Sentiment Analysis On Evolving Social Streams: How Self-Report Imbalances Can Help
WSDM(2014)
摘要
Real-time sentiment analysis is a challenging machine learning task, due to scarcity of labeled data and sudden changes in sentiment caused by real-world events that need to be instantly interpreted. In this paper we propose solutions to acquire labels and cope with concept drift in this setting, by using findings from social psychology on how humans prefer to disclose some types of emotions. In particular, we use findings that humans are more motivated to report positive feelings rather than negative feelings and also prefer to report extreme feelings rather than average feelings.We map each of these self-report imbalances on two machine learning sub-tasks. The preference on the disclosure of positive feelings can be explored to generate labeled data on polarizing topics, where a positive event for one group usually induces negative feelings from the opposing group, generating an imbalance on user activity that unveils the current dominant sentiment.Based on the knowledge that extreme experiences are more reported than average experiences, we propose a feature representation strategy that focus on terms which appear at spikes in the social stream. When comparing to a static text representation (TF-IDF), we found that our feature representation is more capable of detecting new informative features that capture the sudden changes on sentiment stream caused by real-world events.We show that our social psychology-inspired framework produces accuracies up to 84% while analyzing live reactions in the debate of two popular sports on Twitter - soccer and football - despite requiring no human effort in generating supervisory labels.
更多查看译文
关键词
Sentiment Analysis,Stream Data Mining,Social Media Analytics
AI 理解论文
溯源树
样例
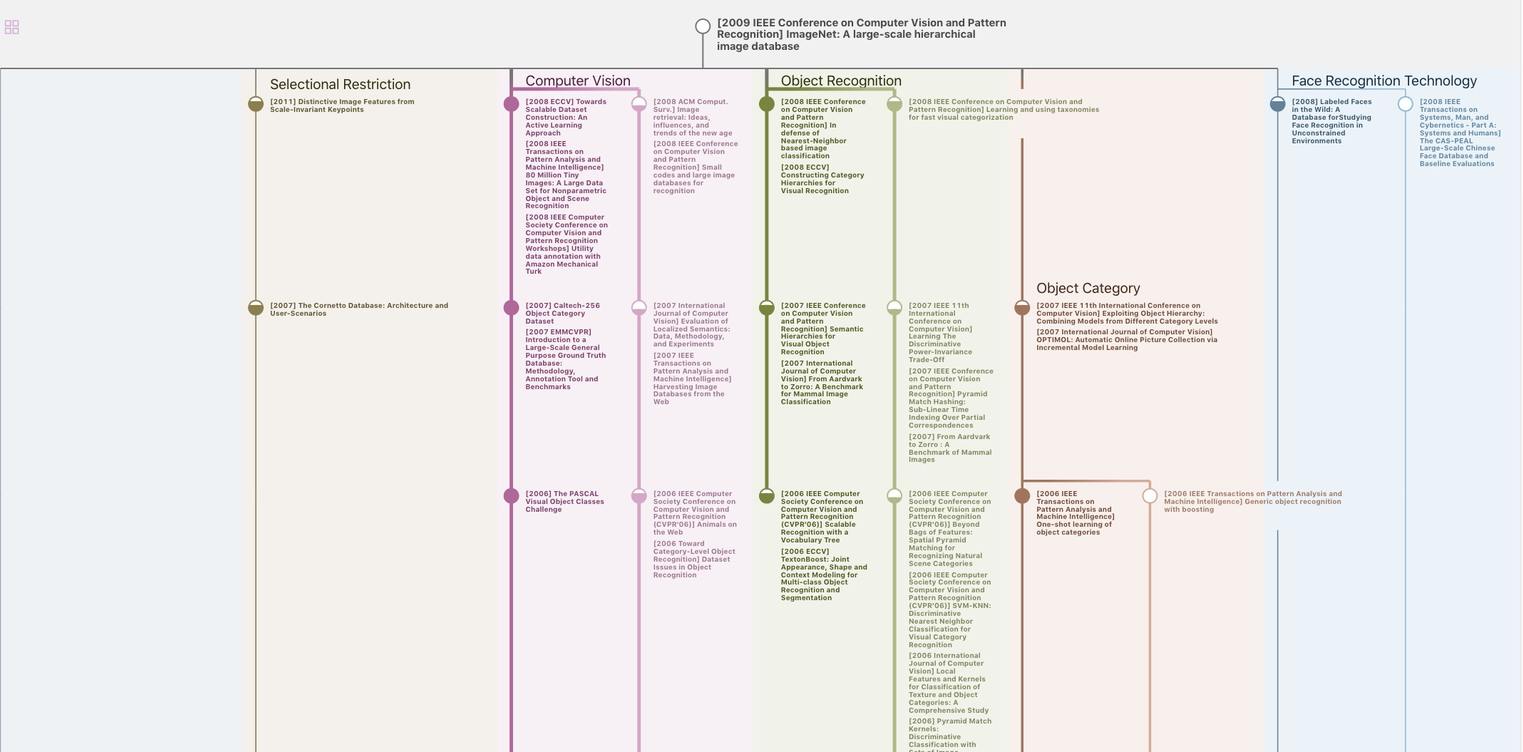
生成溯源树,研究论文发展脉络
Chat Paper
正在生成论文摘要