Monitoring for disease progression via mathematical time-series modeling: Actigraphy-based monitoring patients with depressive disorder
CCNC(2013)
摘要
Home-based monitoring of the changes on patients' state using non-invasive sensing device such as actigraph is important in the aspect of treatment, management and prevention of many chronic diseases. Although the characteristics of the internal structures (i.e., the fluctuation or the trend) inherent in the actigraphic signal can significantly represent the disease state, there has been no method to extract and analyze such information. In this paper, we have proposed a novel approach to determine the disease state based on features extracted from the structural information of patient's actigraphic data by utilizing a mathematical time-series modeling method, autoregressive (AR)-generalized conditional heteroskedascity (GARCH) models. Our approach consists of three steps: finding structural breaks as the disease state transitions, building a time-series model for each local segment, and analyzing the features from each model to classify the severity of the disease. We have applied the proposed method to the actigraphic data of the patients with depressive disorder, and the experimental results showed that features extracted by our modeling method have played an important role to discriminate disease severities.
更多查看译文
关键词
disease state transitions,disease progression monitoring,patient actigraphic data,depression,medical disorders,diseases,actigraphic signal,actigraphy-based patient monitoring,chronic disease prevention,disease state representation,mathematical time-series modeling method,disease monitoring,patient monitoring,disease state determination,time series modeling,home-based monitoring,structural information,structural break,autoregressive processes,chronic disease management,ar-garch models,feature extraction,structural breaks,depressive disorder,disease severity classification,noninvasive sensing device,actigraphy,autoregressive-generalized conditional heteroskedascity model,time series,patient treatment,ar-garch
AI 理解论文
溯源树
样例
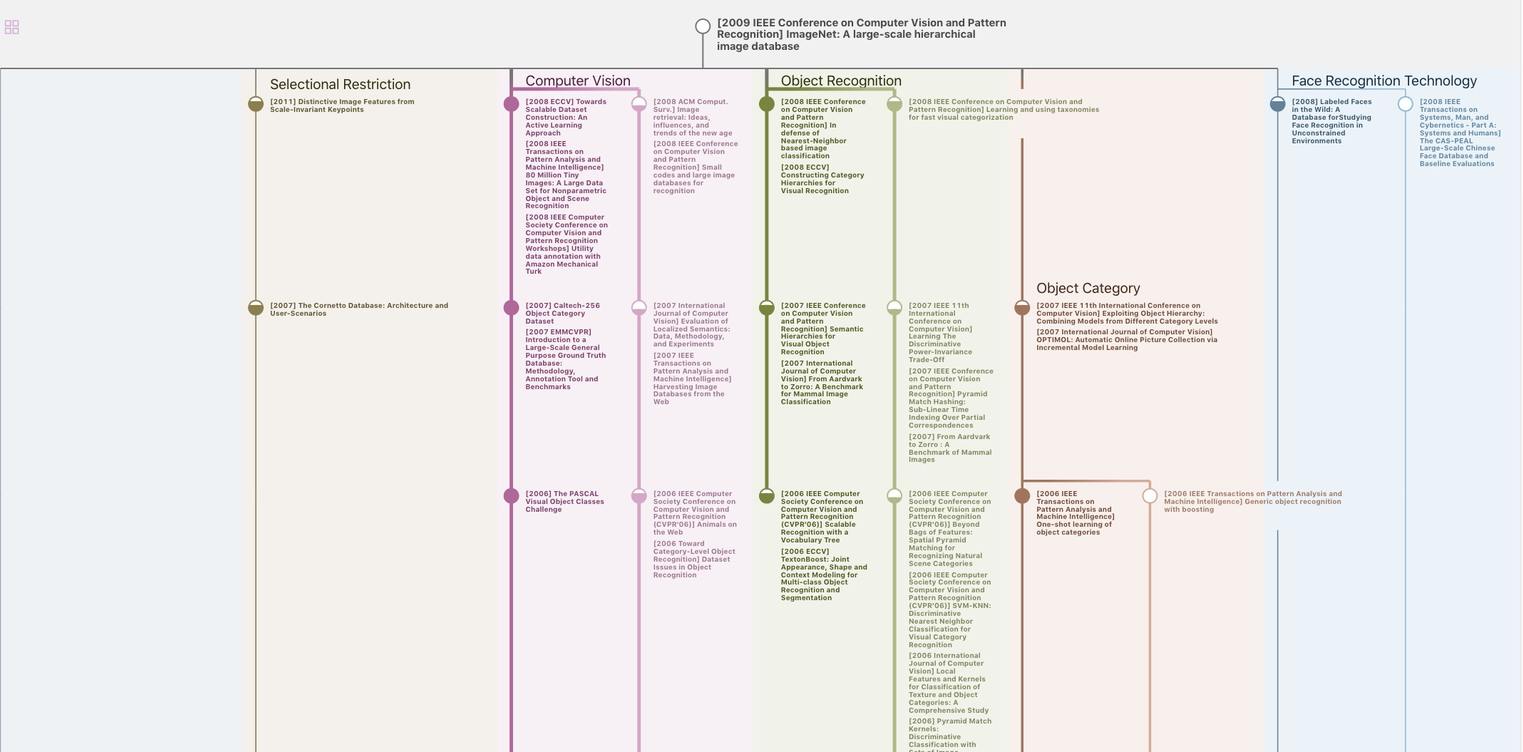
生成溯源树,研究论文发展脉络
Chat Paper
正在生成论文摘要