Kullback-Leibler divergence for nonnegative matrix factorization
ICANN (1)(2011)
摘要
The I-divergence or unnormalized generalization of Kullback-Leibler (KL) divergence is commonly used in Nonnegative Matrix Factorization (NMF). This divergence has the drawback that its gradients with respect to the factorizing matrices depend heavily on the scales of the matrices, and learning the scales in gradient-descent optimization may require many iterations. This is often handled by explicit normalization of one of the matrices, but this step may actually increase the I-divergence and is not included in the NMF monotonicity proof. A simple remedy that we study here is to normalize the input data. Such normalization allows the replacement of the I-divergence with the original KL-divergence for NMF and its variants. We show that using KL-divergence takes the normalization structure into account in a very natural way and brings improvements for nonnegative matrix factorizations: the gradients of the normalized KL-divergence are well-scaled and thus lead to a new projected gradient method for NMF which runs faster or yields better approximation than three other widely used NMF algorithms.
更多查看译文
关键词
nonnegative matrix factorization,input data,gradient-descent optimization,kullback-leibler divergence,original kl-divergence,nmf monotonicity proof,nmf algorithm,normalized kl-divergence,explicit normalization,factorizing matrix,normalization structure
AI 理解论文
溯源树
样例
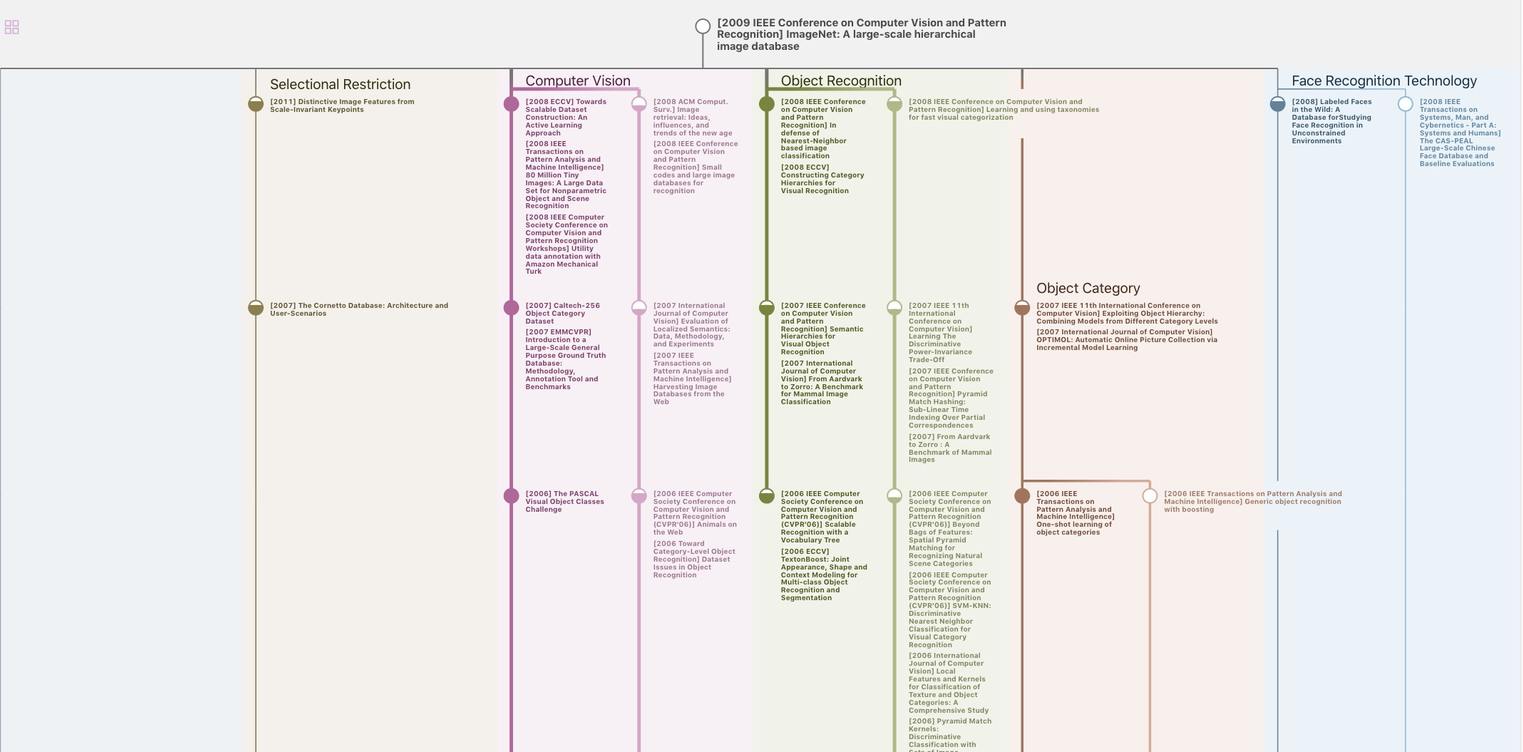
生成溯源树,研究论文发展脉络
Chat Paper
正在生成论文摘要