Importing decision tree rules into Cyc
msra
摘要
We describe a method for automatic discovery of generalizations about data residing in the Cyc Knowledge Base (KB). Data is exported from the Cyc KB into a feature representation, a decision tree classifier is used to discover generalizations in that data, and the learned generalizations are imported back into the KB using probabilistic vocabulary. Our case study is the Whodunit problem: guessing the perpetrator of a terrorism event. We compare two methods of building decision-tree models from which rules can be imported. A single-multiclass model involves a single decision tree with multiple possible values for the predicted variable. A multiple-binary model involves multiple decision trees with binary predicted values, predicting, for a given instance i and class c, whether i has class c. We show that the single multiclass model is more prone to overgeneralization on larger datasets, due to its greater reliance on the closed world assumption. We conclude that for the purpose of rule extraction into a large knowledge base, it is better to use multiple binary decision trees.
更多查看译文
AI 理解论文
溯源树
样例
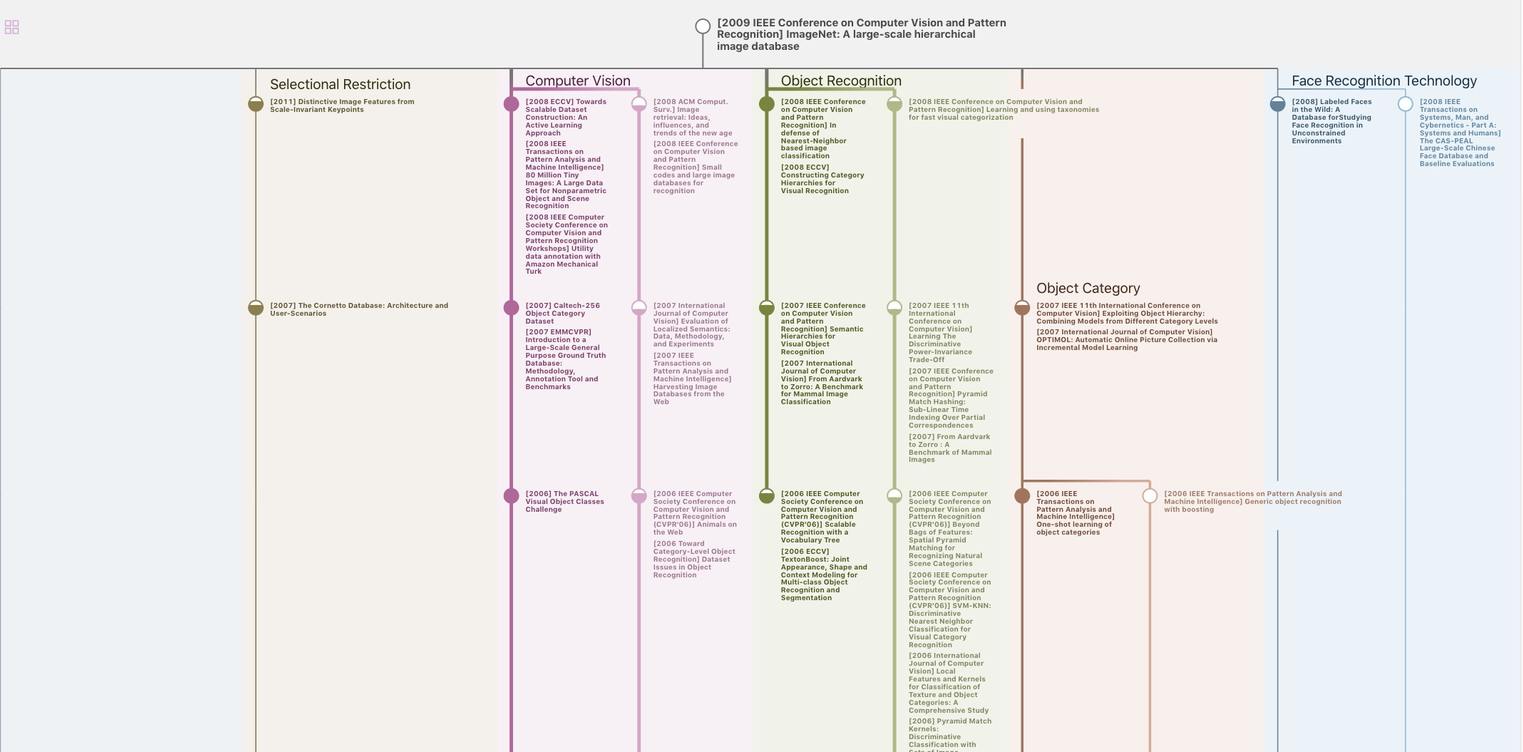
生成溯源树,研究论文发展脉络
Chat Paper
正在生成论文摘要