Text-Image Topic Discovery for Web News Data.
ECIR 2014: Proceedings of the 36th European Conference on IR Research on Advances in Information Retrieval - Volume 8416(2014)
摘要
We formally propose a new application problem: unsupervised text-image topic discovery. The application problem is important because almost all news articles have one picture associated. Unlike traditional topic modeling which considers text alone, the new task aims to discover heterogeneous topics from web news of multiple data types. The heterogeneous topic discovery is challenging because different media data types have different characteristics and structures, and a systematic solution that can integrate information propagation and mutual enhancement between data of different types in a principle way is not easy to obtain, especially when no supervision information is available. We propose to tackle the problem by a regularized nonnegative constrained l 2,1 -norm minimization framework. We also present a new iterative algorithm to solve the optimization problem. To objectively evaluate the proposed method, we collect two real world text-image web news datasets. Experimental results show the effectiveness of the new approach.
更多查看译文
关键词
Text-image topic discovery
AI 理解论文
溯源树
样例
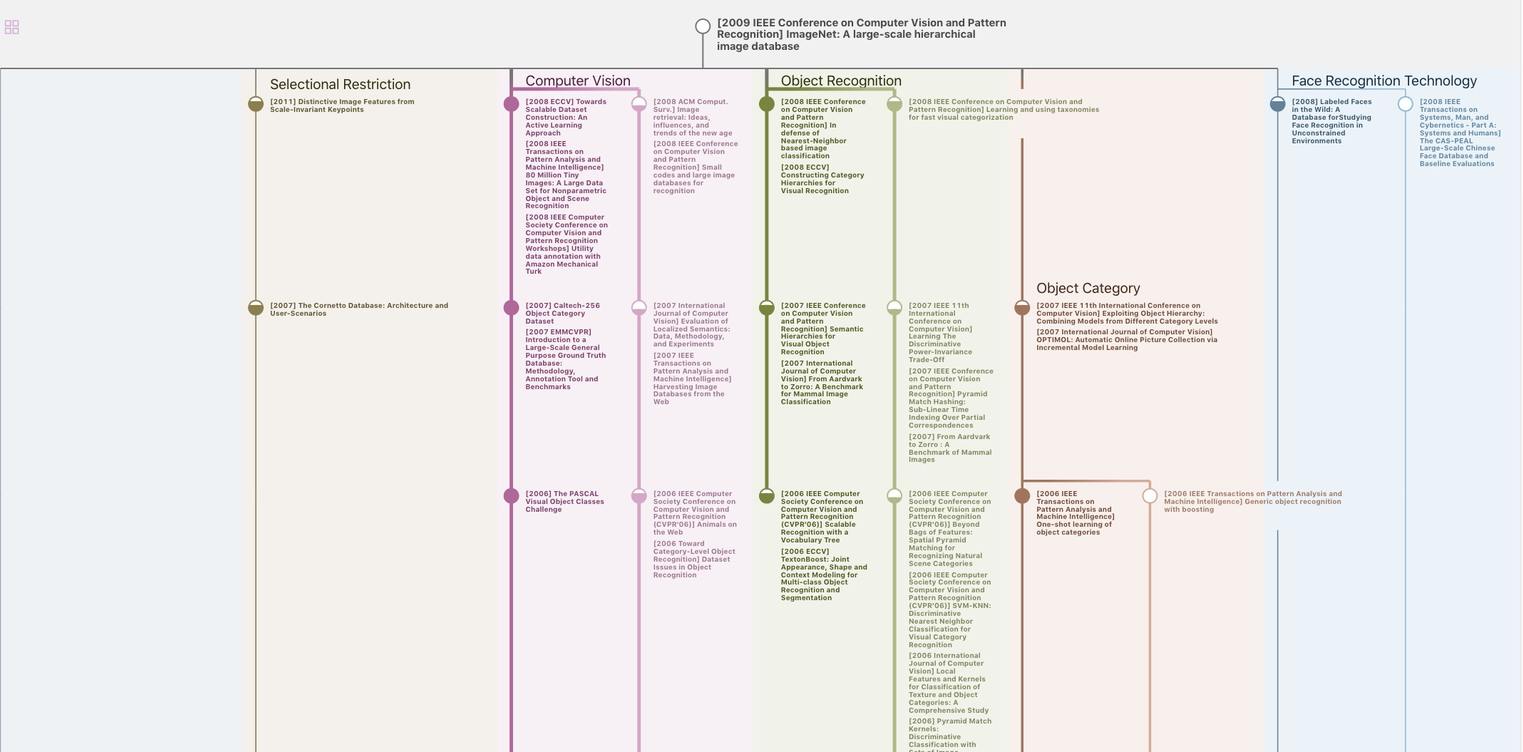
生成溯源树,研究论文发展脉络
Chat Paper
正在生成论文摘要