Learning visual flows: A Lie algebraic approach
CVPR(2009)
摘要
We present a novel method for modeling dynamic visual phenomena, which consists of two key aspects. First, the integral motion of constituent elements in a dynamic scene is captured by a common underlying geometric transform process. Second, a Lie algebraic representation of the transform process is introduced, which maps the transformation group to a vector space, and thus overcomes the difficulties due to the group structure. Consequently, the statistical learning techniques based on vector spaces can be readily applied. Moreover, we discuss the intrinsic connections between the Lie algebra and the Linear dynamical processes, showing that our model induces spatially varying fields that can be estimated from local motions without continuous tracking. Following this, we further develop a statistical framework to robustly learn the flow models from noisy and partially corrupted observations. The proposed methodology is demonstrated on real world phenomenon, inferring common motion patterns from surveillance videos of crowded scenes and satellite data of weather evolution.
更多查看译文
关键词
group structure,dynamic visual phenomena,integral motion,vector space,transformation group,statistical analysis,dynamic scene,linear dynamical process,lie algebraic approach,visual flows learning,lie algebras,geometric transform process,computer vision,lie algebraic representation,statistical learning,geometry,vectors,mathematical model,trajectory,tracking,algebra,lie algebra,layout,robustness,dynamics,motion estimation,satellites
AI 理解论文
溯源树
样例
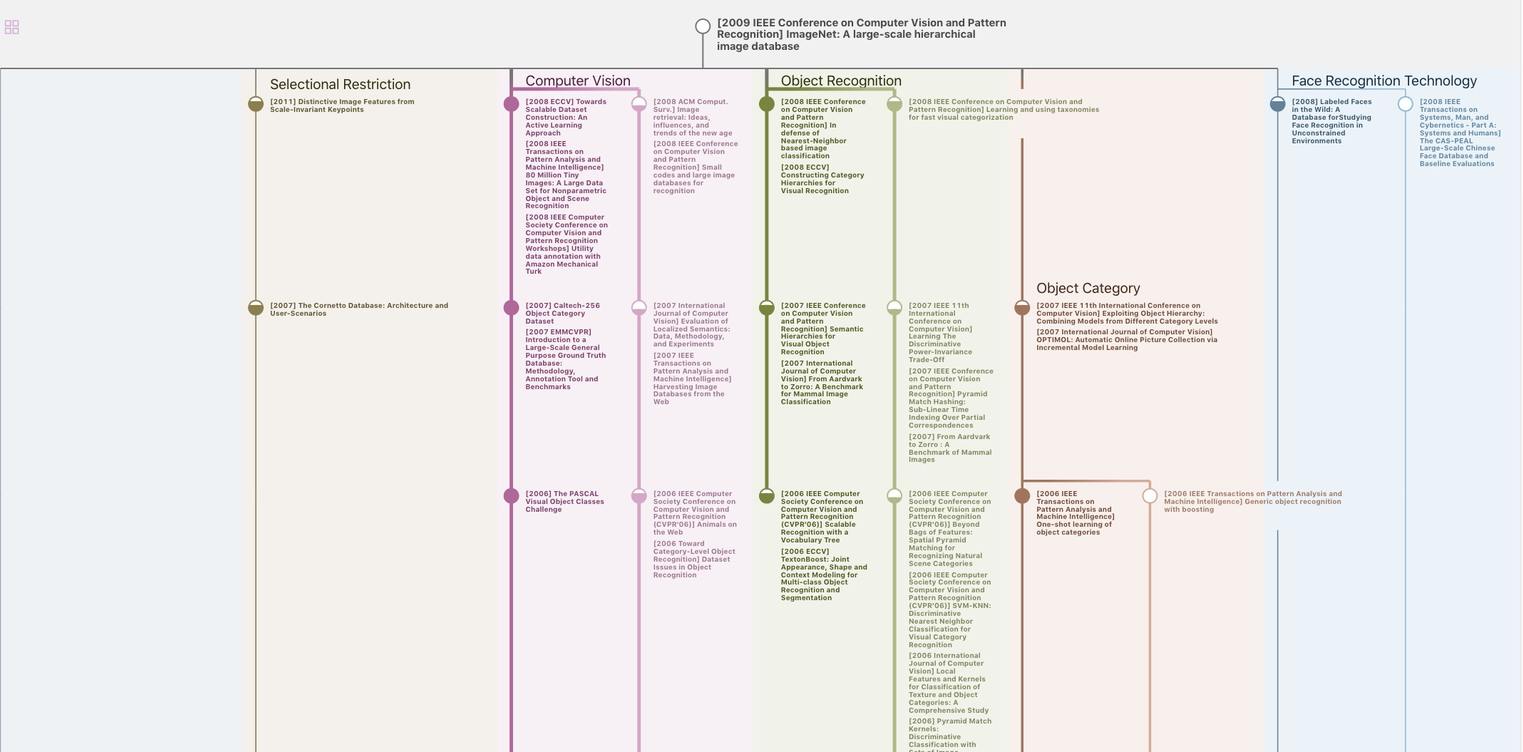
生成溯源树,研究论文发展脉络
Chat Paper
正在生成论文摘要