Robust information-theoretic clustering
KDD(2006)
摘要
How do we find a natural clustering of a real world point set, which contains an unknown number of clusters with dierent shapes, and which may be contaminated by noise? Most clustering algorithms were designed with certain as- sumptions (Gaussianity), they often require the user to give input parameters, and they are sensitive to noise. In this pa- per, we propose a robust framework for determining a nat- ural clustering of a given data set, based on the minimum description length (MDL) principle. The proposed frame- work, Robust Information-theoretic Clustering (RIC), is or- thogonal to any known clustering algorithm: given a pre- liminary clustering, RIC purifies these clusters from noise, and adjusts the clusterings such that it simultaneously de- termines the most natural amount and shape (subspace) of the clusters. Our RIC method can be combined with any clustering technique ranging from K-means and K-medoids to advanced methods such as spectral clustering. In fact, RIC is even able to purify and improve an initial coarse clustering, even if we start with very simple methods such as grid-based space partitioning. Moreover, RIC scales well with the data set size. Extensive experiments on synthetic and real world data sets validate the proposed RIC frame- work.
更多查看译文
关键词
natural amount,data summarization,ric method,clustering technique,natural clustering,robust information-theoretic clustering,spectral clustering,preliminary clustering,ric scale,proposed ric framework,noise- robustness,parameter-free data mining,clustering algorithm,initial coarse clustering,clustering,data mining,k means,minimum description length
AI 理解论文
溯源树
样例
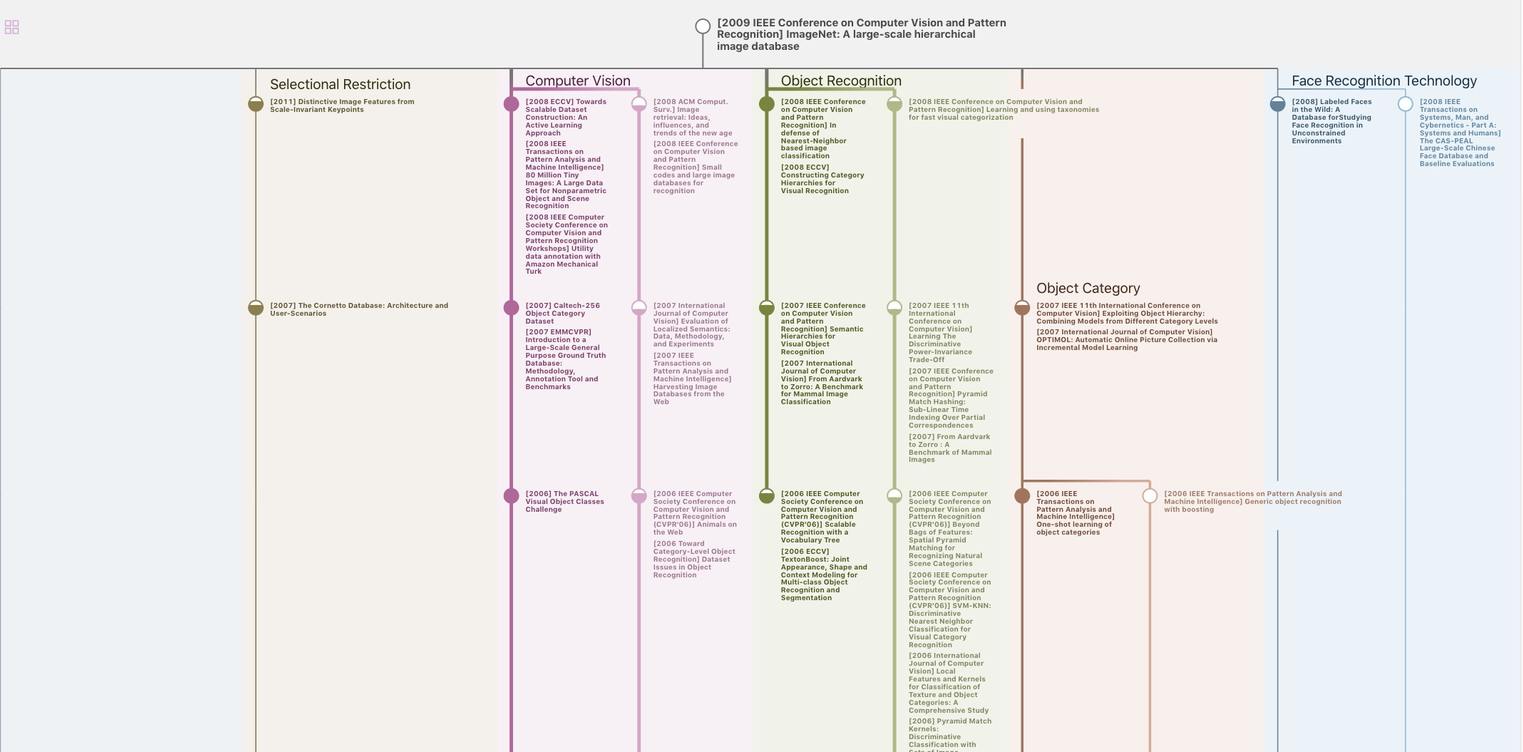
生成溯源树,研究论文发展脉络
Chat Paper
正在生成论文摘要