Knowledge-based gene selection for molecular classification
msra
摘要
Motivation: In this study we designed classifier systems to predict clinical outcomes of several cancer types using the expression levels of microarray data. Before classifi- cation a gene selection is performed by selecting the most informative genes. Instead of regular gene selection tech- niques, which select genes and train a classifier in a purely data-driven fashion, we propose to select genes based on their biological knowledge. Purely data-driven techniques do not exploit the wealth of biological knowledge available, and moreover, when a classifier is constructed based on biologi- cal knowledge, the resulting classifier should be more inter- pretable. The biological knowledge used is the Gene On- tology 1 (The Gene Ontology Consortium, 2000), which is a controlled vocabulary describing gene products and related functions. The Gene Ontology provides us with a relational structure by grouping the genes into gene sets according to their biological descriptions (annotations). These gene sets are connected to more global descriptions by means of a di- rected acyclic graph, specifying relational structures between gene sets. So, instead of considering single genes as fea- tures, we will consider groups of gene sets, as defined in the ontology as features. In addition to gene selection methods, we study the use of classifier combiner techniques using the relational structure between gene sets. We fuse classifiers trained on gene sets to predict one clinical outcome based on the descriptions of these gene sets. Results: We conclude that it is possible to do a dimension- ality reduction that is purely based on biological knowledge. The classifier systems based on the Gene Ontology data- base give the clinical prediction more meaning when consid- ering the description of selected Gene Ontology nodes. In 1With the Gene Ontology we mean the gene annotations and Gene Ontol-
更多查看译文
关键词
ogy database
AI 理解论文
溯源树
样例
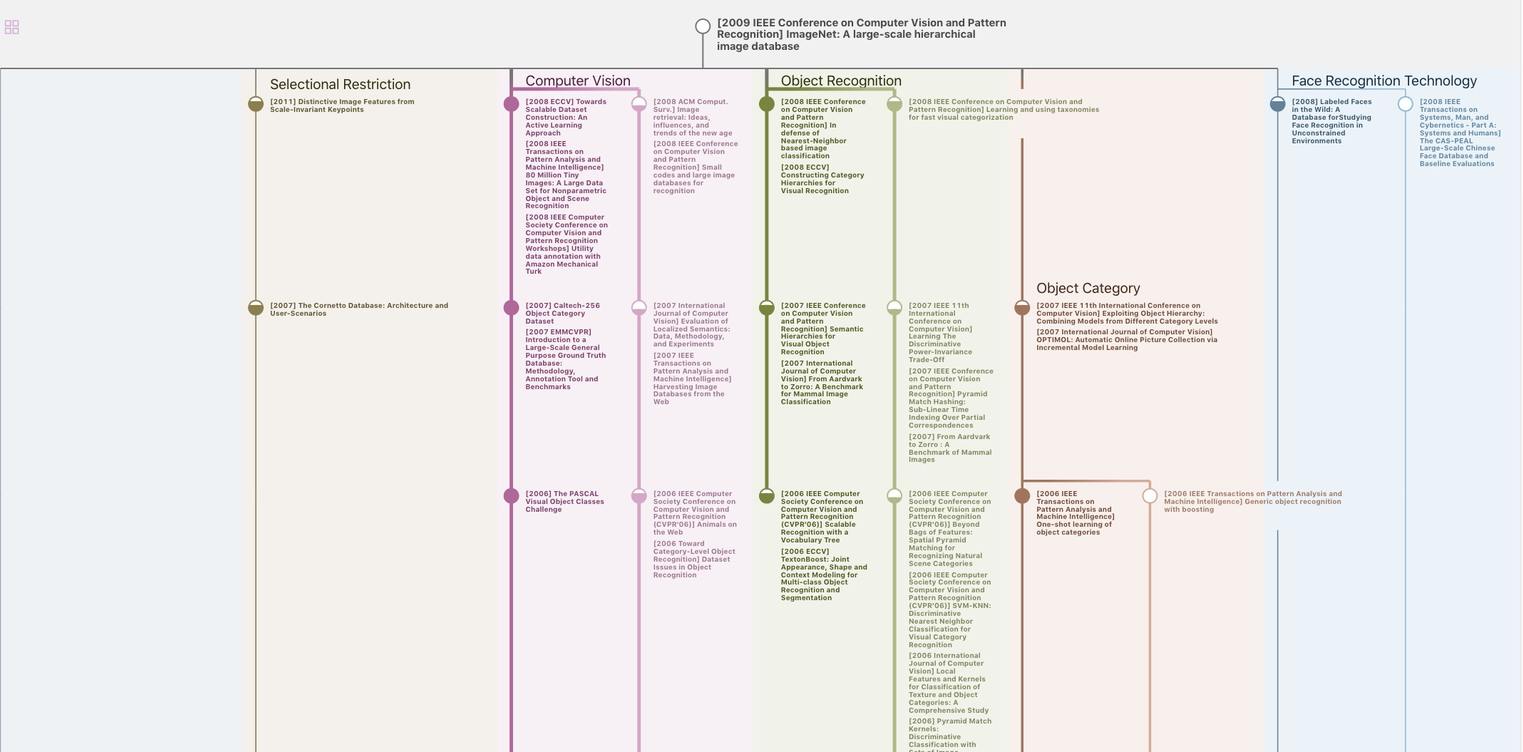
生成溯源树,研究论文发展脉络
Chat Paper
正在生成论文摘要