Unseen Food Creation by Mixing Existing Food Images with Conditional StyleGAN
Proceedings of the 5th International Workshop on Multimedia Assisted Dietary Management(2019)
摘要
In recent years, thanks to the development of generative adversarial networks (GAN), it has become possible to generate food images. However, the quality is still low and it is difficult to generate appetizing and delicious-looking images. In the latest GAN study, StyleGAN enabled high-level feature separation and stochastic variation of generated images by unsupervised learning. However, to manipulate any style, it is necessary to understand the representation of the latent space and to input reference images. In this paper, we propose a conditional version of StyleGAN to control probabilistic fluctuations of disentangled features. The conditional style-based generator can manipulate the style of any domain by providing conditional vectors. By applying the conditional StyleGAN to the food image domain, we successfully have generated higher quality food images than before. In addition, introducing conditional vectors enabled us to control the food categories of generated images and to generate unseen foods by mixing multiple kinds of foods. In the experiment, to show the result of the proposed method explicitly, Food13 dataset is constructed and evaluated by both qualitative evaluation and quantitative evaluation.
更多查看译文
关键词
cnn, food image generation, gan, generative adversarial model
AI 理解论文
溯源树
样例
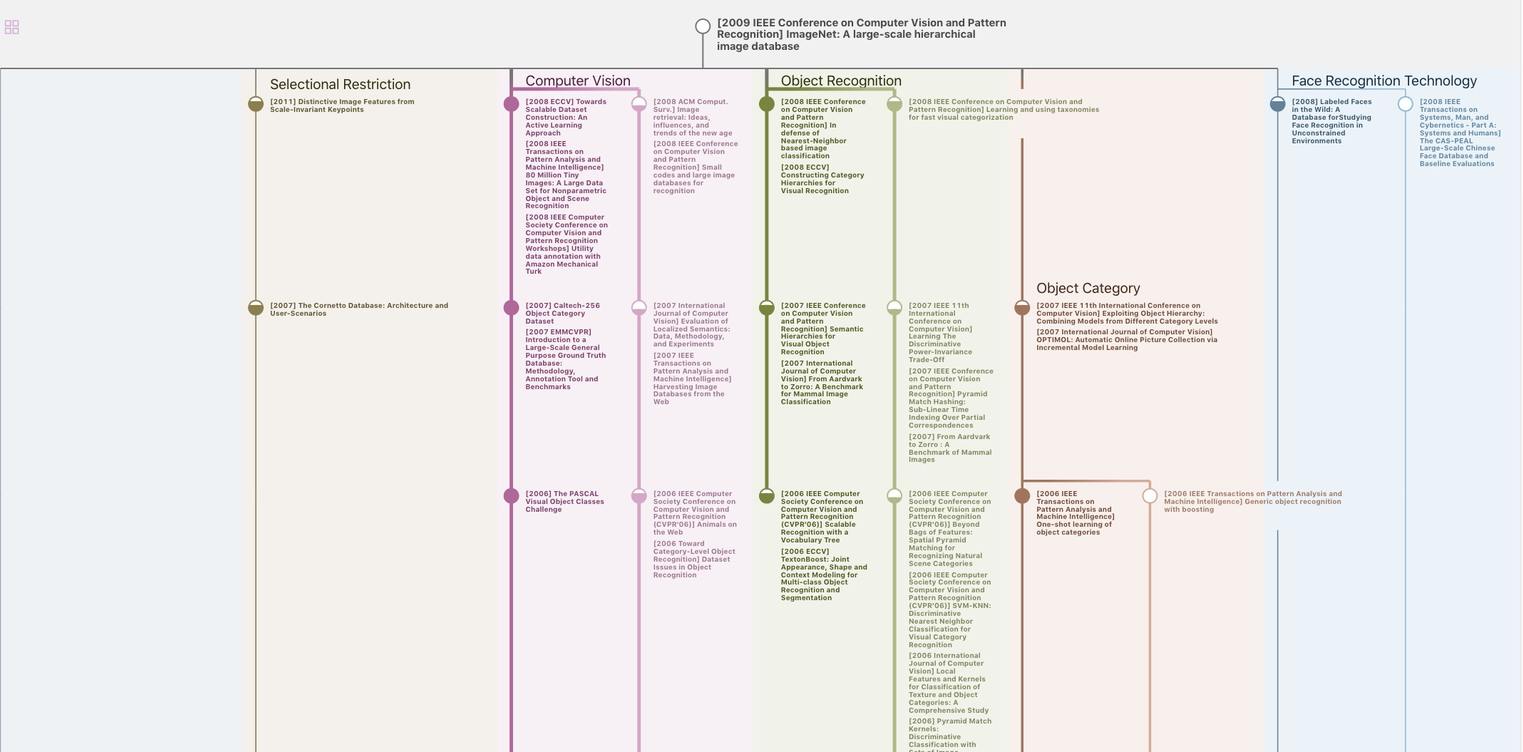
生成溯源树,研究论文发展脉络
Chat Paper
正在生成论文摘要