Detecting epidemics using highly noisy data.
MOBIHOC(2013)
摘要
ABSTRACTFrom Cholera, AIDS/HIV, and Malaria, to rumors and viral video, understanding the causative network behind an epidemic's spread has repeatedly proven critical for managing the spread (controlling or encouraging, as the case may be). Our current approaches to understand and predict epidemics rely on the scarce, but exact/reliable, expert diagnoses. This paper proposes a different way forward: use more readily available but also more noisy data with {\em many false negatives and false positives}, to determine the causative network of an epidemic. Specifically, we consider an epidemic that spreads according to one of two networks. At some point in time we see a small random subsample (perhaps a vanishingly small fraction) of those infected, along with an order-wise similar number of false positives. We derive sufficient conditions for this problem to be detectable, and provide an efficient algorithm that solves the hypothesis testing problem. We apply this model to two settings. In the first setting, we simply want to distinguish between random illness (a complete graph) and an epidemic (spread along a structured graph). In the second, we have a superposition of both of these, and we wish to detect which is the strongest component.
更多查看译文
AI 理解论文
溯源树
样例
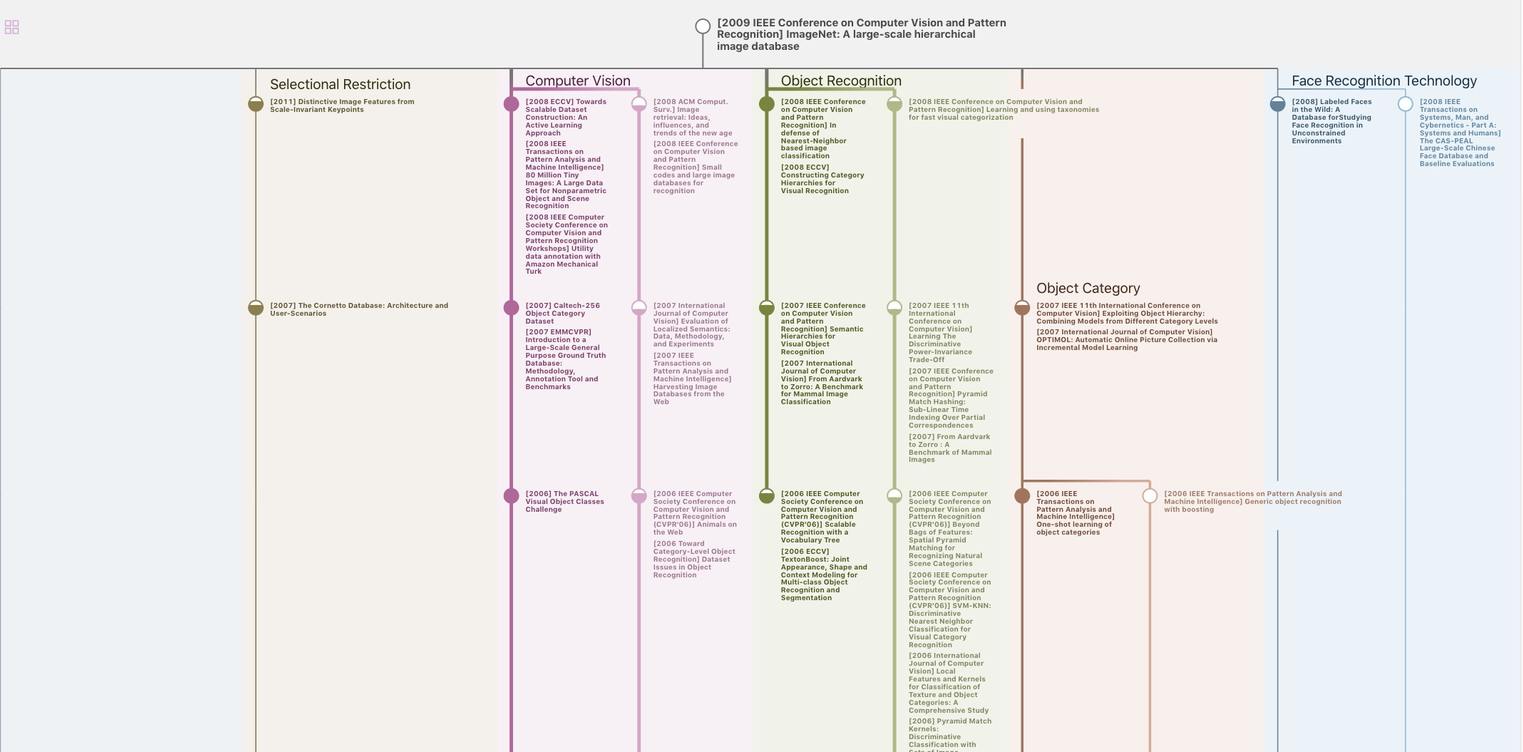
生成溯源树,研究论文发展脉络
Chat Paper
正在生成论文摘要