Massively Parallel Model of Extended Memory Use in Evolutionary Game Dynamics
IPDPS(2013)
摘要
To study the emergence of cooperative behavior, we have developed a scalable parallel framework for evolutionary game dynamics. This is a critical computational tool enabling large-scale agent simulation research. An important aspect is the amount of history, or memory steps, that each agent can keep. When six memory steps are taken into account, the strategy space spans 24096 potential strategies, requiring large populations of agents. We introduce a multi-level decomposition method that allows us to exploit both multi-node and thread-level parallel scaling while minimizing communication overhead. We present the results of a production run modeling up to six memory steps for populations consisting of up to 10^18 agents, making this study one of the largest yet undertaken. The high rate of mutation within the population results in a non-trivial parallel implementation. The strong and weak scaling studies provide insight into parallel scalability and programmability trade-offs for large-scale simulations, while exhibiting near perfect weak and strong scaling on 16,384 tasks on Blue Gene/Q. We further show 99% weak scaling up to 294,912 processors 82% strong scaling efficiency up to 262,144 processors of Blue Gene/P. Our framework marks an important step in the study of game dynamics with potential applications in fields ranging from biology to economics and sociology.
更多查看译文
关键词
non-trivial parallel implementation,parallel model,blue gene,memory step,strong scaling,scalable parallel framework,thread-level parallel scaling,weak scaling study,extended memory use,evolutionary game dynamic,evolutionary game dynamics,strong scaling efficiency,parallel scalability,computational modeling,games,biology,economics,history,thin film transistors,statistics,object oriented programming,parallel programming,sociology,evolutionary dynamics,game theory,evolutionary computation,extended memory
AI 理解论文
溯源树
样例
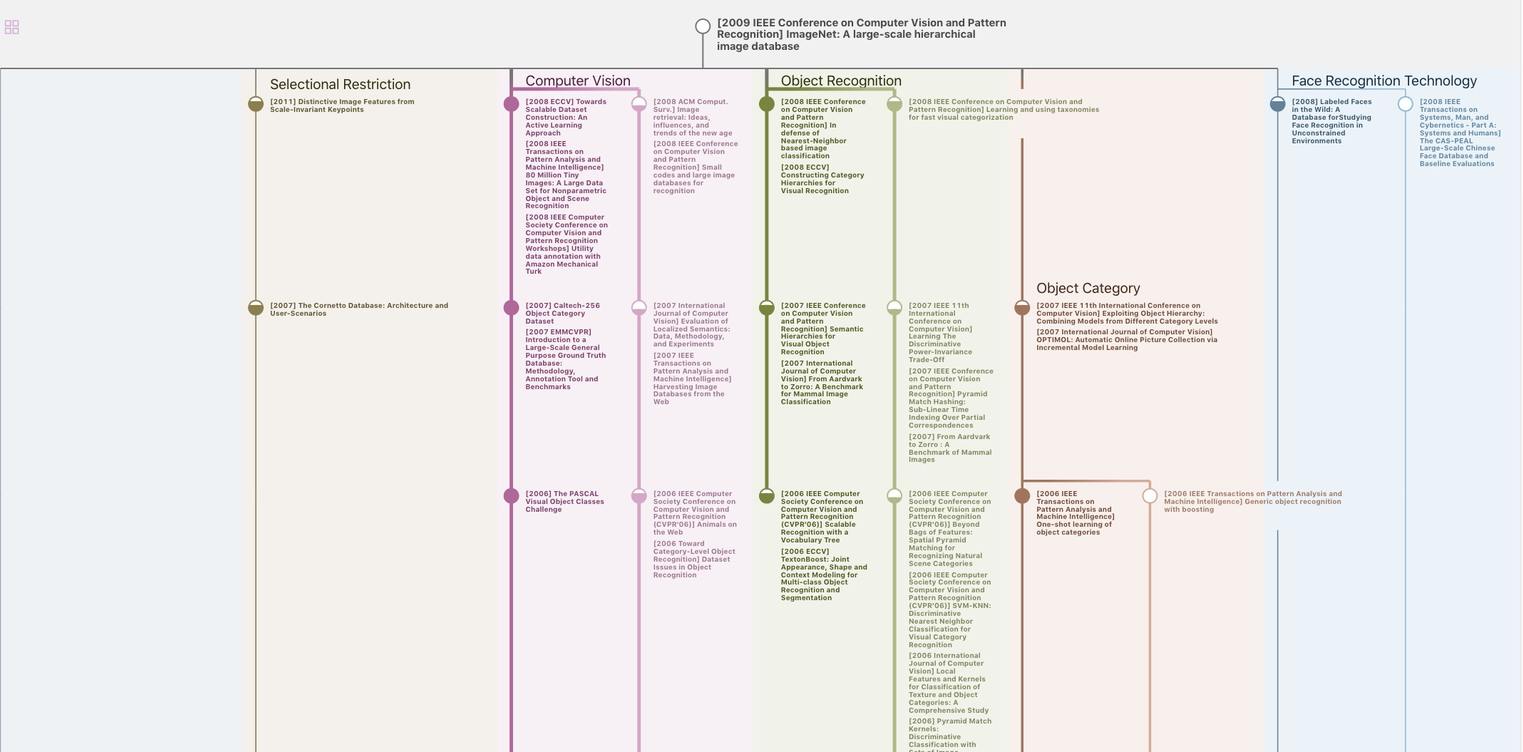
生成溯源树,研究论文发展脉络
Chat Paper
正在生成论文摘要