Implicit trajectory modelling using temporally varying weight regression for automatic speech recognition
ICASSP(2012)
摘要
Recently, implicit trajectory modelling using temporally varying model parameters has achieved promising gains over the discriminatively trained standard HMM system. However, these works only focus on the temporally varying means or precisions explicitly. It is interesting to explore the capability of temporally varying weights, since the effect of time varying Gaussian parameters can be achieved by adjusting the weights of Gaussian Mixture Models (GMM) for different observation. This paper proposes a Temporally Varying Weight Regression (TVWR) model to learn the importance of different Gaussian components under different temporal contexts. Technically, TVWR factorizes the HMM state likelihood such that the contextual information can be modelled using time varying weights. Additionally, approximate constraints are derived to ensure a valid probabilistic model for TVWR. Experimental results for continuous speech recognition on Wall Street Journal show consistent improvements with varying system complexity and about 12% relative significant improvements in the best case.
更多查看译文
关键词
trajectory modelling,gmm,speech recognition,time varying gaussian parameter effect,regression analysis,temporally varying weight regression model,hmm state likelihood,tvwr model,gaussian processes,gaussian mixture models,regression,hidden markov model system,implicit trajectory modelling,nonlinear constrained optimization,continuous speech recognition,hidden markov models,trained standard hmm system,probability,complexity control,automatic speech recognition,probabilistic model,context modeling,trajectory
AI 理解论文
溯源树
样例
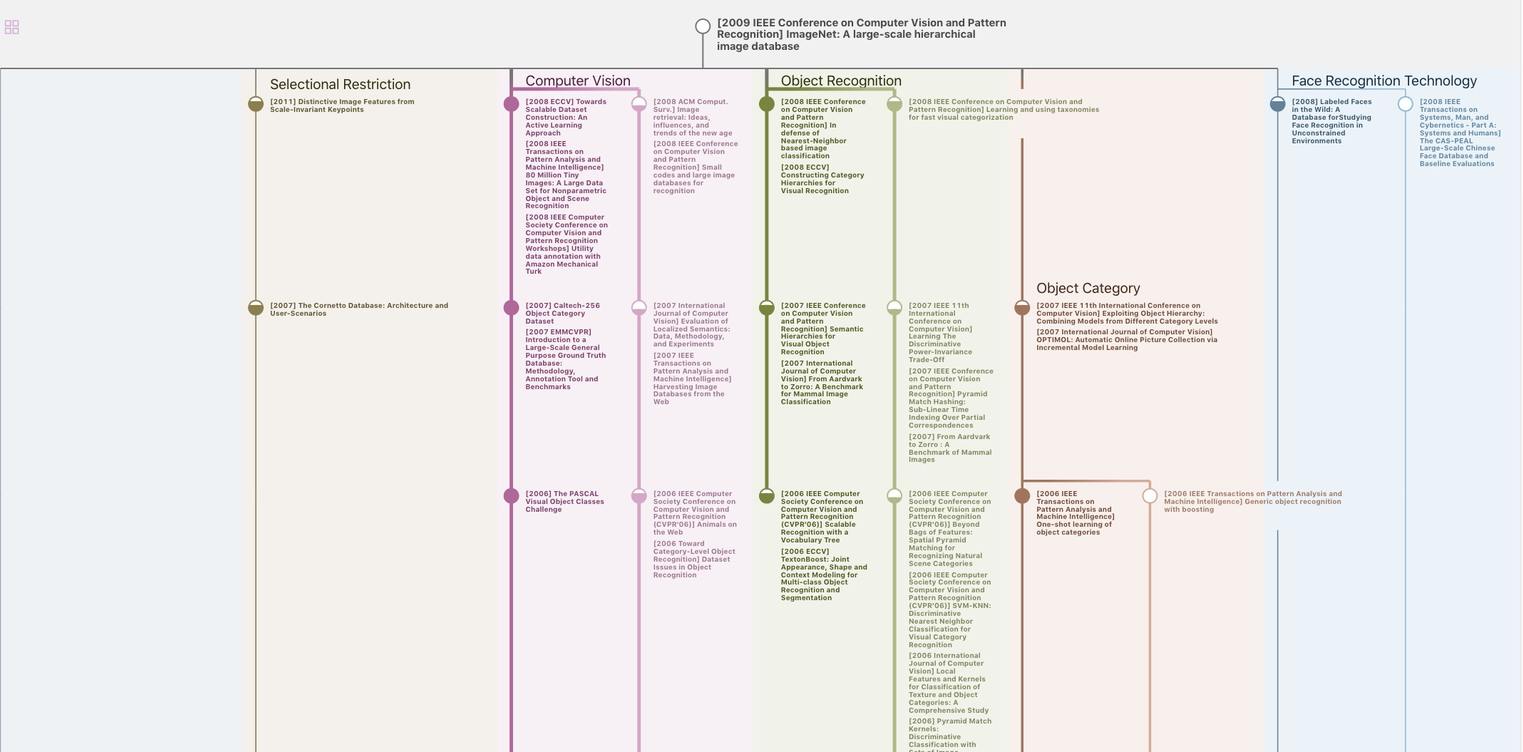
生成溯源树,研究论文发展脉络
Chat Paper
正在生成论文摘要