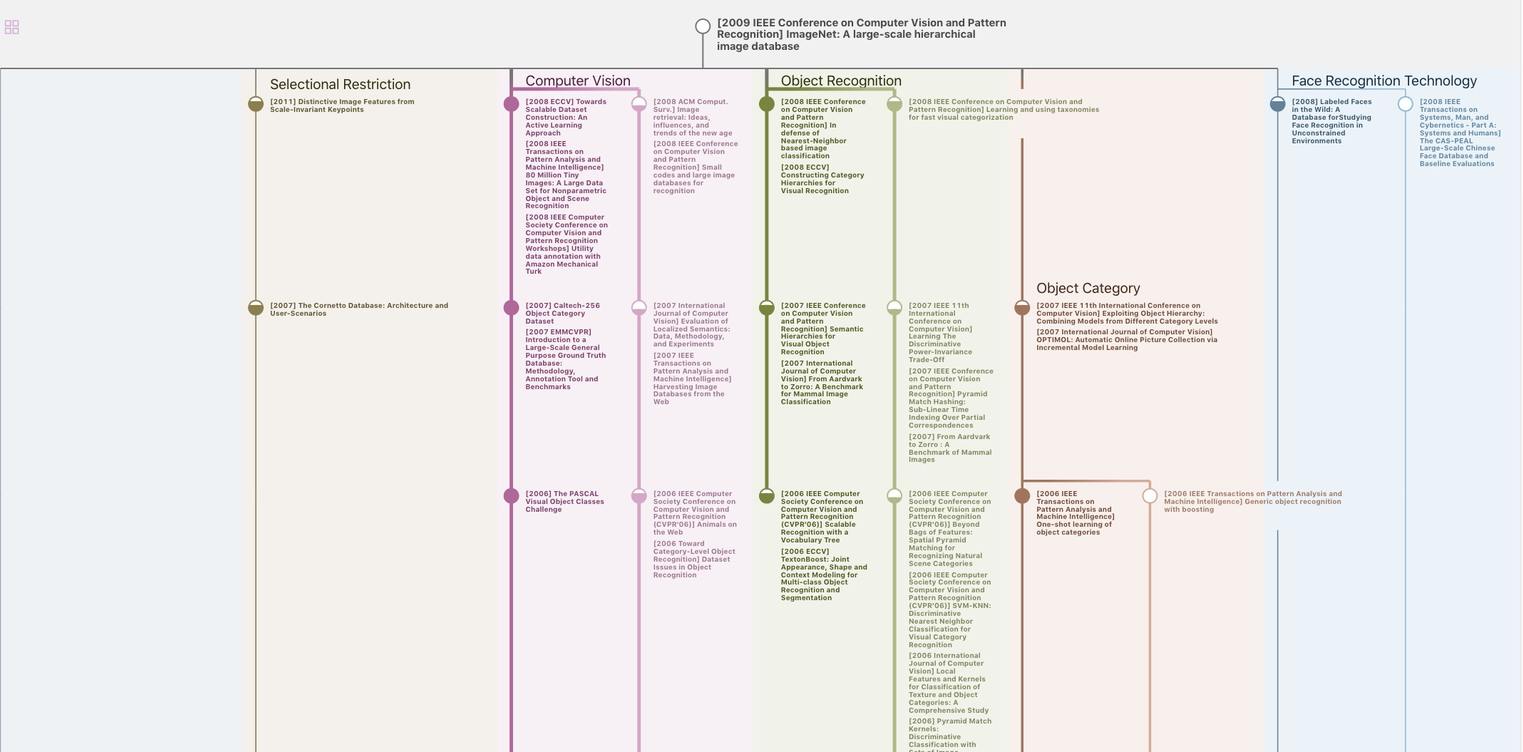
生成溯源树,研究论文发展脉络
Chat Paper
正在生成论文摘要