Uncertainties in Air Toxics Calculated by the Dispersion Models AERMOD and ISCST3 in the Houston Ship Channel Area
JOURNAL OF APPLIED METEOROLOGY AND CLIMATOLOGY(2009)
摘要
The uncertainties in simulations of annually averaged concentrations of two air toxics (benzene and 1,3-butadiene) are estimated for two widely used U. S. air quality models, the Industrial Source Complex Short-Term, version 3, (ISCST3) model and the American Meteorological Society-Environmental Protection Agency Model (AERMOD). The effects of uncertainties in emissions input, meteorological input, and dispersion model parameters are investigated using Monte Carlo probabilistic uncertainty methods, which involve simultaneous random and independent perturbations of all inputs. The focus is on a 15 km x 15 km domain in the Houston, Texas, ship channel area. Concentrations are calculated at hypothetical receptors located at the centroids of population census tracts. The model outputs that are analyzed are the maximum annually averaged maximum concentration at any single census tract or monitor as well as the annually averaged concentration averaged over the census tracts. The input emissions uncertainties are estimated to be about a factor of 3 (i.e., covering the 95% range) for each of several major categories. The uncertainties in meteorological inputs (such as wind speed) and dispersion model parameters (such as the vertical dispersion coefficient sigma(z)) also are estimated. The results show that the 95% range in predicted annually averaged concentrations is about a factor of 2-3 for the air toxics, with little variation by model. The input variables whose variations have the strongest effect on the predicted concentrations are on-road mobile sources and some industrial sources (dependent on chemical), as well as wind speed, surface roughness, and sigma(z). In most scenarios, the uncertainties of the emissions input group contribute more to the total uncertainty than do the uncertainties of the meteorological/dispersion input group.
更多查看译文
AI 理解论文
溯源树
样例
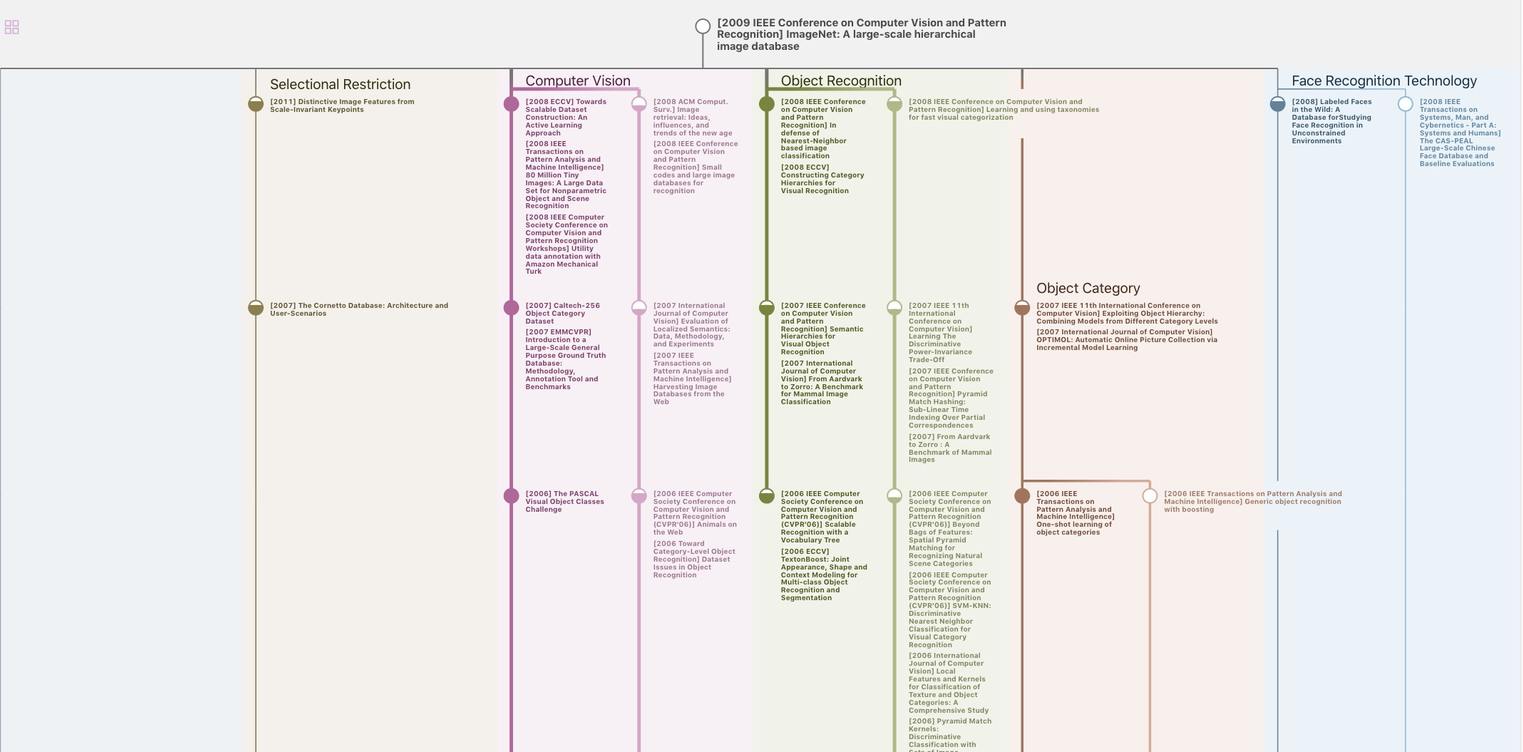
生成溯源树,研究论文发展脉络
Chat Paper
正在生成论文摘要