Optimal gene subset selection using the modified SFFS algorithm for tumor classification
Neural Computing and Applications(2012)
摘要
A reliable and precise classification of tumors is essential for successful treatment of cancer. Gene selection is an important step for improved diagnostics. The modified SFFS (sequential forward floating selection) algorithm based on weighted Mahalanobis distance, called MSWM, is proposed to identify optimal informative gene subsets taking into account joint discriminatory power for accurate discrimination in this study. Firstly, we make use of the one-dimensional weighted Mahalanobis distance to perform a preliminary selection of genes and then make use of the modified SFFS method and multidimensional weighted Mahalanobis distance to obtain the optimal informative gene subset for tumor classification. Finally, we used the k nearest neighbor and naive Bayes methods to classify tumors based on the optimal gene subset selected using the MSWM method. To validate the efficiency, the proposed MSWM method is applied to classify two different DNA microarray datasets. Our empirical study shows that the MSWM method for tumor classification can obtain better effectiveness of classification than the BWR (the ratio of between-groups to within-groups sum of squares) and IVGA_I (independent variable group analysis I) methods. It suggests that the MSWM gene selection method is ability to obtain correct informative gene subsets taking into account genes’ joint discriminatory power for tumor classification.
更多查看译文
关键词
Optimal gene subset selection,Modified SFFS,Weighted Mahalanobis distance,Joint discriminatory power,Tumor classification
AI 理解论文
溯源树
样例
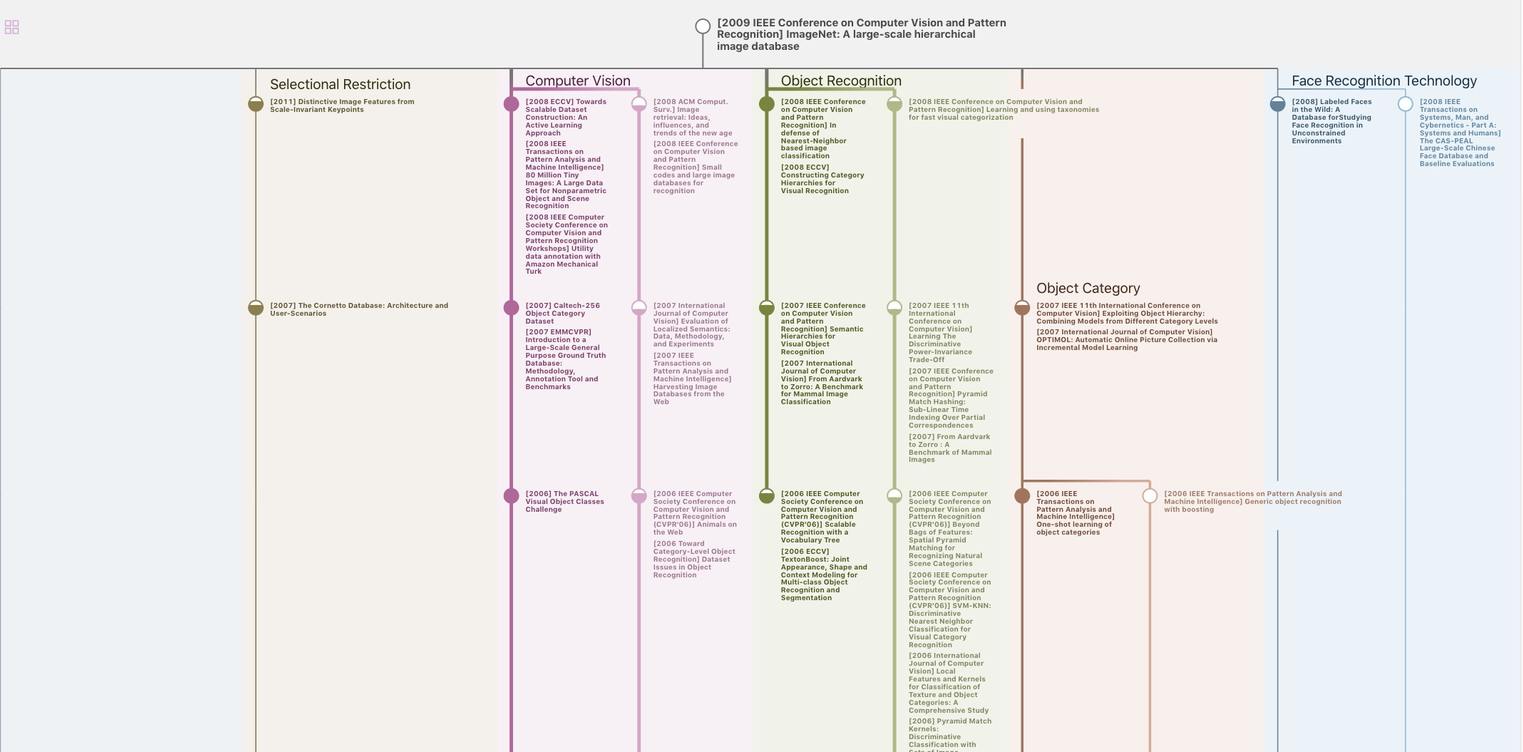
生成溯源树,研究论文发展脉络
Chat Paper
正在生成论文摘要