Semiautomatic Benchmarking of Feature Vectors for Multimedia Retrieval
msra(2007)
摘要
Modern Digital Library applications store and process mas- sive amounts of information. Usually, this data is not limited to raw textual or numeric data { typical applications also deal with multimedia data such as images, audio, video, or 3D geometric models. For providing eective retrieval functionality, appropriate meta data descriptors that allow calculation of similarity scores between data instances are requires. Feature vectors are a generic way for describing multimedia data by vectors formed from numerically cap- tured object features. They are used in similarity search, but also, can be used for clustering and wider multimedia analysis applications. Extracting eective feature vectors for a given data type is a challenging task. Determining good feature vector extrac- tors usually involves experimentation and application of su- pervised information. However, such experimentation usu- ally is expensive, and supervised information often is data dependent. We address the feature selection problem by a novel approach based on analysis of certain feature space images. We develop two image-based analysis techniques for the automatic discrimination power analysis of feature spaces. We evaluate the techniques on a comprehensive fea- ture selection benchmark, demonstrating the eectiveness of our analysis and its potential toward automatically address- ing the feature selection problem.
更多查看译文
关键词
s,feature vectors,self organizing maps,visual analytics
AI 理解论文
溯源树
样例
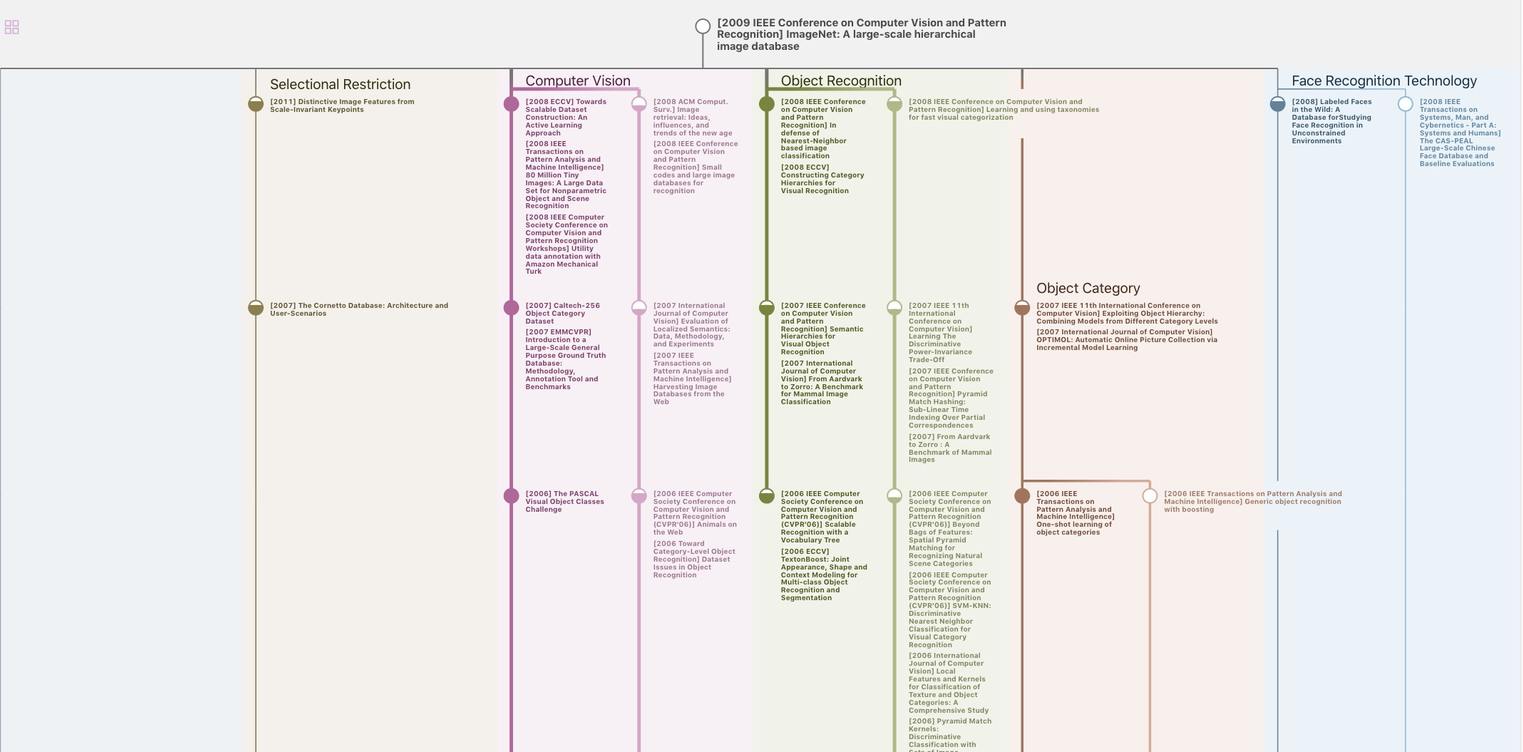
生成溯源树,研究论文发展脉络
Chat Paper
正在生成论文摘要