A Framework for Efficient Mining of Structural Information to Detect Zero-Day Malicious Portable Executables
msra(2009)
摘要
In this paper, we present an accurate and realtime PE-Miner framework that automatically extracts distinguishing features from portable executables (PE) to detect zero-day malware without any a priori knowledge about them. The distinguishing features are extracted using the structural information stan- dardized by the Microsoft Windows operating system for executables, DLLs and object files. We follow a threefold research methodology: (1) identify a set of structural features for PE files, which is com- putable in realtime, (2) use an efficient preprocessor for removing redundancy in the features' set, and (3) select an efficient data mining algorithm for final classification. The primary objective of PE-Miner is to distinguish between the benign and malicious executables; while its secondary task is to categorize the malicious executables as a function of their payload. We evaluated PE-Miner on two malware collec- tions, VX Heavens and Malfease datasets that contain 11 and 5 thousand malicious PE files respectively. The results of our experiments show that PE-Miner achieves more than 99% detection rate with less than 0.5% false alarm rate for distinguishing between the benign and malicious executables. Further- more, it achieves an average detection rate of 90% with an average false alarm rate of less than 5% for categorizing the malicious executables as a function of their payload. It is important to emphasize that PE-Miner has low processing overheads and takes only0.244 seconds on the average to scan a given PE file. Finally, we evaluate the robustness and reliability of PE-Miner under several regression tests. Our results show that extracted features are "robust" to different packing techniques and PE-Miner is also resilient to majority of "crafty" evasion strategies. The research presented in this paper is conducted at nexGIN RC and is funded by National ICT R&D Fund, Ministry of Information Technology, Government of Pakistan under the grant # ICTRDF/AN/2007/37. The information, data, comments, and views detailed herein may not necessarily reflect the endorsements of views of the National ICT R&D Fund.
更多查看译文
关键词
a priori knowledge,regression testing,operating system,information technology,research methodology,false alarm rate
AI 理解论文
溯源树
样例
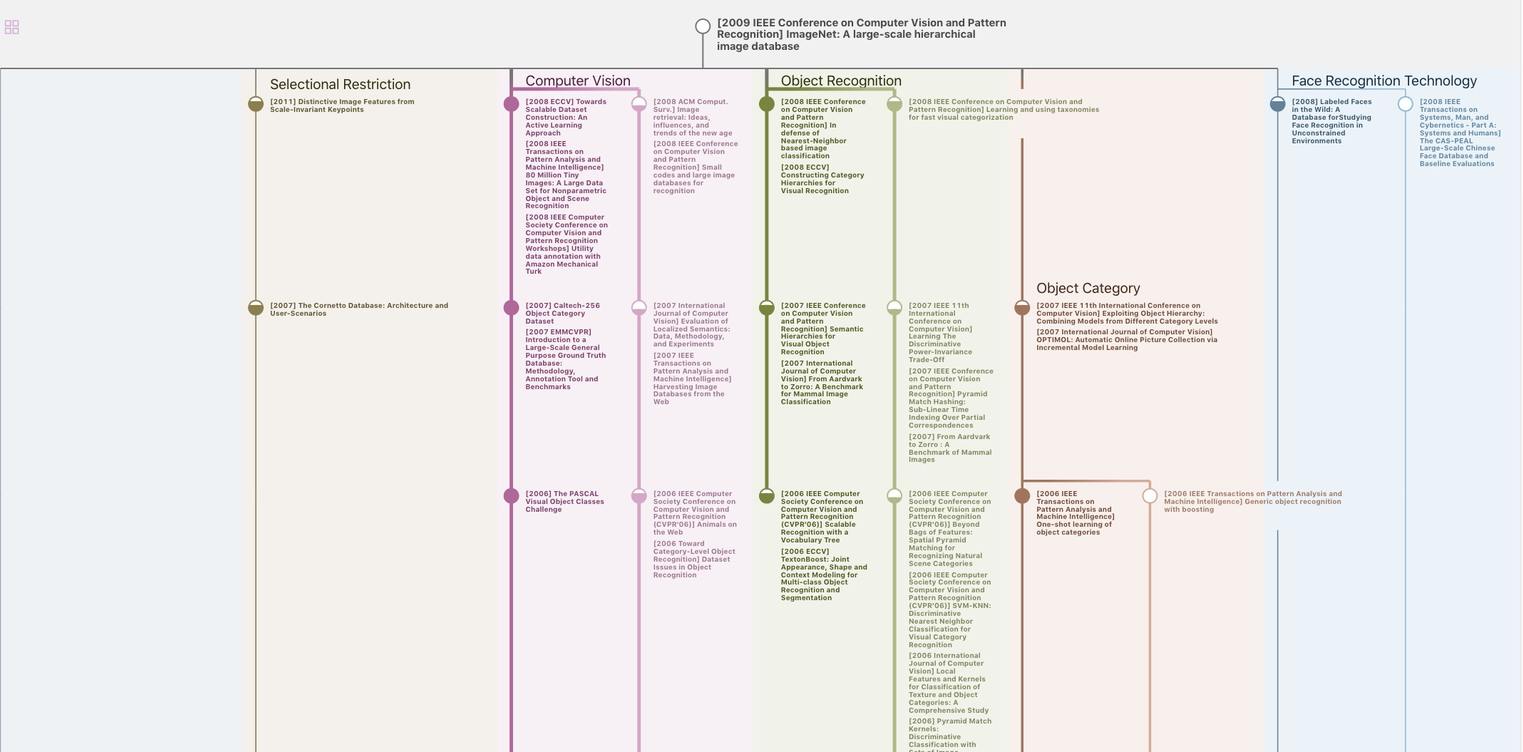
生成溯源树,研究论文发展脉络
Chat Paper
正在生成论文摘要