Detecting Gene Regulation Relations from Microarray Time Series Data
MLMTA(2006)
摘要
Microarrays are important tools in the quest to map the gene regulation networks of cells. A common use of microarrays result in time series pairs that indicates how the output of one gene affects another. Substantial efforts have been made towards identifying pairs of microarray time series that indicate that one gene is a regulator for another. However, most approaches make assumptions about the behavior of the regulation relation time series pairs and then attempt to identify microarray time series pairs with similar profiles. Such approaches are only partially successful and frequently cannot identity microarray times series pairs that are known to be regulation pairs. In this work, we present a new machine learning approach utilizing a set of Hidden Markov Model, HMMs, for scoring temporal relationships between gene expressions that uses a training set of known regulation relation that avoids the need to assume a specific regulation profile. response to an increase of its activator's expression level, and decreases in response to its activator's expression level decreases. The repressor has an opposite effect: target gene's expression level decreases in response to the increase of expression level for its repressor, and increases in response to the decreases of its repressors expression level. In most cases, regulation relations are relationships that are switched on and off by different types of chemical and biological signals. We refer to the behavior of the pair when the relationship is switched on as a temporal episode profile or just a temporal profile.
更多查看译文
关键词
datamining,gene regulatory networks,machine learning,bioinformatics,hidden markov model.,time series data,gene regulation,time series,gene regulatory network,gene expression,hidden markov model
AI 理解论文
溯源树
样例
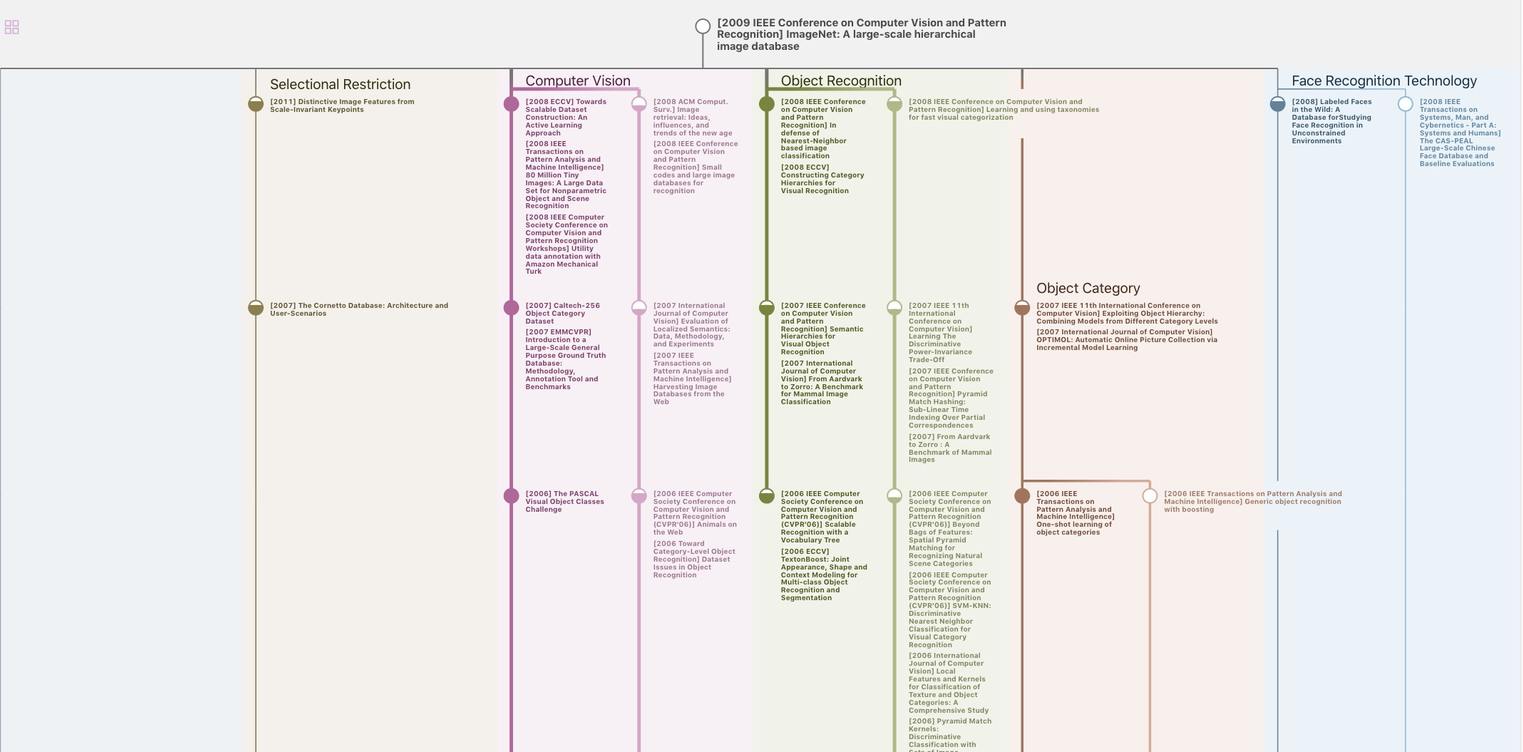
生成溯源树,研究论文发展脉络
Chat Paper
正在生成论文摘要