Gaussian Kullback-Leibler approximate inference
Journal of Machine Learning Research(2013)
摘要
We investigate Gaussian Kullback-Leibler (G-KL) variational approximate inference techniques for Bayesian generalised linear models and various extensions. In particular we make the following novel contributions: sufficient conditions for which the G-KL objective is differentiable and convex are described; constrained parameterisations of Gaussian covariance that make G-KL methods fast and scalable are provided; the lower bound to the normalisation constant provided by G-KL methods is proven to dominate those provided by local lower bounding methods; complexity and model applicability issues of G-KL versus other Gaussian approximate inference methods are discussed. Numerical results comparing G-KL and other deterministic Gaussian approximate inference methods are presented for: robust Gaussian process regression models with either Student-t or Laplace likelihoods, large scale Bayesian binary logistic regression models, and Bayesian sparse linear models for sequential experimental design.
更多查看译文
关键词
g-kl method,linear model,gaussian kullback-leibler,gaussian kullback-leibler approximate inference,deterministic gaussian approximate inference,variational approximate inference technique,gaussian covariance,bayesian sparse linear model,g-kl objective,gaussian approximate inference method,robust gaussian process regression,gaussian processes,experimental design,active learning
AI 理解论文
溯源树
样例
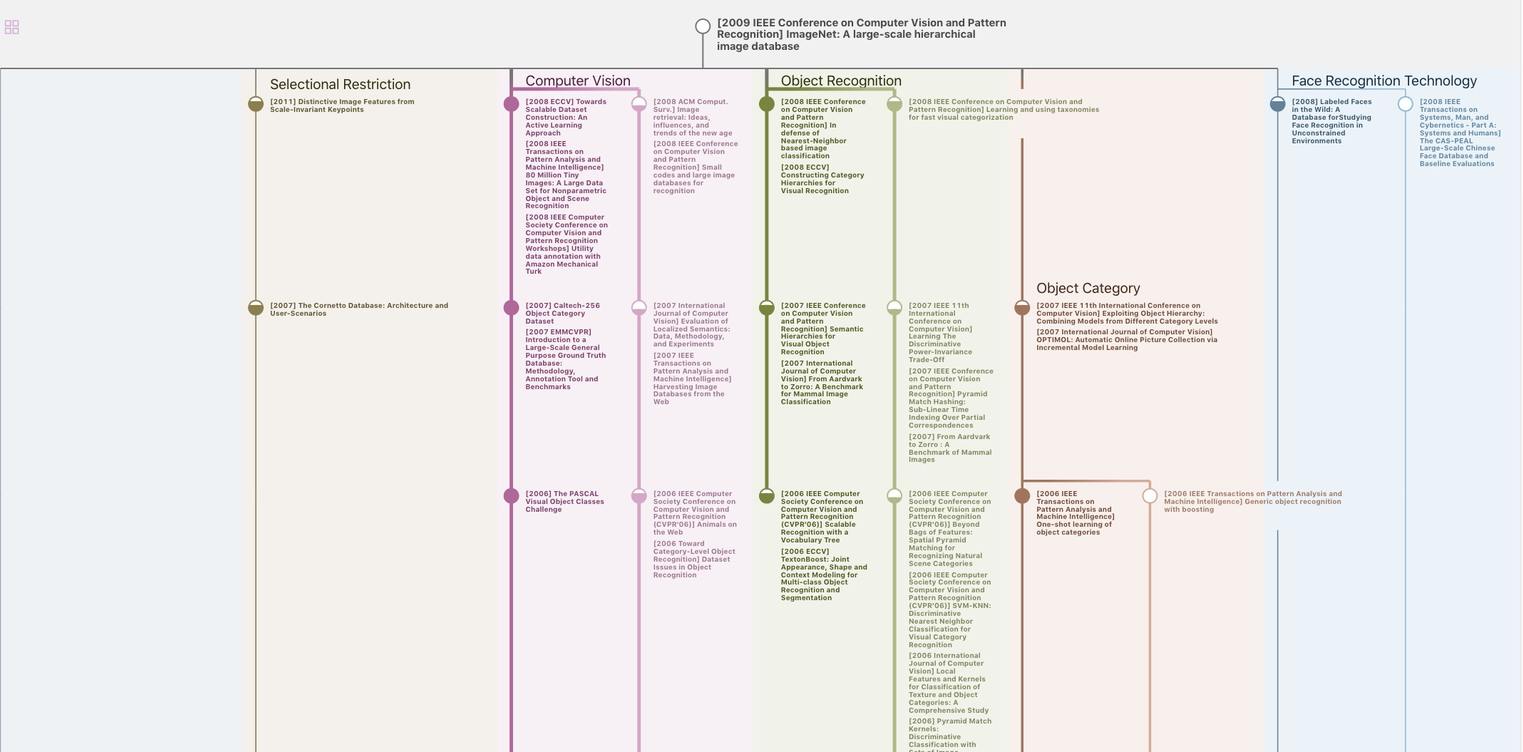
生成溯源树,研究论文发展脉络
Chat Paper
正在生成论文摘要