Characterization of prokaryotic and eukaryotic promoters using hidden Markov models.
PubMed(1996)
摘要
In this paper we utilize hidden Markov models (HMMs) and information theory to analyze prokaryotic and eukaryotic promoters. We perform this analysis with special emphasis on the fact that promoters are divided into a number of different classes, depending on which polymerase-associated factors that bind to them. We find that HMMs trained on such subclasses of Escherichia coli promoters (specifically, the so-called sigma 70 and sigma 54 classes) give an excellent classification of unknown promoters with respect to sigma-class. HMMs trained on eukaryotic sequences from human genes also model nicely all the essential well known signals, in addition to a potentially new signal upstream of the TATA-box. We furthermore employ a novel technique for automatically discovering different classes in the input data (the promoters) using a system of self-organizing parallel HMMs. These self-organizing HMMs have at the same time the ability to find clusters and the ability to model the sequential structure in the input data. This is highly relevant in situations where the variance in the data is high, as is the case for the subclass structure in for example promoter sequences.
更多查看译文
关键词
eukaryotic promoters,hidden markov models
AI 理解论文
溯源树
样例
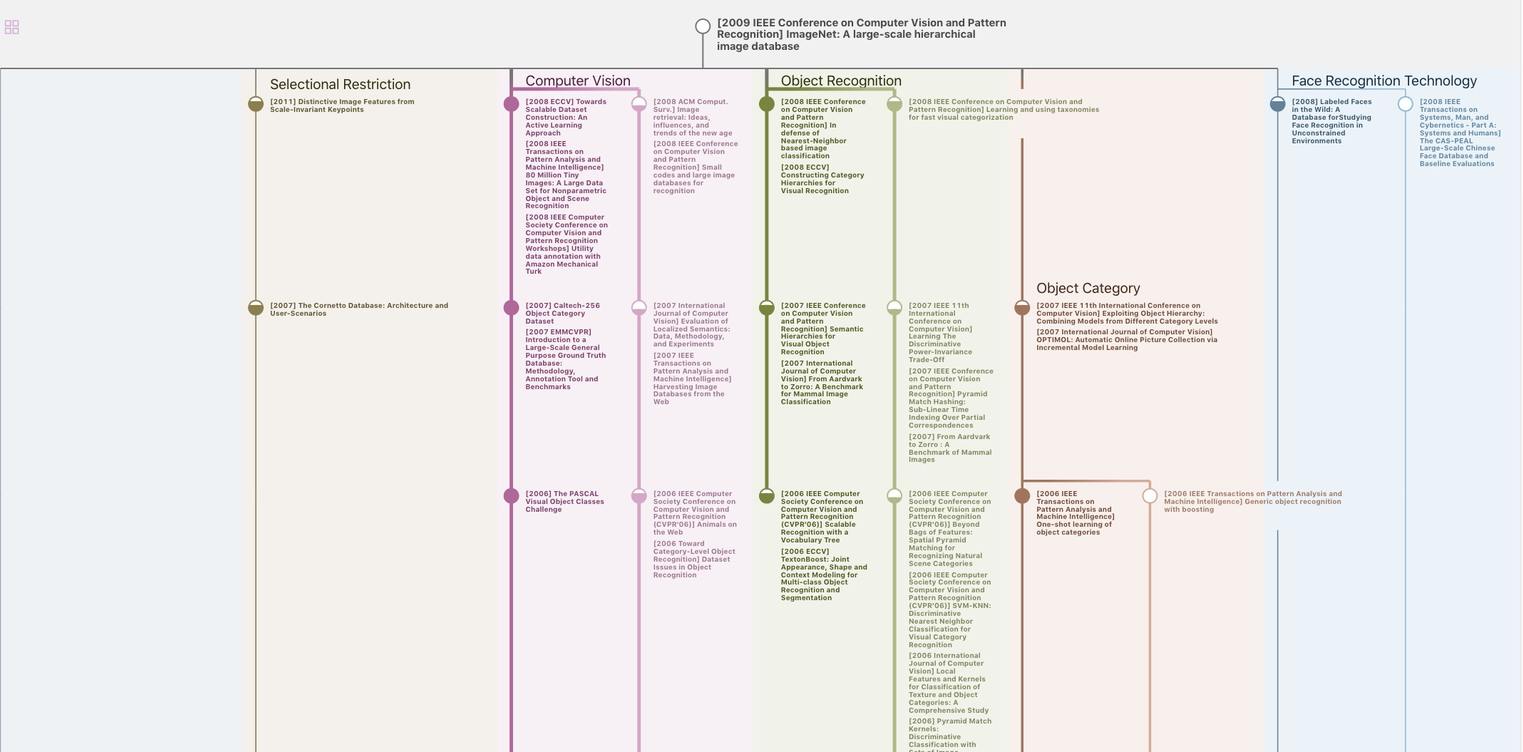
生成溯源树,研究论文发展脉络
Chat Paper
正在生成论文摘要