Quality-Based Visualization Matrices
VMV(2009)
摘要
Parallel coordinates and scatterplot matrices are widely used to visualize multi-dimensional data sets. But these visualization techniques are in- sufficient when the number of dimensions grows. To solve this problem, different approaches to pre- select the best views or dimensions have been pro- posed in the last years. However, there are still sev- eral shortcomings to these methods. In this paper we present three new methods to explore multivari- ate data sets: a parallel coordinates matrix, in anal- ogy to the well-known scatterplot matrix, a class- based scatterplot matrix that aims at finding good projections for each class pair, and an importance aware algorithm to sort the dimensions of scatter- plot and parallel coordinates matrices. where the values of two variables for a sample in a data set are used to plot a point in 2-dimensional space, resulting in a scattering of points. Scatter- plots are very useful for visually determining the correlation between two variables. A SPLOM is a symmetric matrix of adjacent scatterplots and al- lows the user to analyze the diverse dimensions at once. If there are n variables, the SPLOM has di- mension n × n and the element at the i-th row and j-th column is a scatterplot of the i-th and j-th vari- able. Related to this kind of visualization, we pro- pose two extensions: a class based scatterplot ma- trix and an importance oriented reordering of the dimensions of the matrix. The proposal of the class based SPLOM(C-SPLOM) is to support the visual analysis of labeled (classified) data sets. In such data sets, the analyst often searches for projections where distinct clusters can be observed. Previous approaches aim at finding good views of a data set considering all classes at once. The problem with such approaches is that this global optimization may ignore views that separate two classes well, be- cause of the distribution of the remaining classes. To deal with this, our C-SPLOM presents the best projection for each class pair, based on a ranking in- dex. This class based visualization method is useful to analyze labeled data sets with a large number of variables that cannot be well visualized using tradi- tional SPLOMs. Another popular visualization technique are par- allel coordinates plots (PCPs) (9). In such plots, each sample of an N -dimensional data set is rep- resented by a polyline that intersects N vertical axes (dimensions). The intersection point repre- sents its value in the respective dimension. Similar to the scatterplot matrices the parallel coordinates plots, do not scale well when the number of dimen- sions grows, as important dimensional relationships might not be visualized. Addressing this shortcom- ing, we propose an importance oriented parallel co-
更多查看译文
关键词
parallel coordinates,visual analysis,global optimization,2 dimensional,symmetric matrix
AI 理解论文
溯源树
样例
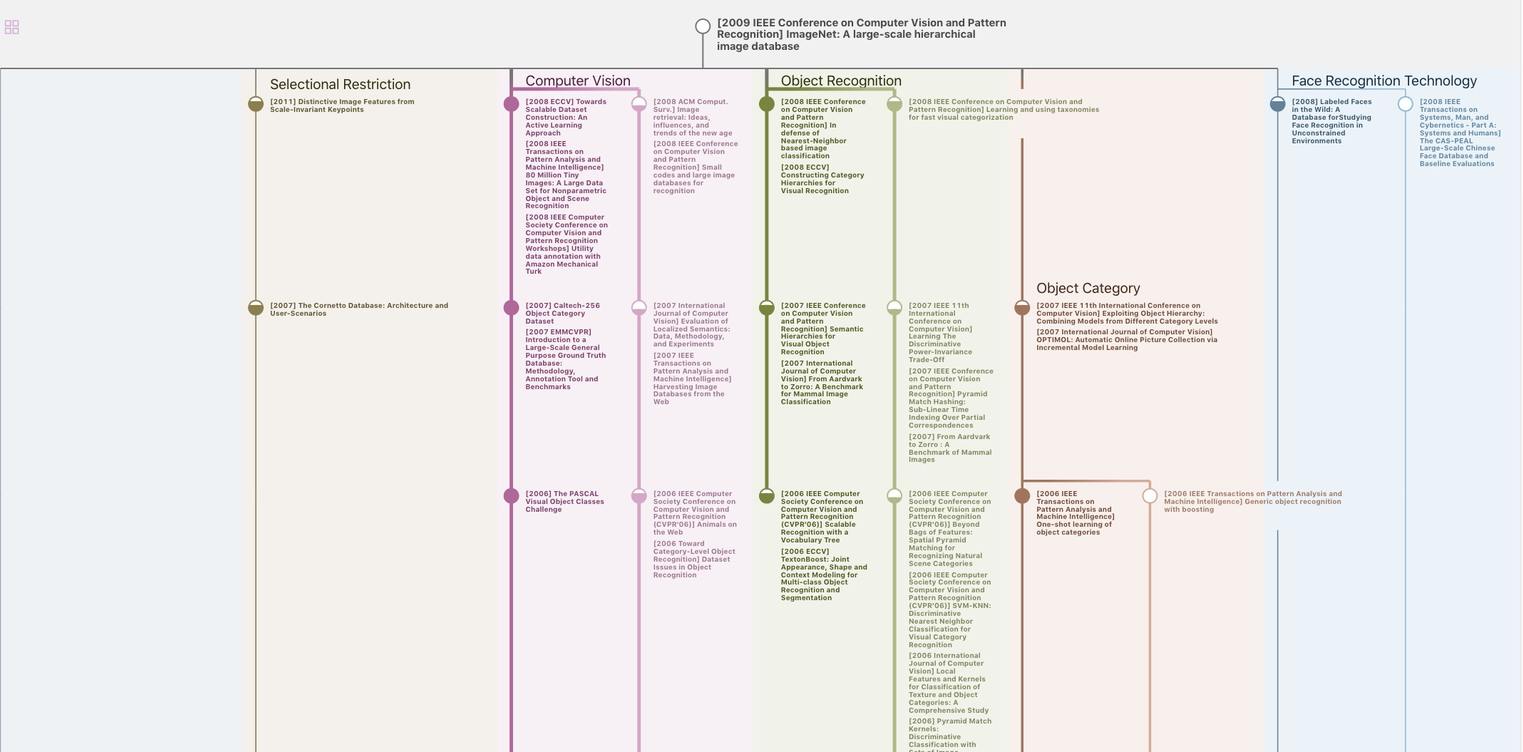
生成溯源树,研究论文发展脉络
Chat Paper
正在生成论文摘要