DFC: A Density-based Fuzzy Clustering Algorithm
msra
摘要
The majority of clustering algorithms assign each object to exactly one cluster, assuming that the boundaries of a cluster are strictly defined. However, this approach does not always corre- spond to the real-world applications since the boundaries among clusters can be fuzzy, in the sense that an object can be assigned to more than one cluster. Thus, fuzzy clustering arises as a commonly used conceptual and algorithmic framework for data analysis and unsupervised pattern recognition. In this paper we present a new non-crisp clustering algorithm, DFC, which based on density notion of clusters, discovers arbitrarily shaped clusters. Furthermore, it takes into account the uncertainty in the clustering process so that the objects are assigned to potentially more than one cluster with some degree of belief. The experimental study with various datasets shows that DFC combines effi- ciently geometrical and uncertainty aspects of the underlying data to define the clustering that best represents the structure of the data set under concern.
更多查看译文
AI 理解论文
溯源树
样例
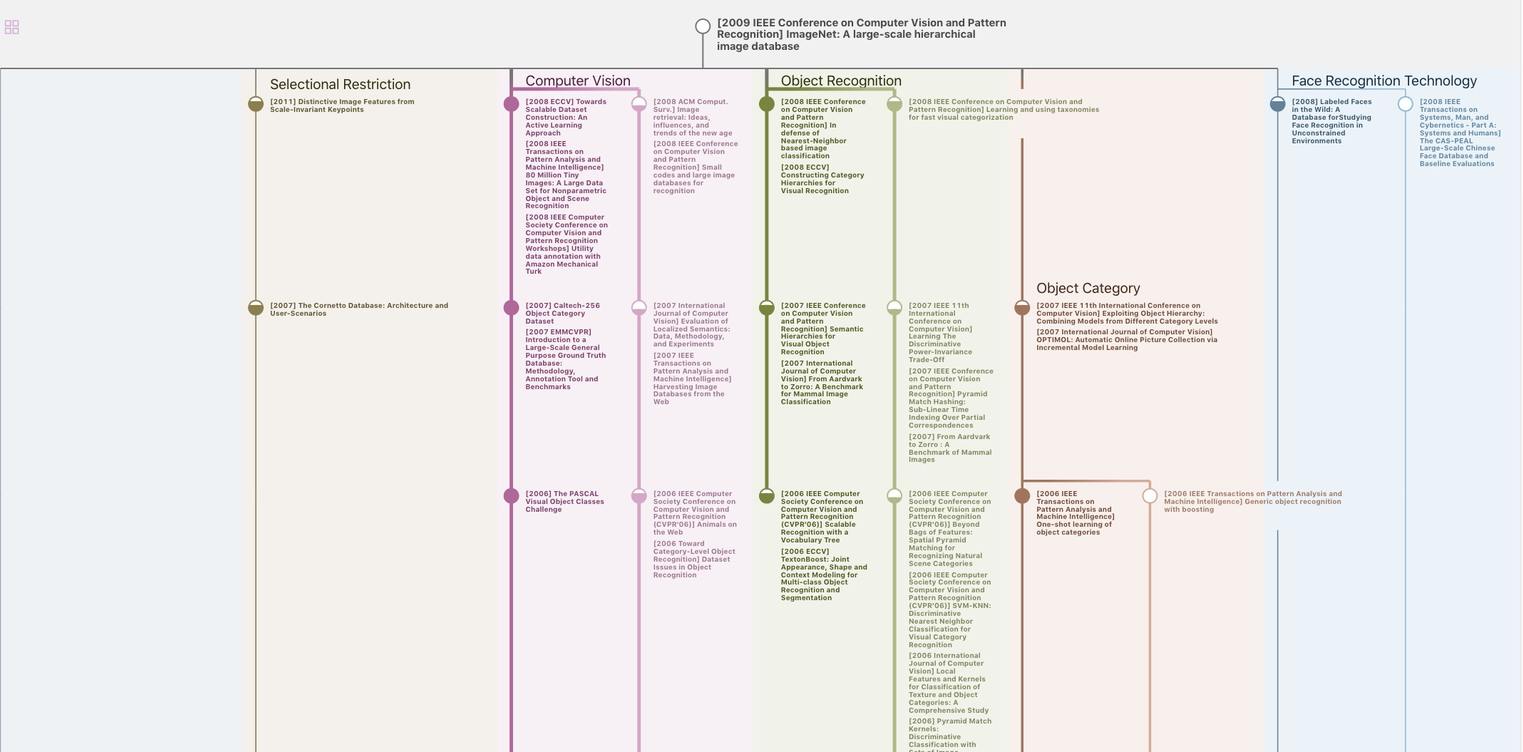
生成溯源树,研究论文发展脉络
Chat Paper
正在生成论文摘要