On the Scalability of Graph Kernels Applied to Collaborative Recommenders
msra(2008)
摘要
We study the scalability of several recent graph kernel-based collaborative recommendation algorithms. We compare the performance of several graph kernel-based recommendation algorithms, focussing on runtime and rec- ommendation accuracy with respect to the reduced rank of the subspace. We inspect the exponential and Laplacian expo- nential kernels, the resistance distance kernel, the regularized Laplacian kernel, and the stochastic diffusion kernel. Further- more, we introduce new variants of kernels based on the graph Laplacian which, in contrast to existing kernels, also allow negative edge weights and thus negative ratings. We perform an evaluation on the Netflix Prize rating corpus on prediction and recommendation tasks, showing that dimensionality re- duction not only makes prediction faster, but sometimes also more accurate. we study the performance of collaborative recommendation algorithms in combination with dimensionality reduction. Our contributions are therefore as follows: First, we study the prediction accuracy of graph kernels for recommendation on signed data, as opposed to unsigned data. Second, we in- troduce signed variants of all graph kernels that are based on the graph Laplacian. Third, we evaluate the influence of di- mensionality reduction on the recommendation accuracy and runtime performance for both training and prediction. Next, we first review related work; we then introduce terms and definitions used in collaborative filtering. The different graph kernels we consider in this paper are presented in the third section and the fourth section describes how to apply dimensionality reduction to each of these kernels. Afterwards, we discuss the application of graph kernels to recommendation algorithms. Finally we present and discuss our experimental evaluation.
更多查看译文
AI 理解论文
溯源树
样例
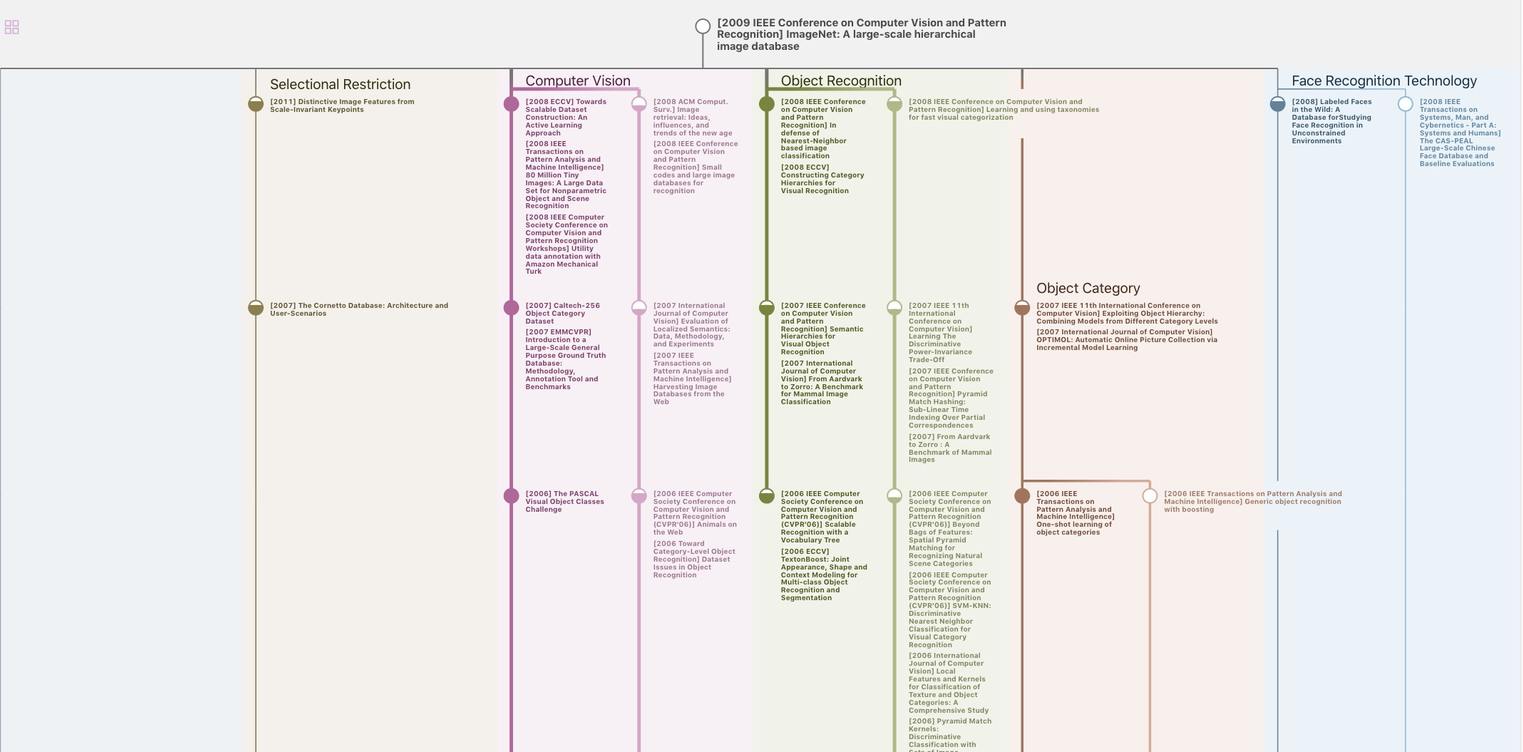
生成溯源树,研究论文发展脉络
Chat Paper
正在生成论文摘要