Geometrically proper models in statistical training.
IPMI'07: Proceedings of the 20th international conference on Information processing in medical imaging(2007)
摘要
In deformable model segmentation, the geometric training process plays a crucial role in providing shape statistical priors and appearance statistics that are used as likelihoods. Also, the geometric training process plays a crucial role in providing shape probability distributions in methods finding significant differences between classes. The quality of the training seriously affects the final results of segmentation or of significant difference finding between classes. However, the lack of shape priors in the training stage itself makes it difficult to enforce shape legality, i.e., making the model free of local self-intersection or creases. Shape legality not only yields proper shape statistics but also increases the consistency of parameterization of the object volume and thus proper appearance statistics. In this paper we propose a method incorporating explicit legality constraints in training process. The method is mathematically sound and has proved in practice to lead to shape probability distributions over only proper objects and most importantly to better segmentation results.
更多查看译文
关键词
shape legality,geometric training process,crucial role,shape prior,shape probability distribution,shape statistical prior,yields proper shape statistic,training process,training stage,better segmentation result,Geometrically proper model,statistical training
AI 理解论文
溯源树
样例
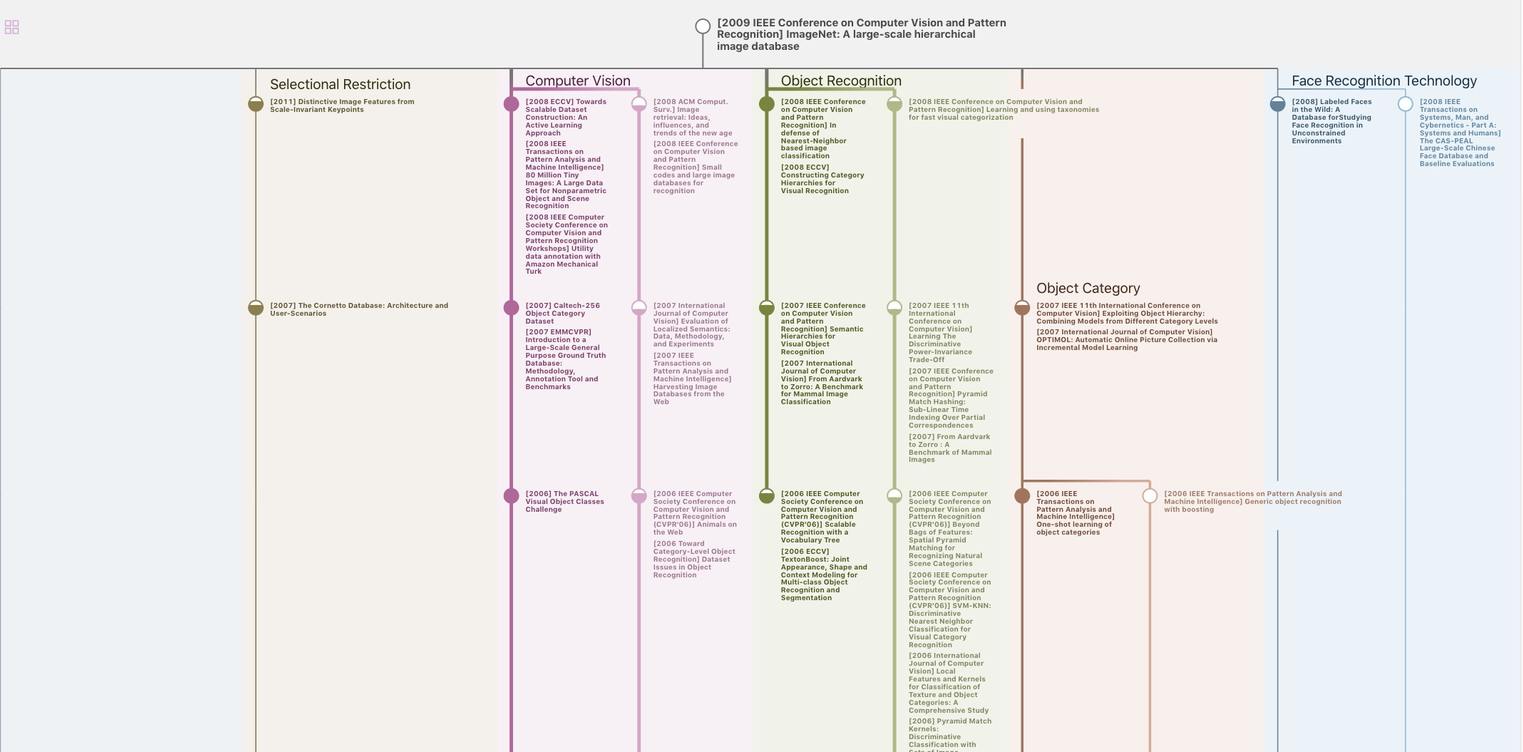
生成溯源树,研究论文发展脉络
Chat Paper
正在生成论文摘要