Hyperlink Prediction In Hypernetworks Using Latent Social Features
DISCOVERY SCIENCE(2013)
摘要
Predicting the existence of links between pairwise objects in networks is a key problem in the study of social networks. However, relationships among objects are often more complex than simple pairwise relations. By restricting attention to dyads, it is possible that information valuable for many learning tasks can be lost. The hypernetwork relaxes the assumption that only two nodes can participate in a link, permitting instead an arbitrary number of nodes to participate in so-called hyperlinks or hyperedges, which is a more natural representation for complex, multi-party relations. However, the hyperlink prediction problem has yet to be studied. In this paper, we propose HPLSF (Hyperlink Prediction using Latent Social Features), a hyperlink prediction algorithm for hypernetworks. By exploiting the homophily property of social networks, HPLSF explores social features for hyperlink prediction. To handle the problem that social features are not always observable, a latent social feature learning scheme is developed. To cope with the arbitrary cardinality hyperlink issue in hypernetworks, we design a feature-embedding scheme to map the a priori arbitrarily-sized feature set associated with each hyperlink into a uniformly-sized auxiliary space. To address the fact that observed features and latent features may be not independent, we generalize a structural SVM to learn using both observed features and latent features. In experiments, we evaluate the proposed HPLSF framework on three large-scale hypernetwork datasets. Our results on the three diverse datasets demonstrate the effectiveness of the HPLSF algorithm. Although developed in the context of social networks, HPLSF is a general methodology and applies to arbitrary hypernetworks.
更多查看译文
关键词
Hypernetworks, Hyperlink Prediction, Social Features
AI 理解论文
溯源树
样例
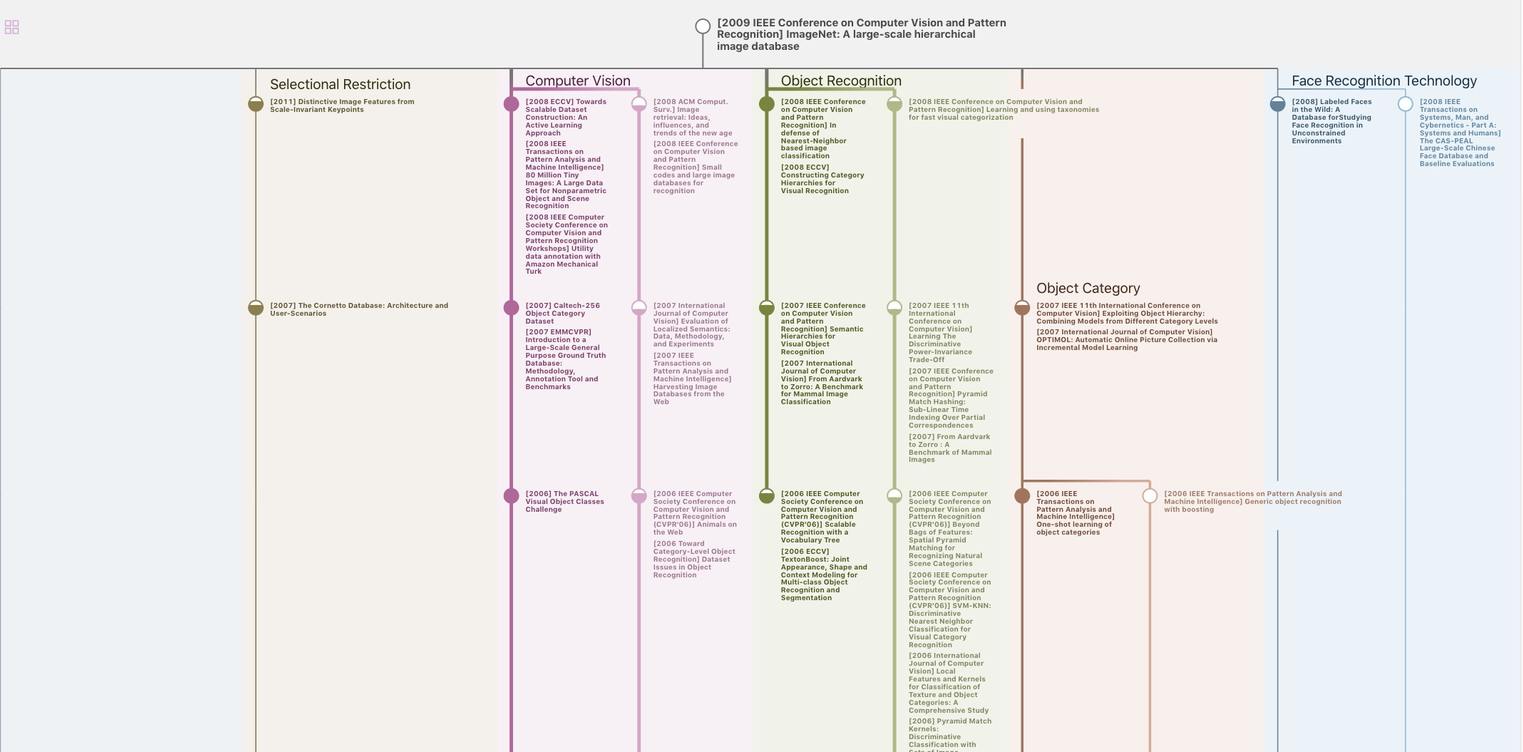
生成溯源树,研究论文发展脉络
Chat Paper
正在生成论文摘要