SoftRank with Gaussian Processes
msra(2007)
摘要
We address the problem of learning to rank based on a large feature set and a training set of judged documents for given queries. Recently there has been interest in using IR evaluation metrics to assist in training ranking functions. However, direct optimization of an IR metric such as NDCG with respect to model parameters is difficult because such a metric is non-smooth with respect to document scores. Recently Taylor et al. presented a method called SoftRank which smooths a metric such as NDCG by introducing uncertainty into the scores, thus making it amenable for optimization. In this paper we extend SoftRank by combining it with a Gaussian process (GP) model for the ranking function. The advantage is that the SoftRank smoothing uncertainties are naturally supplied by the GP, reflecting the underlying modelling uncertainty in individual document scores. We can also use these document uncertainties to rank differently, depending on how risky or conservative we want to make the ranking. We test our method on the publicly available LETOR OHSUMED data set and show very competitive results.
更多查看译文
AI 理解论文
溯源树
样例
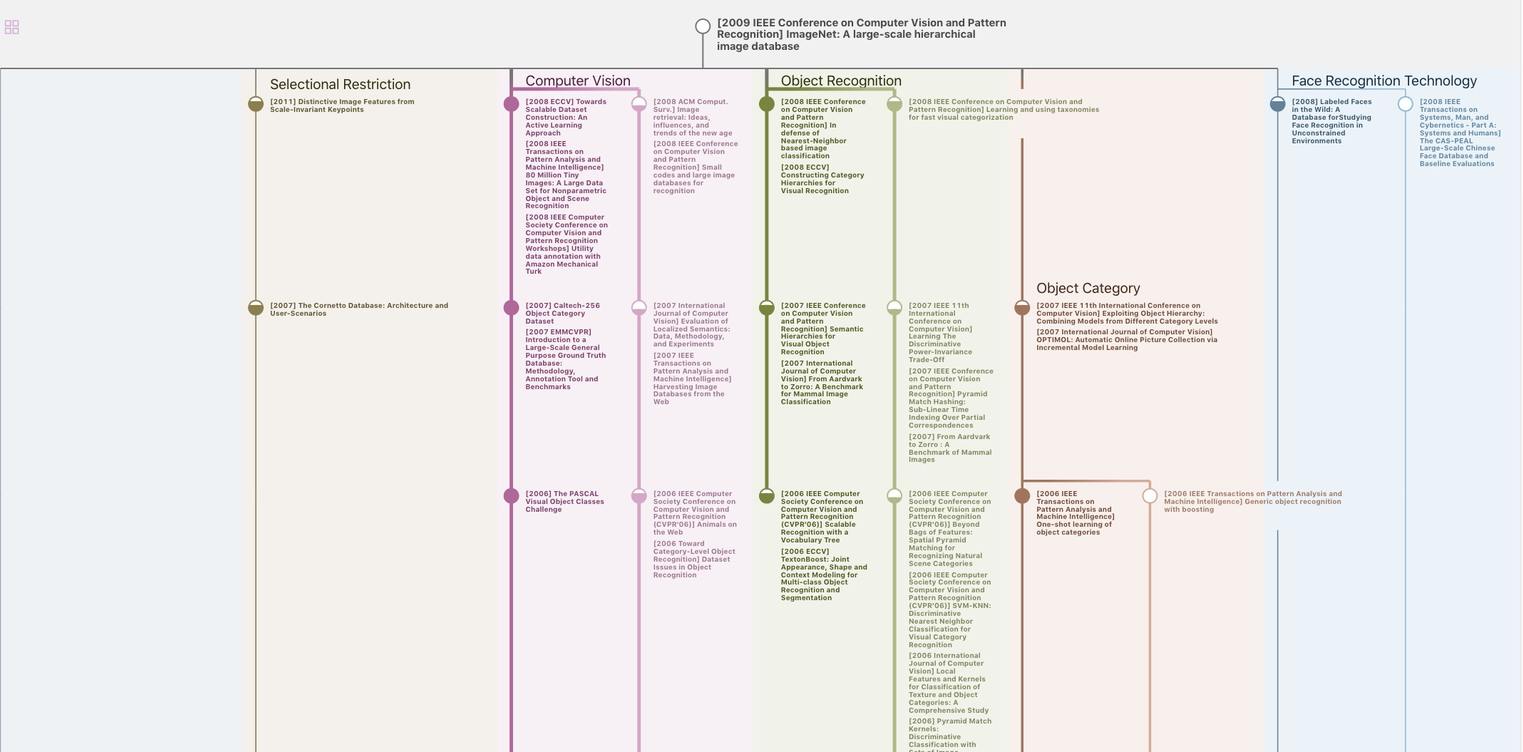
生成溯源树,研究论文发展脉络
Chat Paper
正在生成论文摘要