Modeling, searching, and explaining abnormal instances in multi-relational networks
Modeling, searching, and explaining abnormal instances in multi-relational networks(2006)
摘要
An important research problem in knowledge discovery and data mining is to identify abnormal instances. Finding anomalies in data has important applications in domains such as fraud detection and homeland security. While there are several existing methods to identify anomalies in numerical datasets, there has been little work aimed at discovering abnormal instances in large and complex relational networks whose nodes are richly connected with many different types of links. To address this problem we designed a novel, unsupervised, domain independent framework that utilizes the information provided by different types of links to identify abnormal nodes. Our approach measures the dependencies between nodes and paths in the network to capture what we call "semantic profiles" of nodes, and then applies a distance-based outlier detection method to find abnormal nodes that are significantly different from their closest neighbors. In a set of experiments on synthetic data about organized crime, our system can almost perfectly identify the hidden crime perpetrators and outperforms several other state-of-the-art methods that have been used to analyze the 9/11 terrorist network by a significant margin. To facilitate validation, we designed a novel explanation mechanism that can generate meaningful and human-understandable explanations for abnormal nodes discovered by our system. Such explanations not only facilitate the verification and screening out of false positives, but also provide directions for further investigation. The explanation system uses a classification-based approach to summarize the characteristic features of a node together with a path-to-sentence generator to describe these features in natural language. In an experiment with human subjects we show that the explanation system allows them to identify hidden perpetrators in a complex crime dataset much more accurately and efficiently. We also demonstrate the generality and domain independence of our system by applying it to find abnormal and interesting instances in two representative natural datasets in the movie and bibliography domain. Finally, we discuss our solutions to several related applications including abnormal path discovery, local node discovery, automatic node description and explanation-based outlier detection.
更多查看译文
关键词
abnormal node,abnormal instance,explanation system,different type,abnormal path discovery,automatic node description,bibliography domain,complex crime dataset,data mining,distance-based outlier detection method,multi-relational network
AI 理解论文
溯源树
样例
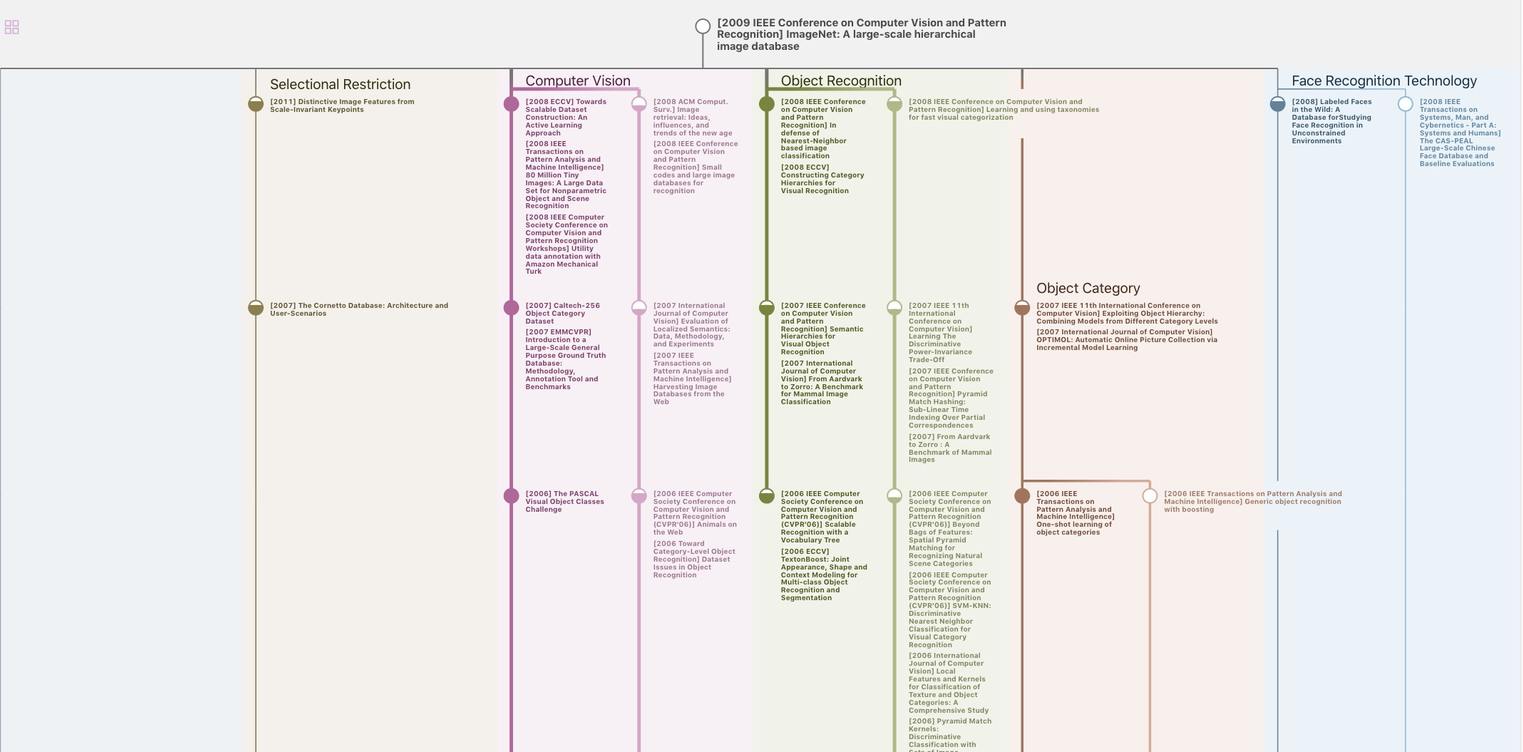
生成溯源树,研究论文发展脉络
Chat Paper
正在生成论文摘要