Active microscopic cellular image annotation by superposable graph transduction with imbalanced labels
CVPR(2008)
摘要
Systematic content screening of cell phenotypes in microscopic images has been shown promising in gene function understanding and drug design. However, manual annotation of cells and images in genome-wide studies is cost prohibitive. In this paper, we propose a highly efficient active annotation framework, in which a small amount of expert input is leveraged to rapidly and effectively infer the labels over the remaining unlabeled data. We formulate this as a graph based transductive learning problem and develop a novel method for label propagation. Specifically, a label regularizer method is proposed to handle the important label imbalance issue, typically seen in the cellular image screening applications. We also design a new scheme which breaks the graph into linear superposition of contributions from individual labeled samples. We take advantage of such a superposable representation to achieve fast annotation in an interactive setting. Extensive evaluations over toy data and realistic cellular images confirm the superiority of the proposed method over existing alternatives.
更多查看译文
关键词
systematic content screening,label propagation,cellular biophysics,gene function,drug design,learning (artificial intelligence),genetics,microscopy,active microscopic cellular image annotation,superposable graph transductive learning problem,graph theory,imbalanced label,medical image processing,label regularizer method,biology,interference,image retrieval,fluorescence,bioinformatics,image annotation,transductive learning,dna,learning artificial intelligence,biological processes,genomics,harmonic analysis
AI 理解论文
溯源树
样例
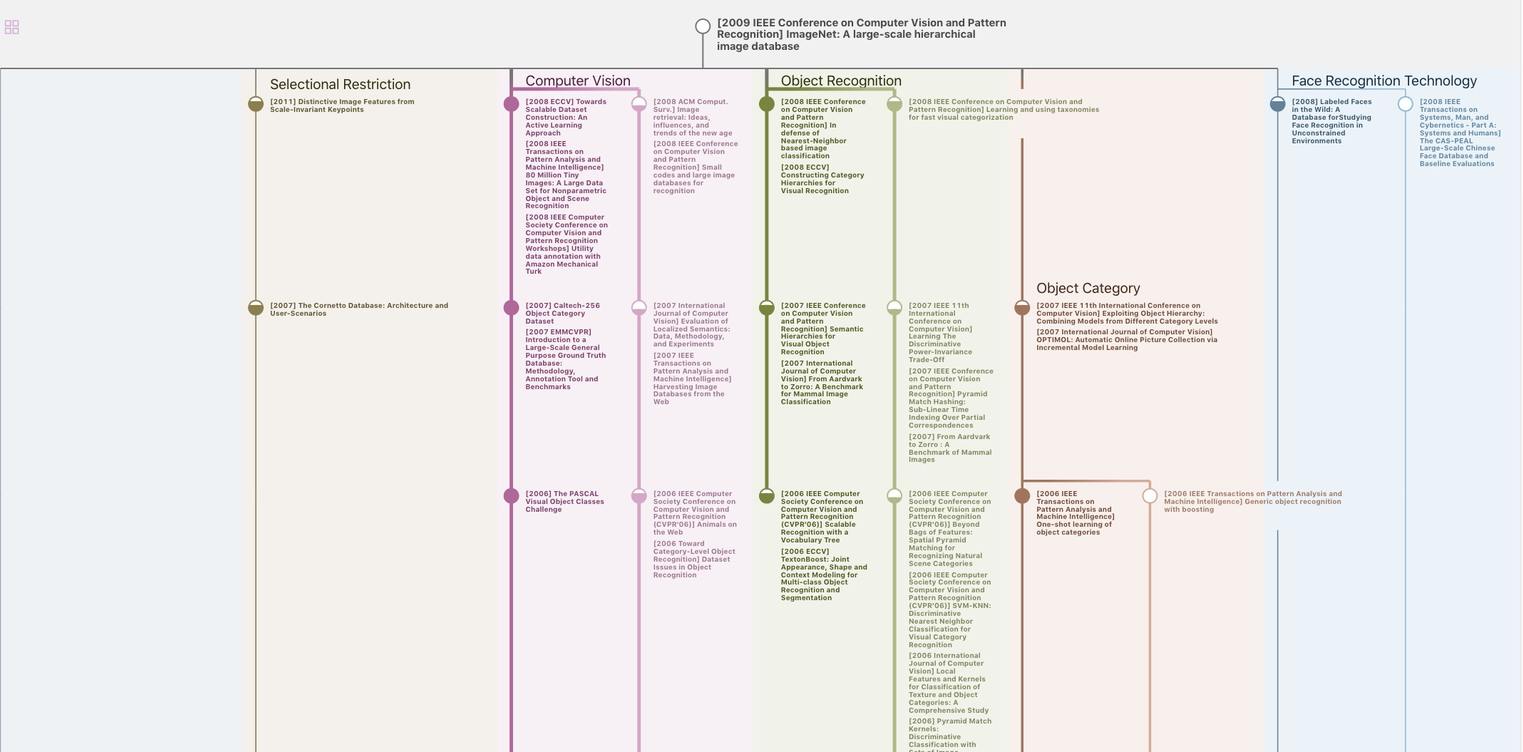
生成溯源树,研究论文发展脉络
Chat Paper
正在生成论文摘要