Mixture deconvolution and analysis of Ames mutagenicity data
Chemometrics and Intelligent Laboratory Systems(2002)
摘要
Mixtures abound in chemistry: two or more compounds may be present in the same sample, the same biological effect may be produced by two different mechanisms, or two compounds might bind to a receptor in different orientations or even in different places. Sometimes, results are given in summary form. For example, a chemical may be declared a mutagen due to any of several assay results from an Ames test. Clearly, a single mathematical model is not going to hold for data sets where such multiplicity of phenomena are represented. We need molecular descriptors and statistical methods which enable us to deconvolute such mixtures. Our idea is to combine topological chemical descriptors—augmented atoms and through-bond distance measures—with a statistical technique, segmentation recursive partitioning, that is capable of dealing with mixtures. The benefit is the ability to develop structure–activity relationships for large, heterogeneous data sets. We successfully demonstrate the effectiveness of the above descriptors and the technique of recursive partitioning with Ames test results taken from public sources.
更多查看译文
关键词
Mutagenicity,Ames test,Recursive partitioning,Mixtures,Segmentation,QSAR
AI 理解论文
溯源树
样例
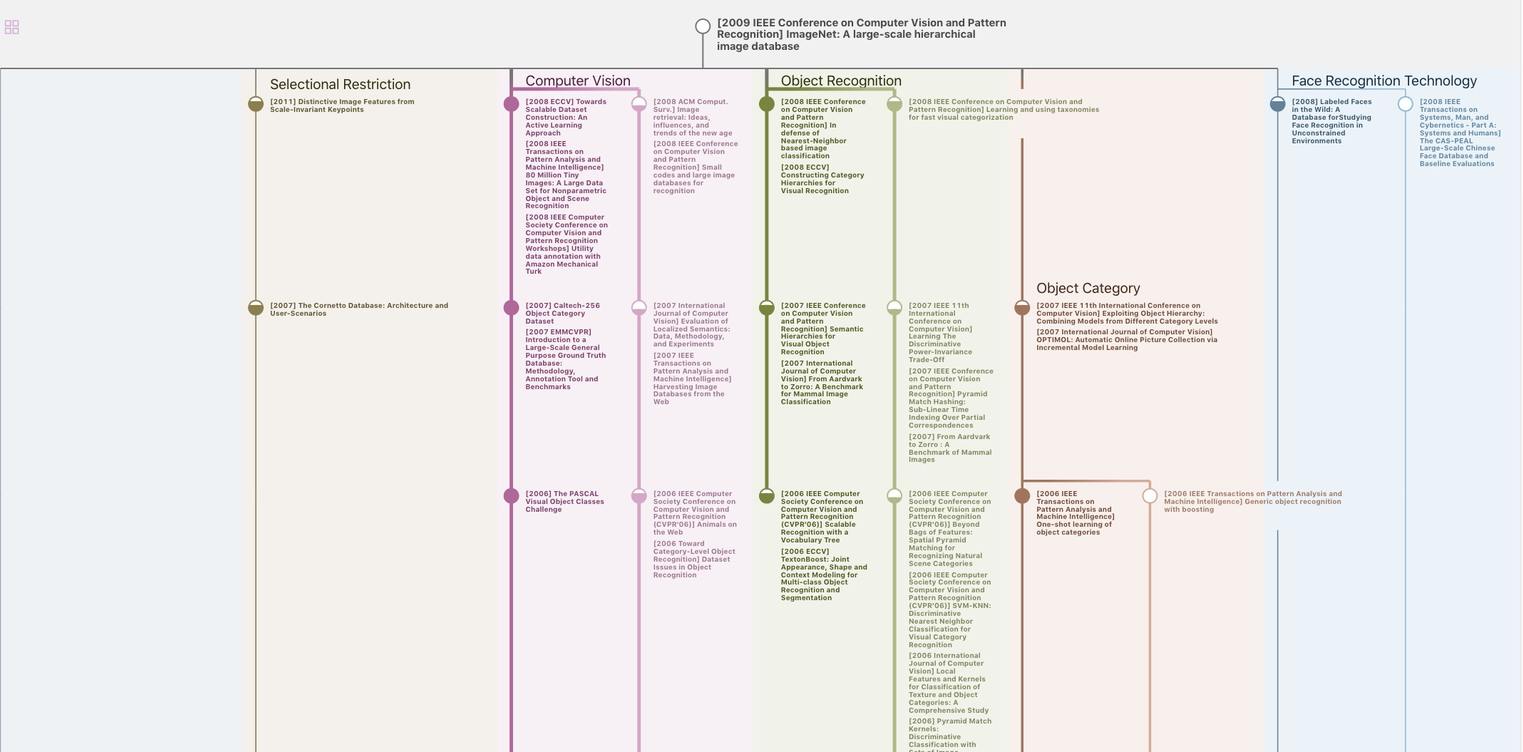
生成溯源树,研究论文发展脉络
Chat Paper
正在生成论文摘要