A self-organizing multi-memory system for autonomous agents
IJCNN(2012)
摘要
This paper presents a self-organizing approach to the learning of procedural and declarative knowledge in parallel using independent but interconnected memory models. The proposed system, employing fusion Adaptive Resonance Theory (fusion ART) network as a building block, consists of a declarative memory module, that learns both episodic traces and semantic knowledge in real time, as well as a procedural memory module that learns reactive responses to its environment through reinforcement learning. More importantly, the proposed multi-memory system demonstrates how the various memory modules transfer knowledge and cooperate with each other for a higher overall performance. We present experimental studies, wherein the proposed system is tasked to learn the procedural and declarative knowledge for an autonomous agent playing in a first person game environment called Unreal Tournament. Our experimental results show that the multi-memory system is able to enhance the performance of the agent in a real time environment by utilizing both its procedural and declarative knowledge.
更多查看译文
关键词
semantic knowledge,procedural memory module,art,interconnected memory models,reinforcement learning,reactive response learning,fusion art network,fusion adaptive resonance theory network,autonomous agents,semantic memory,learning (artificial intelligence),unreal tournament,episodic memory,art neural nets,declarative knowledge learning,multi-robot systems,independent memory models,declarative memory module,agent,self-organizing,knowledge transfer,multi-agent systems,self-organizing multimemory system,procedural knowledge learning,self-organising feature maps,procedural memory,computational modeling,vectors,semantics,multi agent systems,learning artificial intelligence
AI 理解论文
溯源树
样例
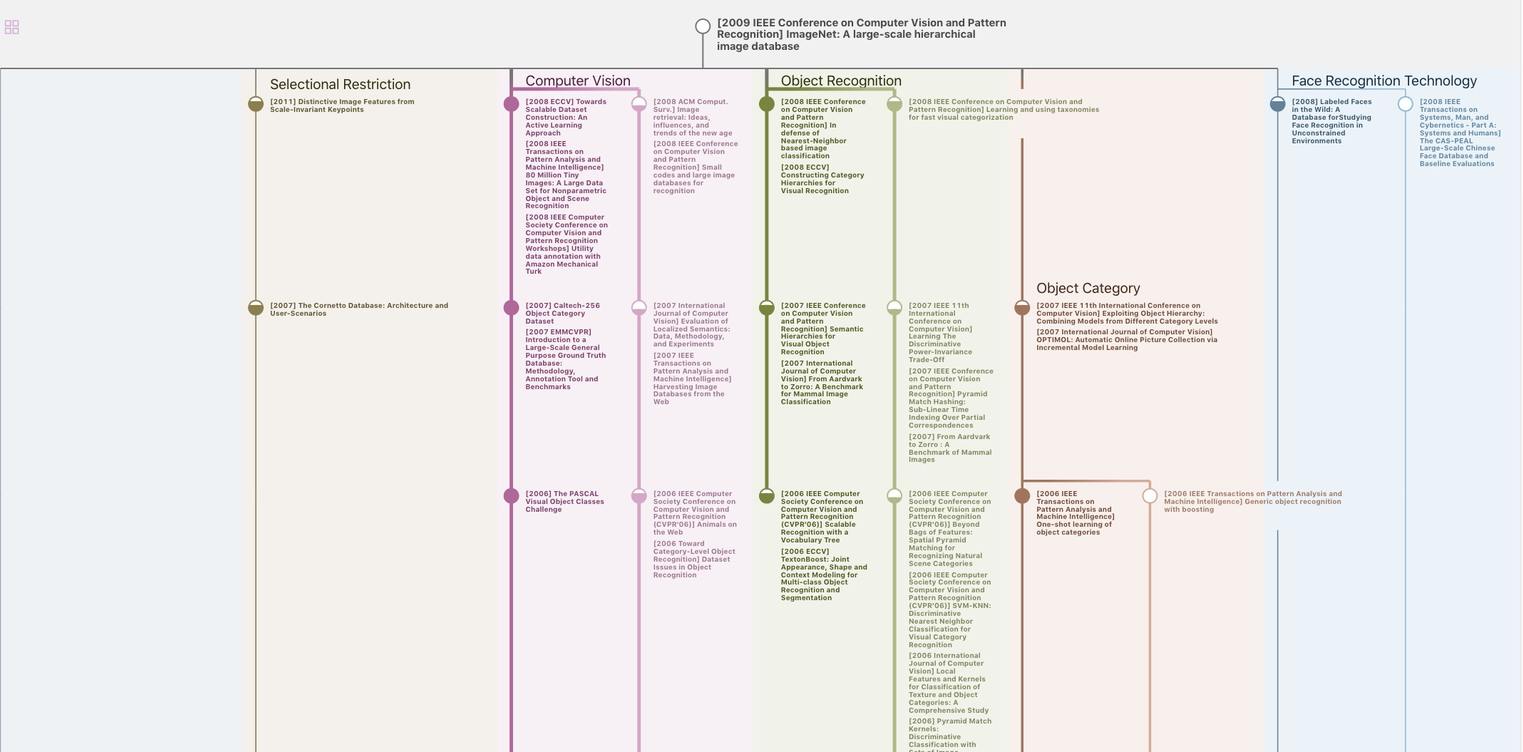
生成溯源树,研究论文发展脉络
Chat Paper
正在生成论文摘要