Predictions for biomedical decision support
Predictions for biomedical decision support(2010)
摘要
Post-discharge medications worked for a general population may not work well for each individual. Similarly, patterns observed from naturally occurring disease outbreaks do not necessarily describe outbreaks of purposeful disease outbreaks (e.g. bioterrorism). Thus, the next frontier in bioanalytical and detection science is developing predictive tools to support the decision system for emergency response. To tackle these challenges, my thesis introduces data-driven paradigms that predict a particular case will have the outcome of interest. My insight is to accommodate individual differences by coherently leveraging information from complementary perspectives (e.g., temporal dependency, relational correlation, feature similarity, and estimation uncertainty) to provide more reliable predictions than possible with existing cohort-based approaches. Specifically, I carefully investigated two representative problems, bioterrorism-related disease outbreak prediction and personalized hospital discharge risk estimation, for which previous research does not provide satisfactory solutions. I developed a data-driven model Temporal Maximum Margin Markov Network to consider the temporal correlation concurrently with relational dependency in bioterrorism-related diseases' outbreaks. This framework reduces the ambiguity in estimating outcome variables from noisy manifestations by considering complementary information. The model is capable of providing state estimation at 69% accuracy one week before the observation, which improved state-of-the-art methods by 12%.Regarding the hospital discharge, I focused on an important but less-studied measurement "calibration," which stratifies how outcomes affect various population groups within a patient-diagnosis population. I designed joint optimization framework to combine discrimination and calibration, and demonstrated models developed under this multi-targeted framework perform better on both metrics than single-targeted models. Concretely, my model AC-LR improved AUCs of existing approaches by 1.3% with calibrated outputs while none of the existing models could.
更多查看译文
关键词
estimation uncertainty,data-driven model,bioterrorism-related disease outbreak prediction,model AC-LR,existing model,disease outbreak,bioterrorism-related disease,biomedical decision support,joint optimization framework,multi-targeted framework,general population
AI 理解论文
溯源树
样例
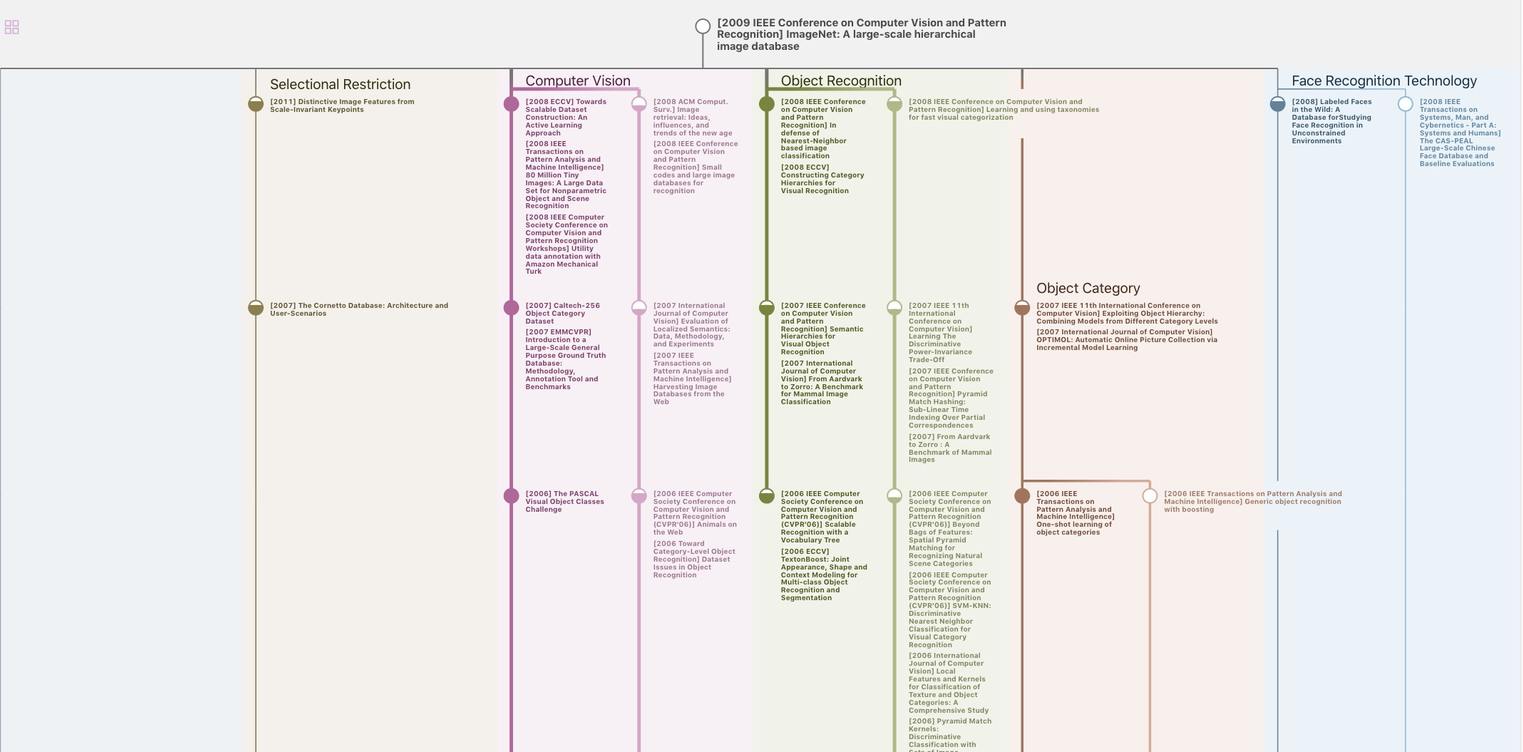
生成溯源树,研究论文发展脉络
Chat Paper
正在生成论文摘要